Context-GMM: Incremental learning of sparse priors for Gaussian mixture regression
Robotics and Biomimetics(2012)
摘要
Gaussian Mixture Models have been widely used in robotic control and in sensory anticipation applications. A mixture model is learnt from demonstrations and later used to infer the most likely control signals, or is also used as a forward model to predict the change in sensory signals over time. However, such models often are too big to be tractable in real-time applications. In this paper we introduce the Context-GMM, a method to learn sparse priors over the mixture components. Such priors are stable over large amounts of time and provide a way of selecting very small subsets of mixture components without significant loss in accuracy and with huge computational savings.
更多查看译文
关键词
Gaussian processes,learning (artificial intelligence),prediction theory,real-time systems,regression analysis,set theory,Gaussian mixture regression,change prediction,computational savings,context-GMM,control signals,incremental learning,mixture components,real-time applications,robotic control,sensory anticipation applications,sparse priors
AI 理解论文
溯源树
样例
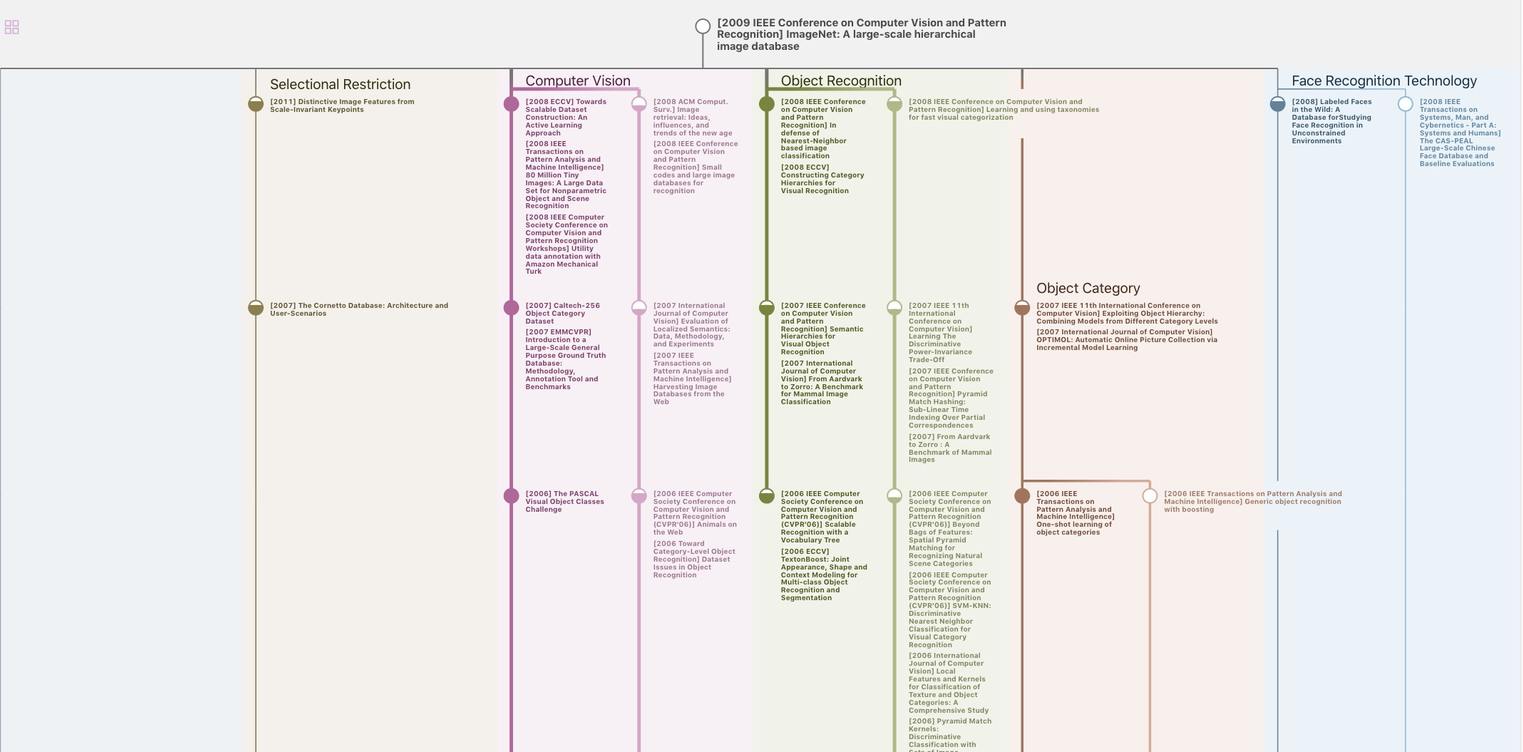
生成溯源树,研究论文发展脉络
Chat Paper
正在生成论文摘要