Classification with invariant scattering representations
Ithaca, NY(2011)
摘要
A scattering transform defines a signal representation which is invariant to translations and Lipschitz continuous relatively to deformations. It is implemented with a non-linear convolution network that iterates over wavelet and modulus operators. Lipschitz continuity locally linearizes deformations. Complex classes of signals and textures can be modeled with low-dimensional affine spaces, computed with a PCA in the scattering domain. Classification is performed with a penalized model selection. State of the art results are obtained for handwritten digit recognition over small training sets, and for texture classification.
更多查看译文
关键词
image classification,transforms,Lipschitz continuity,PCA,handwritten digit recognition,image classification,invariant scattering transform representation,modulus operator,nonlinear convolution network,signal representation,Image classification,Invariant representations,local image descriptors,pattern recognition,texture classification
AI 理解论文
溯源树
样例
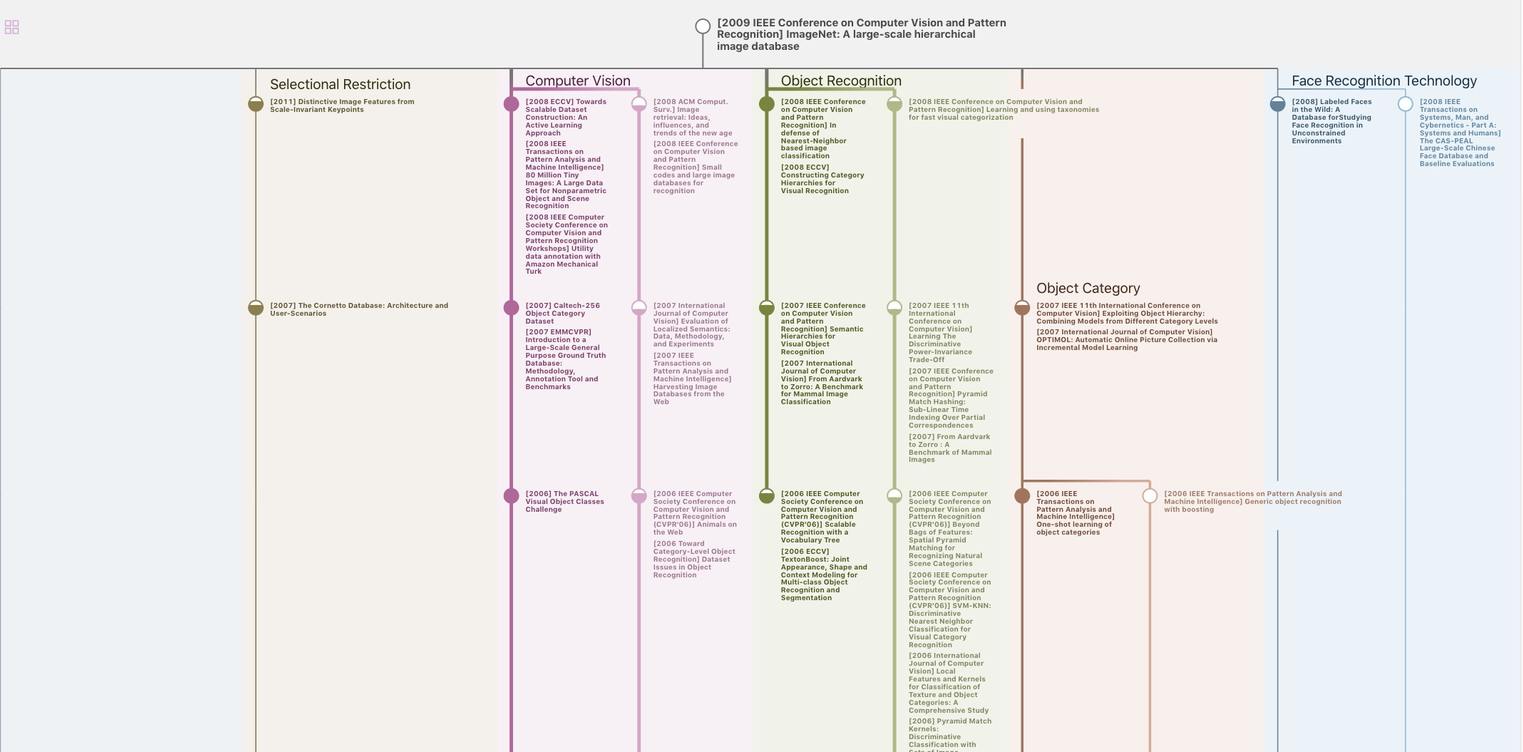
生成溯源树,研究论文发展脉络
Chat Paper
正在生成论文摘要