Multi-label fast marching and seeded watershed segmentation methods for diagnosis of breast cancer cytology.
EMBC(2013)
摘要
Digital cytology plays an increasingly important role in breast cancer diagnosis. However, analysis of cytologic images is a very difficult task. Especially, nuclei segmentation is extremely challenging. In our work on fully automated medical diagnosis system we encountered the problem of densely clustered nuclei. We decided to use a segmentation algorithm that is rather rarely found in the literature. Multi-label fast marching was applied and compared to well-known and extensively used seeded watershed algorithm. In both methods, it is critical to determine the appropriate starting points (seeds). The seeds were determined using a combination of adaptive thresholding in grayscale, clustering in color space and conditional erosion. The proposed segmentation procedure was tested for suitability for diagnosis of the cancer. Experiments were conducted on a set of 450 microscopic images of fine needle biopsies obtained from patients of the Regional Hospital in Zielona Góra, Poland. The images were classified as either benign or malignant using 84 features extracted from isolated nuclei. Both methods gave very promising results and showed that our method is effective and can be successfully applied for computer-aided diagnosis system.
更多查看译文
关键词
cellular biophysics,image classsification,seeded watershed segmenation algorithm,image segmentation,color space clustering,computer-aided diagnosis system,nuclei segmentation,breast cancer cytology diagnosis,adaptive thresholding,automated medical diagnosis system,cancer,feature extraction,image classification,fine needle biopsies,grayscale,cytologic image analysis,multilabel fast marching method,medical image processing,erosion clustering,biomedical imaging,breast cancer,accuracy
AI 理解论文
溯源树
样例
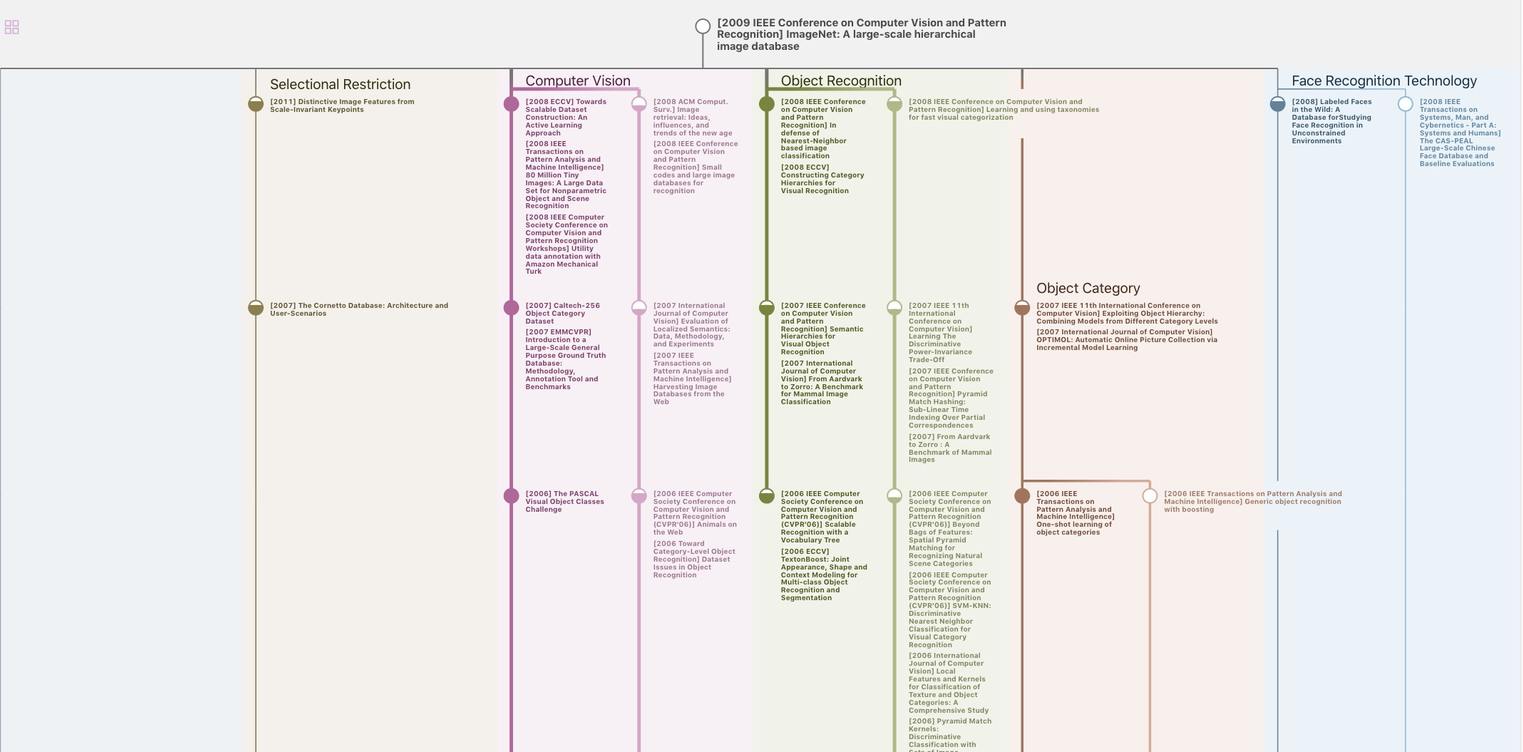
生成溯源树,研究论文发展脉络
Chat Paper
正在生成论文摘要