Statistical Analysis Of Tensor Fields
MEDICAL IMAGE COMPUTING AND COMPUTER-ASSISTED INTERVENTION - MICCAI 2010, PT I(2010)
摘要
In this paper, we propose a Riemannian framework for statistical analysis of tensor fields. Existing approaches to this problem have been mainly voxel-based that overlook the correlation between tensors at different voxels. In our approach, the tensor fields are considered as points in a high-dimensional Riemannian product space and accordingly, we extend Principal Geodesic Analysis (PGA) to the product space. This provides us with a principled method for linearizing the problem, and coupled with the usual log-exp maps that relate points on manifold to tangent vectors, the global correlation of the tensor field can be captured using Principal Component Analysis in a tangent space. Using the proposed method, the modes of variation of tensor fields can be efficiently determined, and dimension reduction of the data is also easily implemented. Experimental results on characterizing the variation of a large set of tensor fields are presented in the paper, and results on classifying tensor fields using the proposed method are also reported. These preliminary experimental results demonstrate the advantages of our method over the voxel-based approach.
更多查看译文
关键词
tensor field,proposed method,principled method,high-dimensional Riemannian product space,product space,tangent space,Principal Component Analysis,Principal Geodesic Analysis,Riemannian framework,experimental result,Statistical analysis
AI 理解论文
溯源树
样例
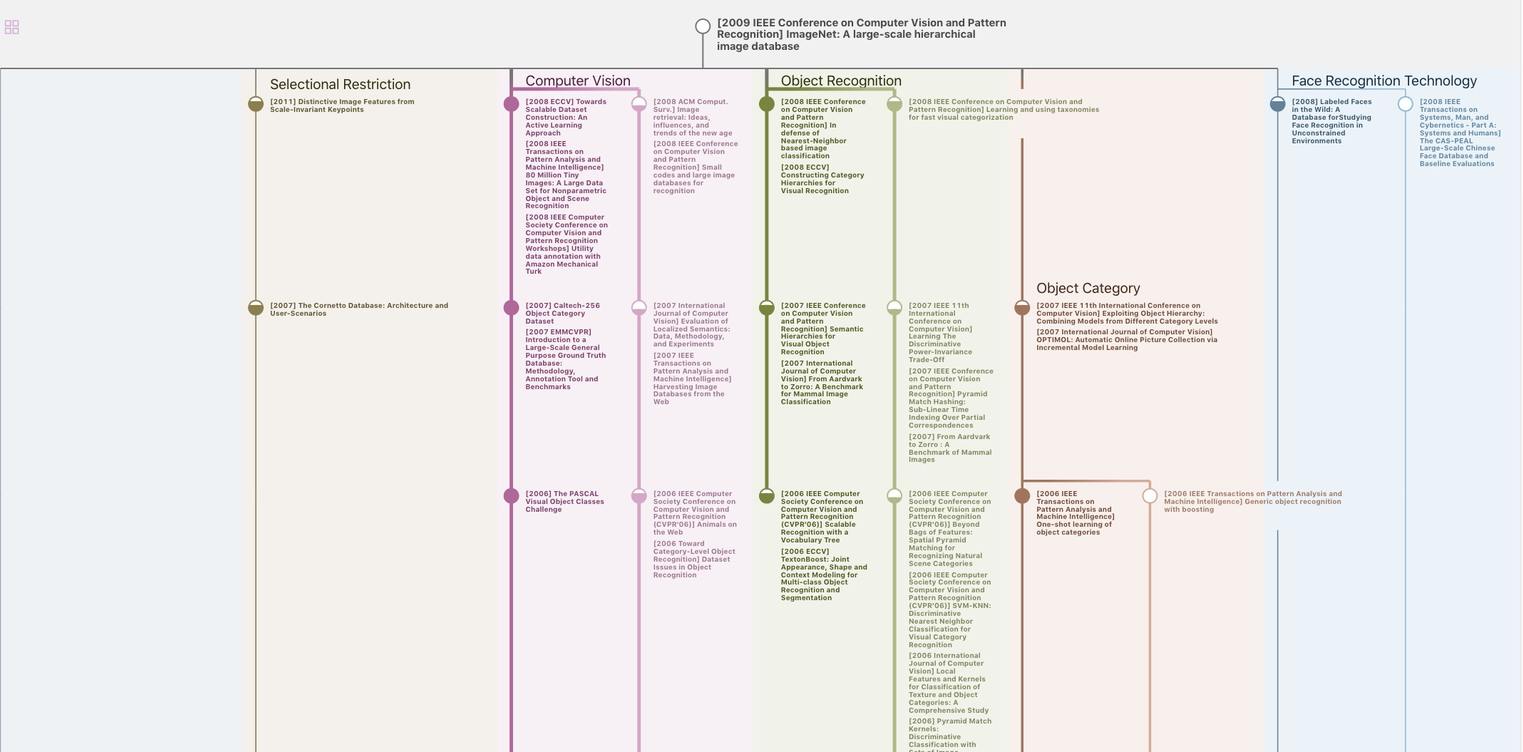
生成溯源树,研究论文发展脉络
Chat Paper
正在生成论文摘要