Region-based Convolutional Networks for Accurate Object Detection and Segmentation
Pattern Analysis and Machine Intelligence, IEEE Transactions(2016)
摘要
Object detection performance, as measured on the canonical PASCAL VOC Challenge datasets, plateaued in the final years of the competition. The best-performing methods were complex ensemble systems that typically combined multiple low-level image features with high-level context. In this paper, we propose a simple and scalable detection algorithm that improves mean average precision (mAP) by more than 50% relative to the previous best result on VOC 2012—achieving a mAP of 62.4%. Our approach combines two ideas: (1) one can apply high-capacity convolutional networks (CNNs) to bottom-up region proposals in order to localize and segment objects and (2) when labeled training data are scarce, supervised pre-training for an auxiliary task, followed by domain-specific fine-tuning, boosts performance significantly. Since we combine region proposals with CNNs, we call the resulting model an R-CNN or Region-based Convolutional Network. Source code for the complete system is available at http://www.cs.berkeley.edu/rbg/rcnn.
更多查看译文
关键词
convolutional networks,deep learning,detection,object recognition,semantic segmentation,transfer learning,support vector machines,detectors,image segmentation,feature extraction
AI 理解论文
溯源树
样例
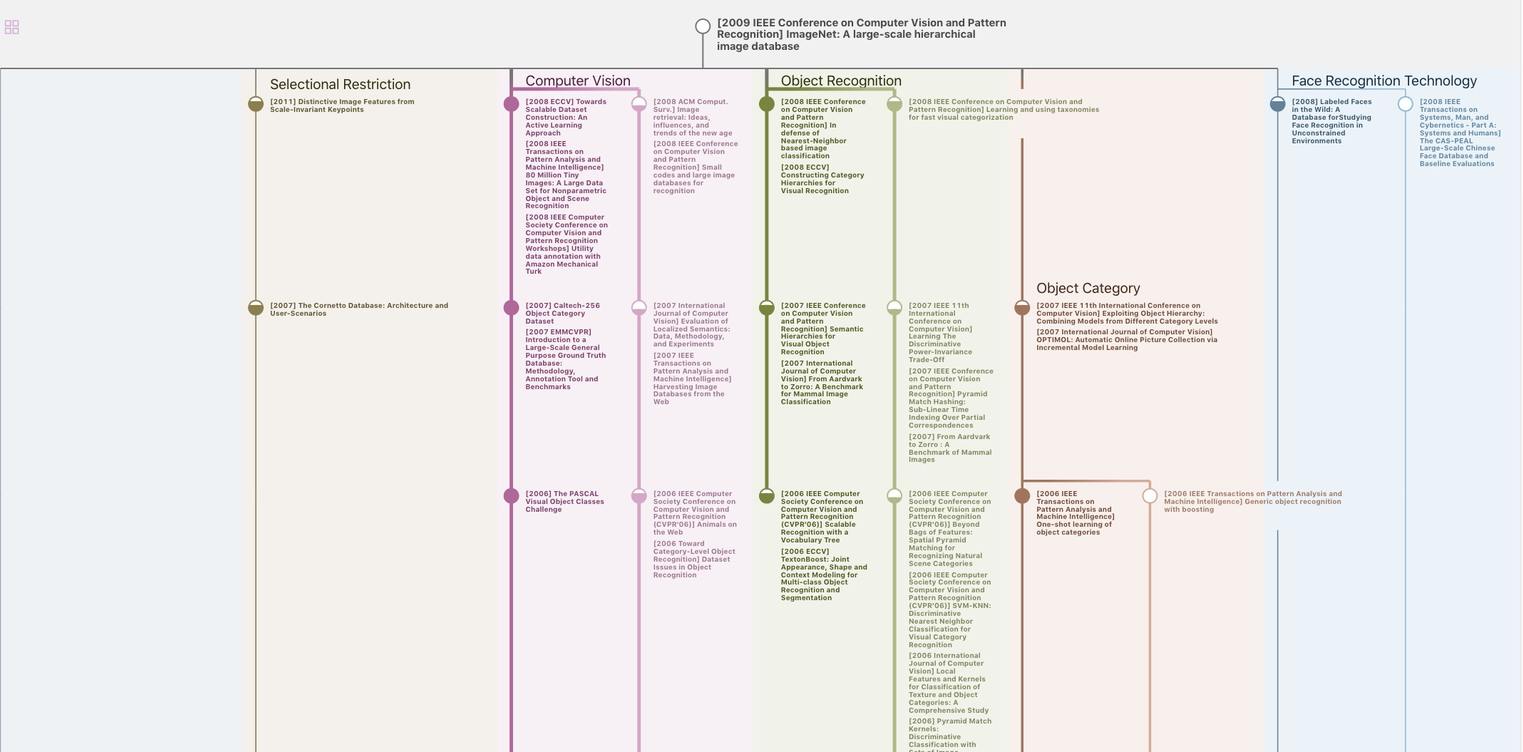
生成溯源树,研究论文发展脉络
Chat Paper
正在生成论文摘要