Traffic sign classification with Quantized Local Zernike Moments
Signal Processing and Communications Applications Conference(2013)
摘要
In this paper, it is shown that Local Zernike Moments (LZM), applied successfully to face recognition, can also be used successfully for the classification of traffic signs. The direct usage of the images produced by LZM is not very efficient in terms of computation time. So, a new method, named Quantized Local Zernike Moments (QLZM), is developed. QLZM has an advantage of reducing the number of images in LZM representation by packaging the binarized images of LZM. In addition, Zernike Moments (ZM) and Hue histogram are also used in conjunction with QLZM. By using only QLZM, 97.34% accuracy is achieved and by using only ZM, 93.74% accuracy is achieved. With the usage of QLZM, ZM feature vectors and Hue histogram together, 97.62% success rate is achieved. In this classification processes, the GTSRB dataset is used, which is widely preferred for the classification of traffic signs.
更多查看译文
关键词
Zernike polynomials,feature extraction,image classification,traffic engineering computing,GTSRB dataset,Hue histogram,QLZM,ZM feature vectors,binarized image packaging,quantized local Zernike moments,traffic sign classification,Zernike moments,image classification,local Zernike moments,traffic sign classification
AI 理解论文
溯源树
样例
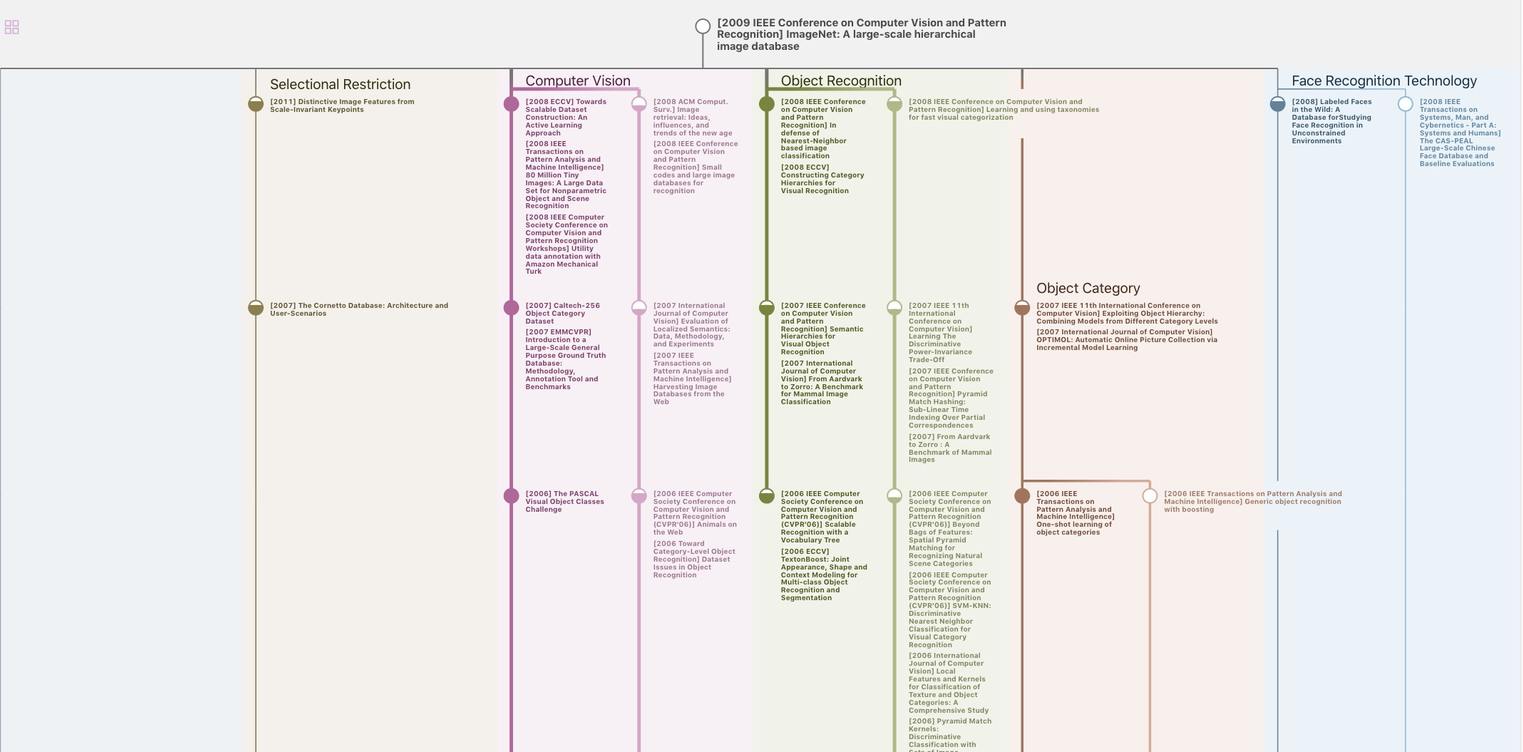
生成溯源树,研究论文发展脉络
Chat Paper
正在生成论文摘要