User-Entity Differential Privacy in Learning Natural Language Models
arxiv(2022)
摘要
In this paper, we introduce a novel concept of user-entity differential privacy (UeDP) to provide formal privacy protection simultaneously to both sensitive entities in textual data and data owners in learning natural language models (NLMs). To preserve UeDP, we developed a novel algorithm, called UeDP-Alg, optimizing the trade-off between privacy loss and model utility with a tight sensitivity bound derived from seamlessly combining user and sensitive entity sampling processes. An extensive theoretical analysis and evaluation show that our UeDP-Alg outperforms baseline approaches in model utility under the same privacy budget consumption on several NLM tasks, using benchmark datasets.
更多查看译文
关键词
Differential privacy,natural language models,entities,user identity
AI 理解论文
溯源树
样例
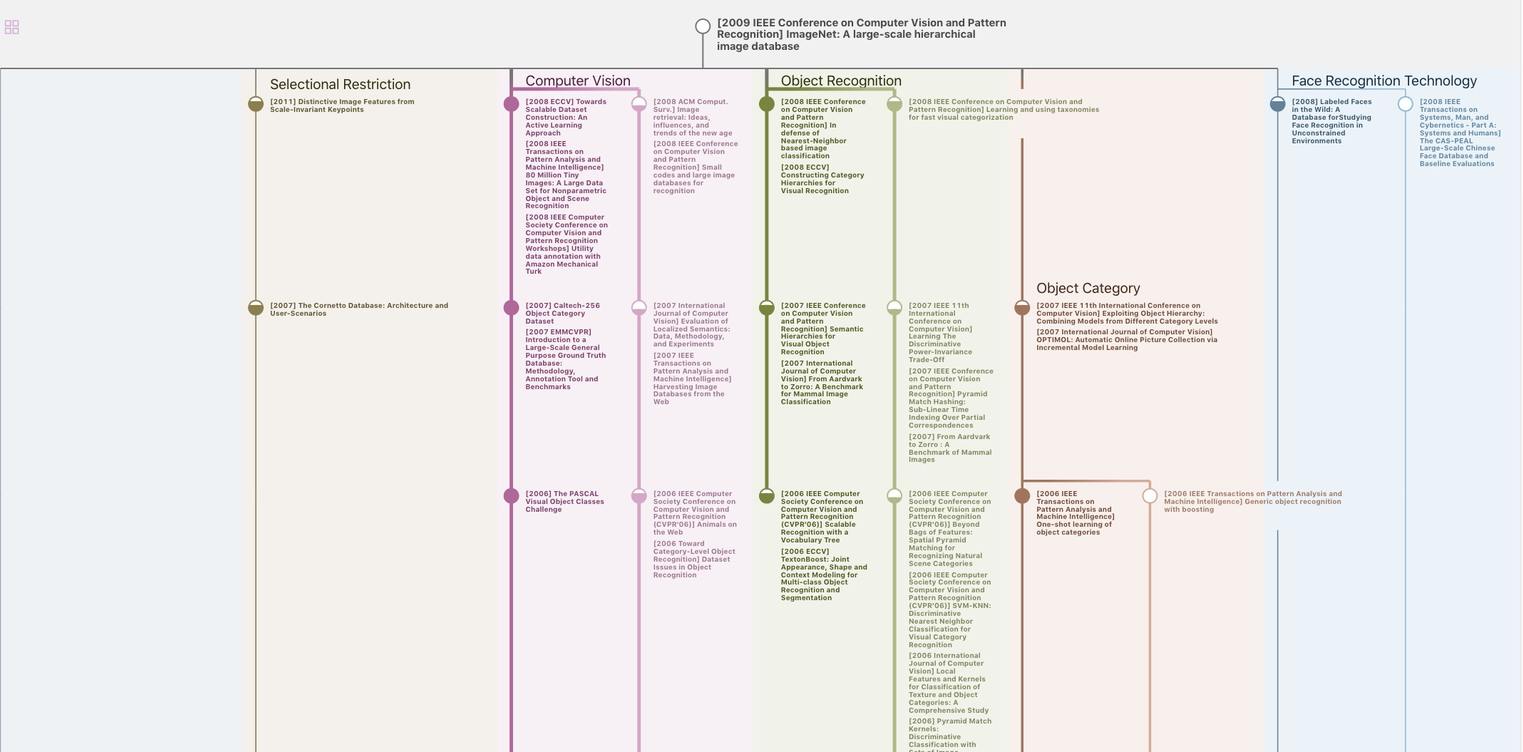
生成溯源树,研究论文发展脉络
Chat Paper
正在生成论文摘要