Progress curve analysis of qRT-PCR reactions using the logistic growth equation.
BIOTECHNOLOGY PROGRESS(2011)
摘要
We present an alternate approach for analyzing data from real-time reverse transcription polymerase chain reaction (qRT-PCR) experiments by fitting individual fluorescence vs. cycle number (F vs. C) curves to the logistic growth equation. The best fit parameters determined by nonlinear least squares were used to compute the second derivative of the logistic equation and the cycle threshold, C-t, was determined from the maximum value of the second derivative. This C-t value was subsequently used to determine Delta Delta C-t and the amplification efficiency, E-n, thereby completing the analysis on a qRT-PCR data set. The robustness of the logistic approach was verified by testing similar to 600 F vs. C curves using both new and previously published data sets. In most cases, comparisons were made between the logistic estimates and those from the standard curve and comparative C-t methods. Deviations between the logistic and standard curve method ranged between 3-10% for C-t estimates, 2-10% for Delta Delta C-t estimates, and 1-11% for E-n estimates. The correlations between C-t estimates from the logistic and standard curve methods were very high, often > 0.95. When compared with five other established methods of qRT-PCR data analysis to predict initial concentrations of two genes encompassing a total of 500 F vs. C curves, the logistic estimates were of comparable accuracy. This reliable performance of the logistic approach comes without the need to construct standard curves which can be a laborious undertaking. Also, no a priori assumptions for E-n are necessary while some other methods assume equal E-n values for the reference and target genes, an assumption that is not universally valid. In addition, by accurately describing the data in the plateau region of the F vs. C curve, the logistic method overcomes the limitations of the sigmoidal curve fitting method. The streamlined nature of the logistic approach makes it ideal for complete automation on a variety of computing environments thereby completely eliminating user bias. The simplicity, robustness, and ease of computer implementation of the logistic approach should make it an attractive alternative for rapidly analyzing qRT-PCR data. (C) 2011 American Institute of Chemical Engineers Biotechnol. Prog., 27: 1407-1414, 2011
更多查看译文
关键词
gene expression analysis,logistic modeling,mammalian cell culture,qRT-PCR,relative quantification
AI 理解论文
溯源树
样例
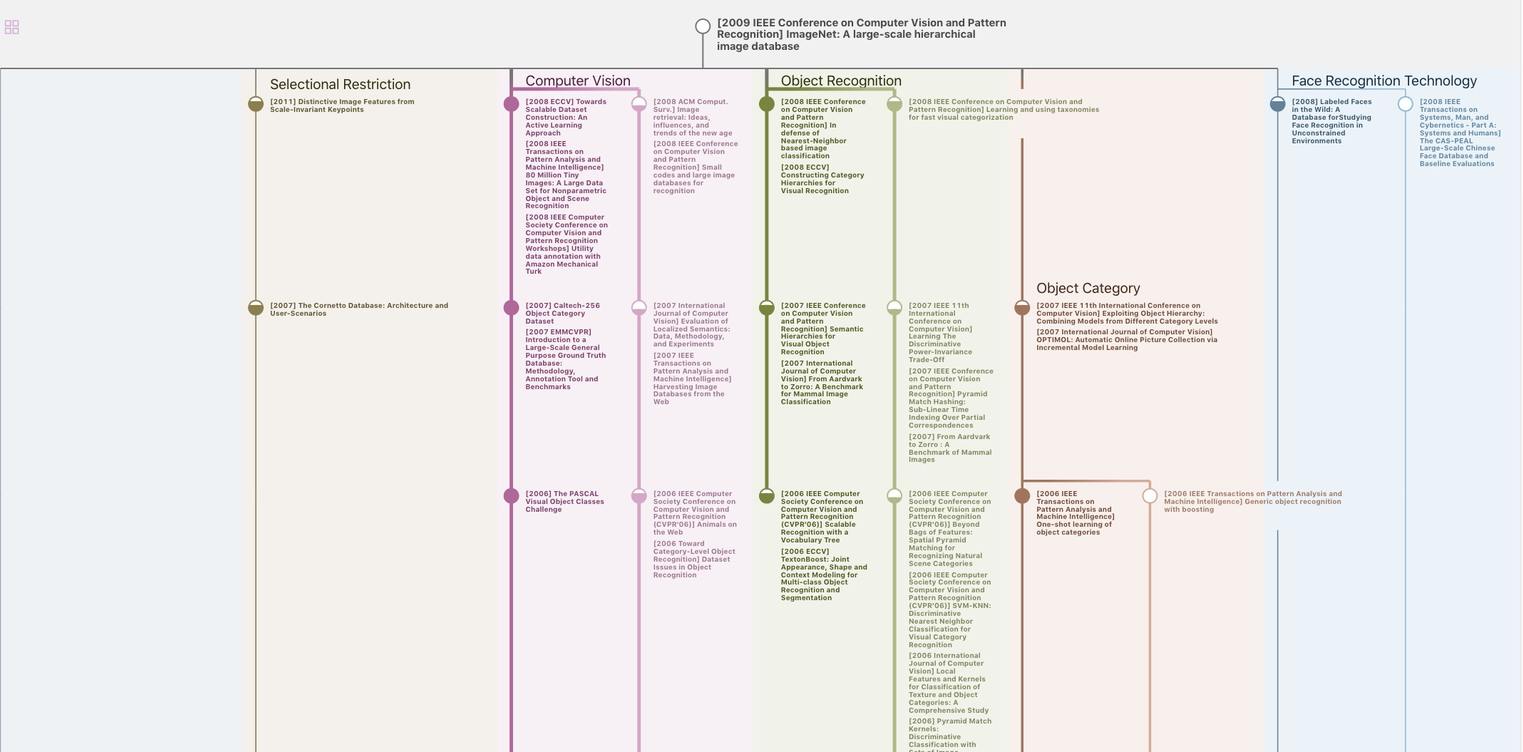
生成溯源树,研究论文发展脉络
Chat Paper
正在生成论文摘要