A MULTIVARIATE GAUSSIAN MIXTURE MODEL OF LINEAR PREDICTION ERROR FOR COLOUR TEXTURE SEGMENTATION
European Signal Processing Conference(2009)
摘要
This paper presents an algorithm for parametric supervised colour texture segmentation using a novel image observa- tion model. The proposed segmentation algorithm consists of two phases: In the first phase, we estimate an initial class label field of the image based on a 2D multichannel complex linear prediction model. Information of both luminance and chrominance spatial variation feature cues are used to charac- terize colour textures. Complex multichannel version of 2D Quarter Plane Autoregressive model is used to model these spatial variations of colour texture images in CIE L*a*b* colour space. Overall colour distribution of the image is es- timated from the multichannel prediction error sequence of this Autoregressive model. Another significant contribution of this paper is the modelling of this multichannel error se- quence using Multivariate Gaussian Mixture Model instead of a single Gaussian probability. Gaussian parameters are calculated through Expectation Maximization on a training dataset. In second phase of the algorithm, initial class label field obtained through the first stage is spatially regularized by ICM algorithm to have the final segmented image. Visual and quantitative results for different number of components of Multivariate Gaussian Mixture Model are presented and discussed.
更多查看译文
关键词
Gaussian processes,autoregressive processes,image colour analysis,image segmentation,image texture,mixture models,2D multichannel complex linear prediction model,2D quarter plane autoregressive model,CIE L*a*b* colour space,ICM algorithm,colour texture segmentation,expectation maximization,image observation model,linear prediction error,multichannel prediction error sequence,multivariate Gaussian mixture model,
AI 理解论文
溯源树
样例
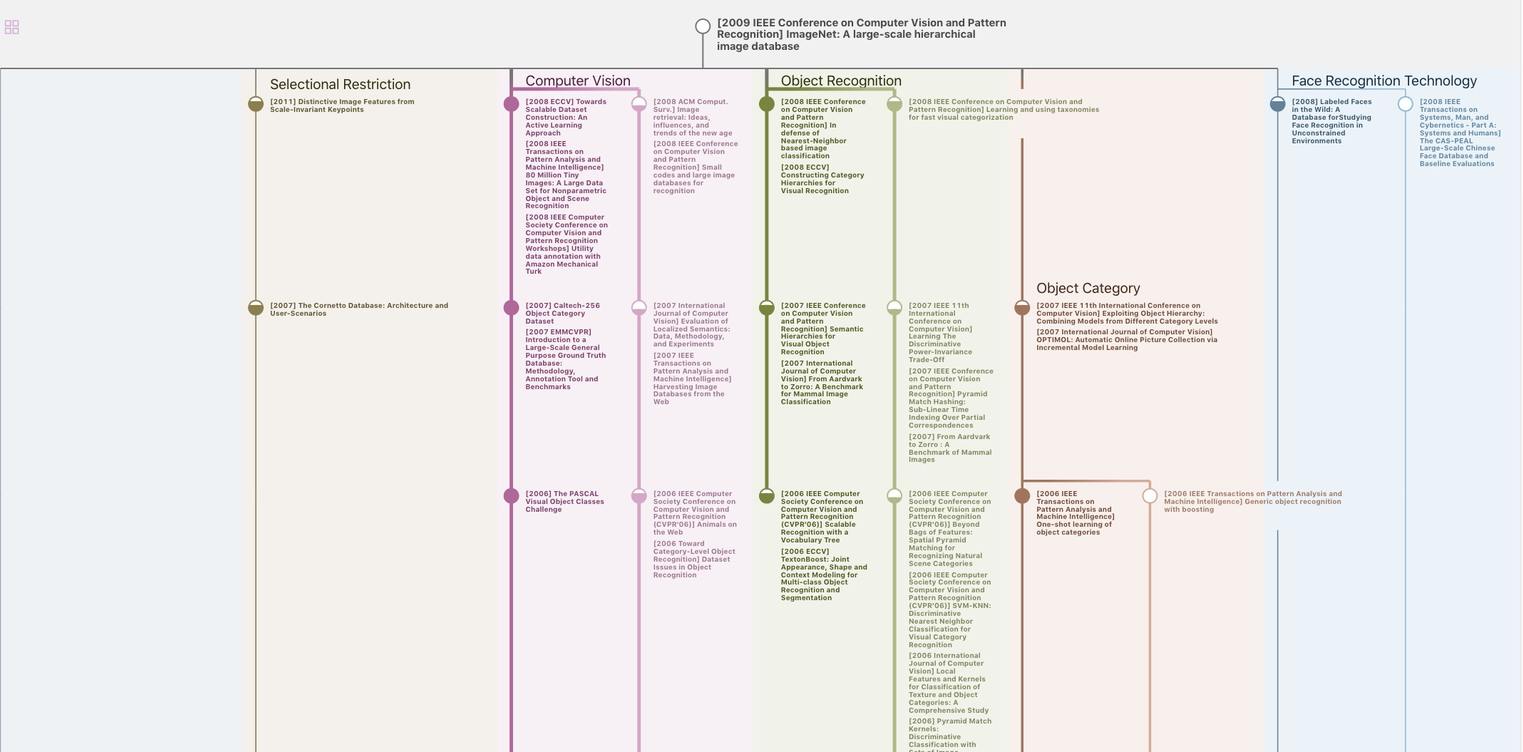
生成溯源树,研究论文发展脉络
Chat Paper
正在生成论文摘要