Tracking Conductivity Variations in the Absence of Accurate State Evolution Models in Electrical Impedance Tomography
Bioinformatics and Biomedical Engineering(2010)
摘要
We present results on both linear and non-linear approaches in tracking conductivity variations in electrical impedance tomography. Throughout this study, we use both synthetic and measured data. The true system dynamics is considered as unknown and modelled as a random walk. In the linear reconstructions, the time evolution model is augmented with a Gaussian smoothness prior and results are shown using two different models for the covariance matrix of the process noise. Furthermore, we compare the reconstructions of the one step Gauss-Newton method to the Kalman filter on measured data from an adult human subject. In the non-linear study, we compare the performance of the extended Kalman filter against the particle filter on a simple test case. It is observed that the particle filter shows superior performance in tracking nonlinear/non-Gaussian conductivity variations.
更多查看译文
关键词
gaussian processes,kalman filters,electric impedance imaging,medical signal processing,gaussian smoothness,kalman filter,accurate state evolution models,adult human subject,covariance matrix,electrical impedance tomography,linear reconstructions,particle filter,process noise,random walk,step gauss-newton method,time evolution model,tracking nongaussian conductivity variation,tracking nonlinear conductivity variation,true system dynamics,conductivity,tomography,system dynamics,gaussian noise,impedance,extended kalman filter,newton method,image reconstruction,mathematical model,particle filters
AI 理解论文
溯源树
样例
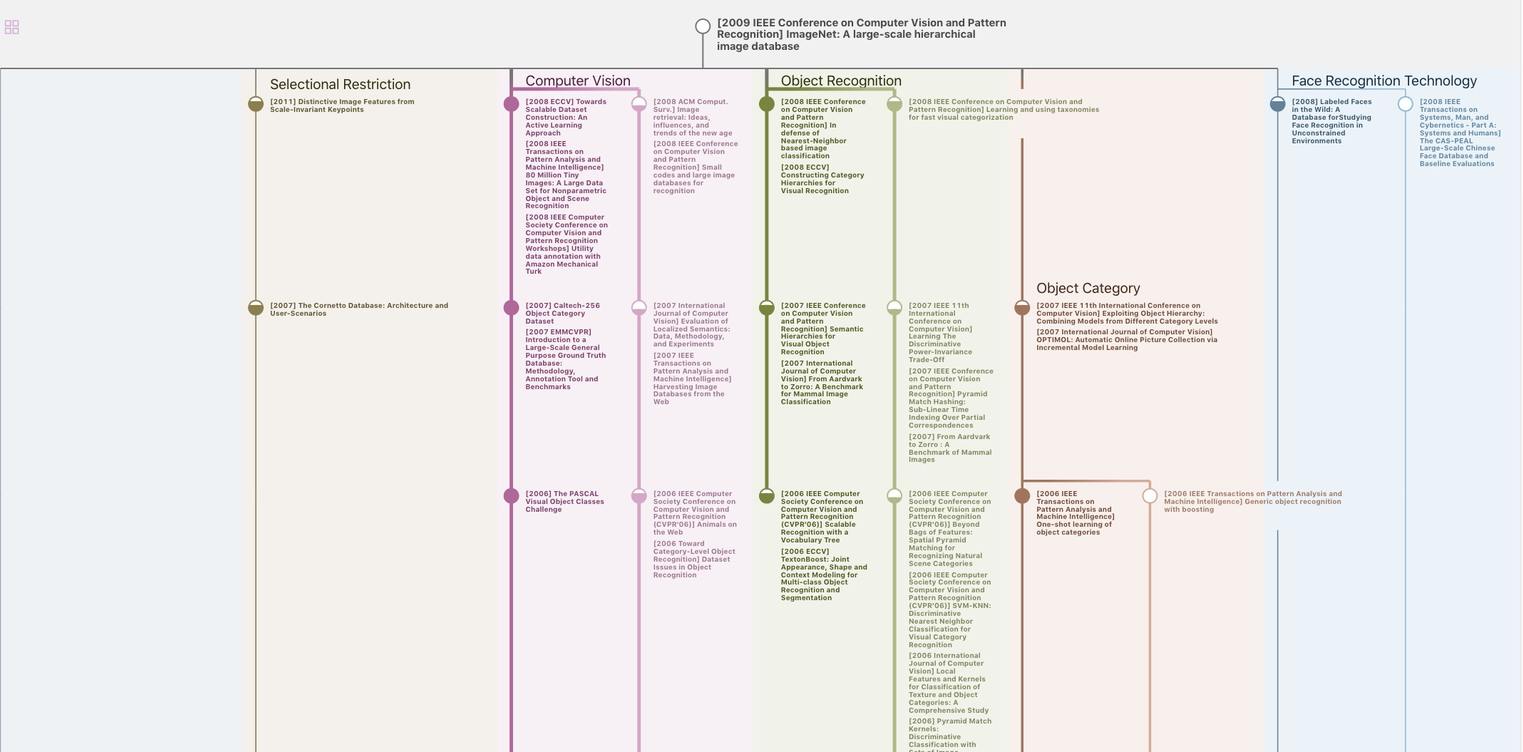
生成溯源树,研究论文发展脉络
Chat Paper
正在生成论文摘要