Space-Time Super-Resolution Using Graph-Cut Optimization
Pattern Analysis and Machine Intelligence, IEEE Transactions(2011)
摘要
We address the problem of super-resolution—obtaining high-resolution images and videos from multiple low-resolution inputs. The increased resolution can be in spatial or temporal dimensions, or even in both. We present a unified framework which uses a generative model of the imaging process and can address spatial super-resolution, space-time super-resolution, image deconvolution, single-image expansion, removal of noise, and image restoration. We model a high-resolution image or video as a Markov random field and use maximum a posteriori estimate as the final solution using graph-cut optimization technique. We derive insights into what super-resolution magnification factors are possible and the conditions necessary for super-resolution. We demonstrate spatial super-resolution reconstruction results with magnifications higher than predicted limits of magnification. We also formulate a scheme for selective super-resolution reconstruction of videos to obtain simultaneous increase of resolutions in both spatial and temporal directions. We show that it is possible to achieve space-time magnification factors beyond what has been suggested in the literature by selectively applying super-resolution constraints. We present results on both synthetic and real input sequences.
更多查看译文
关键词
Markov processes,graph theory,image resolution,optimisation,Markov random field,graph cut optimization,image deconvolution,image restoration,imaging process,space time super resolution,spatial dimensions,spatial super resolution,temporal dimensions,Markov random field (MRF),Super-resolution,graph-cut,maximum a posteriori (MAP),minimization.,nonlinear,space-time
AI 理解论文
溯源树
样例
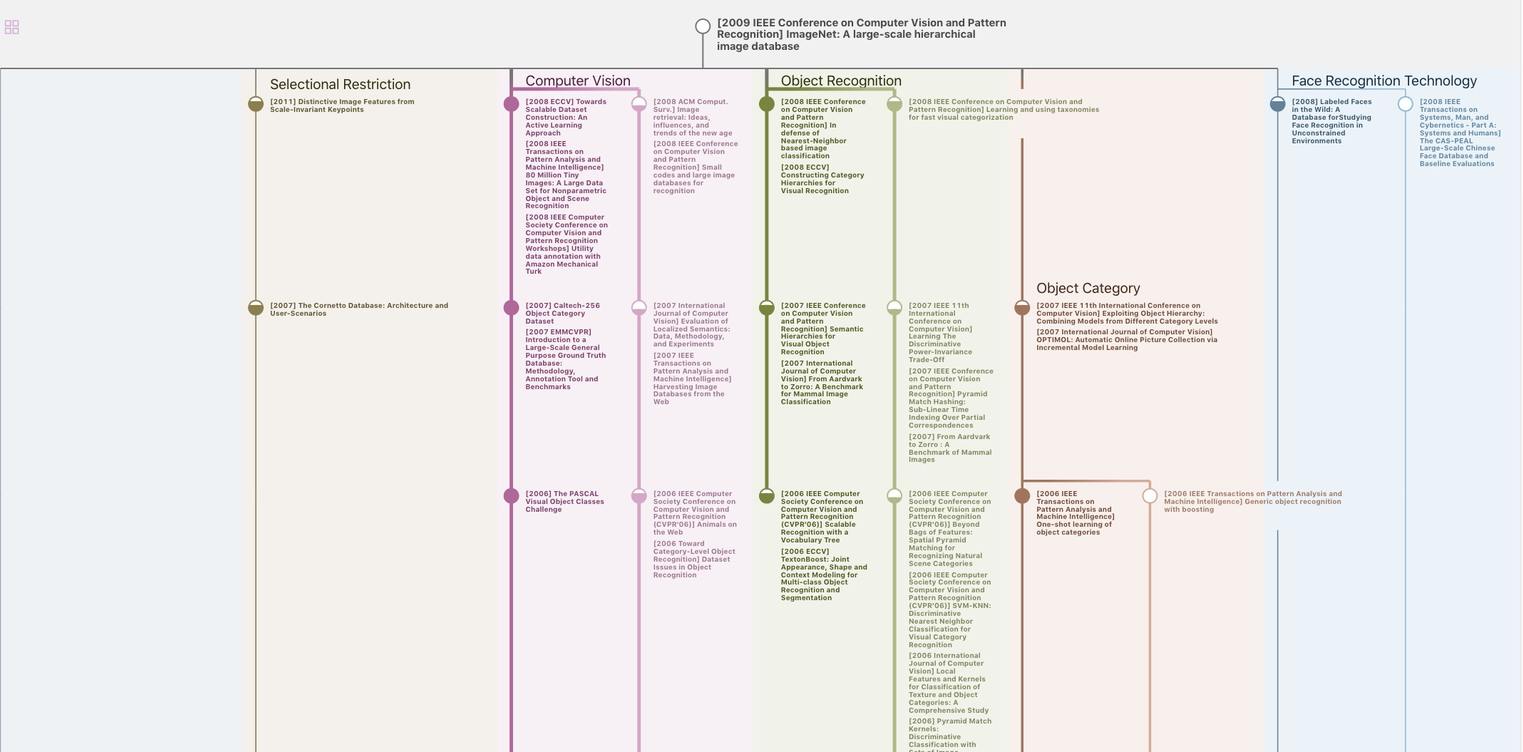
生成溯源树,研究论文发展脉络
Chat Paper
正在生成论文摘要