Virtual screening using molecular simulations.
PROTEINS-STRUCTURE FUNCTION AND BIOINFORMATICS(2011)
摘要
Effective virtual screening relies on our ability to make accurate prediction of protein-ligand binding, which remains a great challenge. In this work, utilizing the molecular-mechanics Poisson-Boltzmann (or Generalized Born) surface area approach, we have evaluated the binding affinity of a set of 156 ligands to seven families of proteins, trypsin beta, thrombin alpha, cyclin-dependent kinase (CDK), cAMP-dependent kinase (PKA), urokinase-type plasminogen activator, beta-glucosidase A, and coagulation factor Xa. The effect of protein dielectric constant in the implicit-solvent model on the binding free energy calculation is shown to be important. The statistical correlations between the binding energy calculated from the implicit-solvent approach and experimental free energy are in the range of 0.56-0.79 across all the families. This performance is better than that of typical docking programs especially given that the latter is directly trained using known binding data whereas the molecular mechanics is based on general physical parameters. Estimation of entropic contribution remains the barrier to accurate free energy calculation. We show that the traditional rigid rotor harmonic oscillator approximation is unable to improve the binding free energy prediction. Inclusion of conformational restriction seems to be promising but requires further investigation. On the other hand, our preliminary study suggests that implicit-solvent based alchemical perturbation, which offers explicit sampling of configuration entropy, can be a viable approach to significantly improve the prediction of binding free energy. Overall, the molecular mechanics approach has the potential for medium to high-throughput computational drug discovery. Proteins 2011; 79:1940-1951. (C) 2011 Wiley-Liss, Inc.
更多查看译文
关键词
molecular mechanics,MM-GBSA,MM-PBSA,dielectric constant,configurational entropy,alchemical free energy calculation
AI 理解论文
溯源树
样例
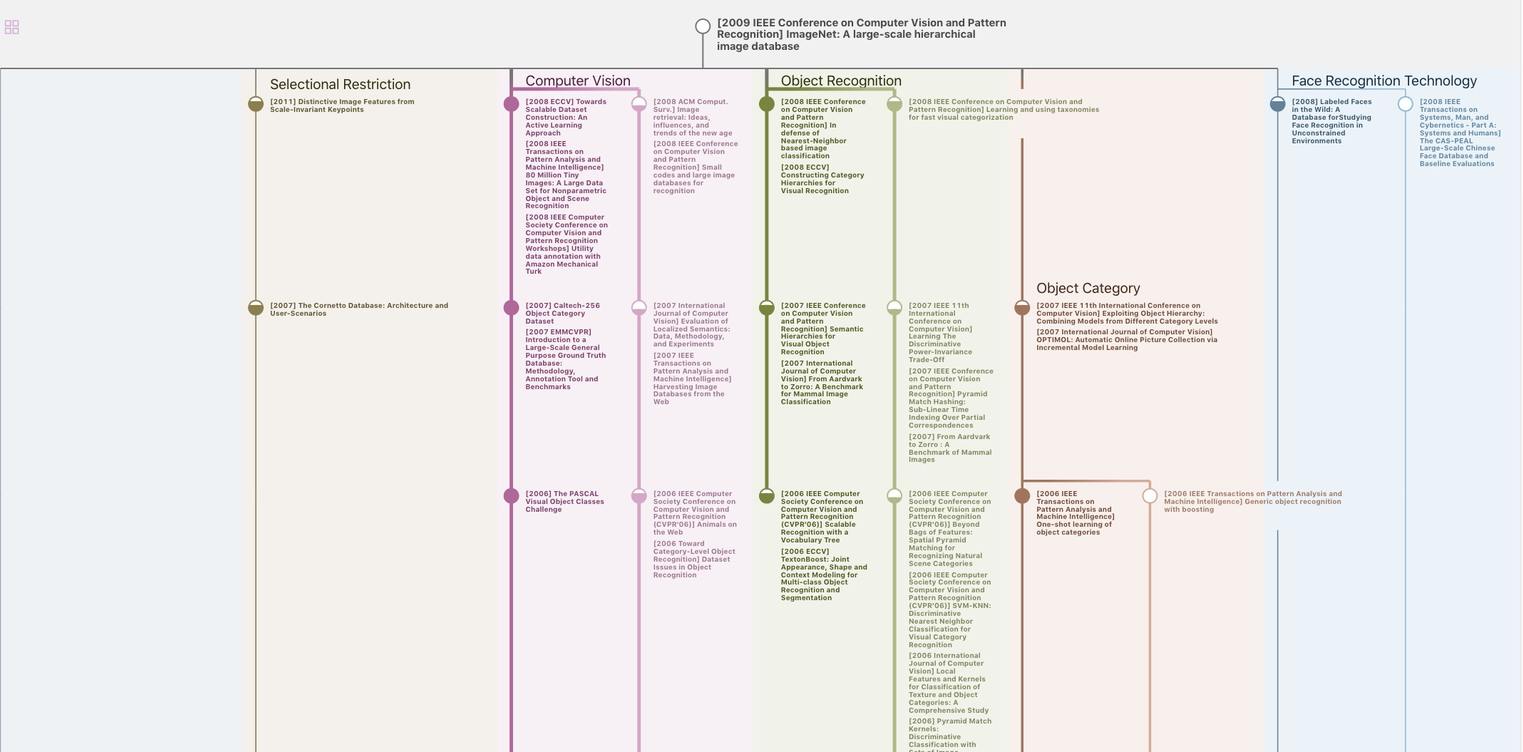
生成溯源树,研究论文发展脉络
Chat Paper
正在生成论文摘要