State of health estimation for lithium-ion batteries based on two-stage features extraction and gradient boosting decision tree
Energy(2023)
摘要
To ensure the stable, reliable, and safe operation of lithium-ion batteries, the state of health (SOH) serves as a crucial basis for battery safety monitoring and operational maintenance. However, the complex working environment of batteries leads to the presence of a significant amount of noise data, abnormal data, and data discontinuities, posing challenges for battery feature extraction and SOH estimation. To address this issue, this paper proposes a two-stage transformation-based feature extraction method combined with the gradient boosting decision tree (GBDT) algorithm. The two-stage transformation feature extraction method utilizes singular spectrum analysis (SSA) and fast Fourier transform (FFT) to extract time-domain and frequency-domain health features from the battery data, respectively. The GBDT ensemble learning algorithm is employed to estimate the SOH of lithium-ion batteries, leveraging the capability to effectively uncover and learn relevant features by combining multiple weak learners into a strong model. Furthermore, correlation analysis and ablation experiments are conducted to validate the effectiveness of the two-stage feature extraction method. Additionally, four sets of comparative experiments are designed to demonstrate the superior performance of the GBDT method compared to four other machine learning methods. The experimental results show that the mean absolute error (MAE) and root mean square error (RMSE) of SOH estimation using the proposed method do not exceed 3 % on the NASA and CALCE datasets.
更多查看译文
关键词
State of health,Two-stage transformation,Ensemble learning,Gradient boosting decision tree,Lithium-ion batteries
AI 理解论文
溯源树
样例
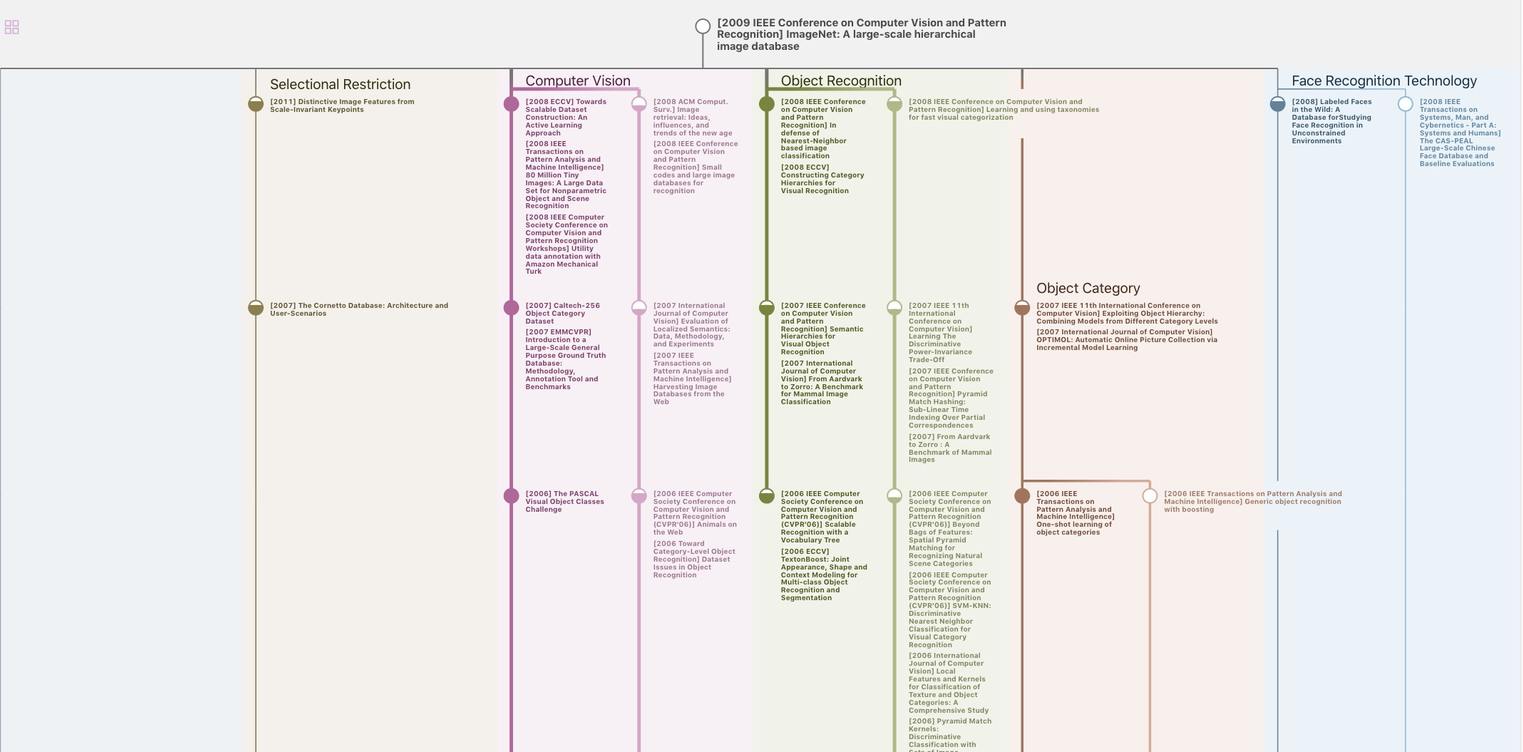
生成溯源树,研究论文发展脉络
Chat Paper
正在生成论文摘要