Relative Hidden Markov Models for Video-Based Evaluation of Motion Skills in Surgical Training
Pattern Analysis and Machine Intelligence, IEEE Transactions(2015)
摘要
A proper temporal model is essential to analysis tasks involving sequential data. In computer-assisted surgical training, which is the focus of this study, obtaining accurate temporal models is a key step towards automated skill-rating. Conventional learning approaches can have only limited success in this domain due to insufficient amount of data with accurate labels. We propose a novel formulation termed Relative Hidden Markov Model and develop algorithms for obtaining a solution under this formulation. The method requires only relative ranking between input pairs, which are readily available from training sessions in the target application, hence alleviating the requirement on data labeling. The proposed algorithm learns a model from the training data so that the attribute under consideration is linked to the likelihood of the input, hence supporting comparing new sequences. For evaluation, synthetic data are first used to assess the performance of the approach, and then we experiment with real videos from a widely-adopted surgical training platform. Experimental results suggest that the proposed approach provides a promising solution to video-based motion skill evaluation. To further illustrate the potential of generalizing the method to other applications of temporal analysis, we also report experiments on using our model on speech-based emotion recognition.
更多查看译文
关键词
emotion recognition,relative hidden markov model,relative learning,surgical skill,temporal model,speech recognition,training data,hidden markov models,synthetic data,computational modeling,surgery,data models
AI 理解论文
溯源树
样例
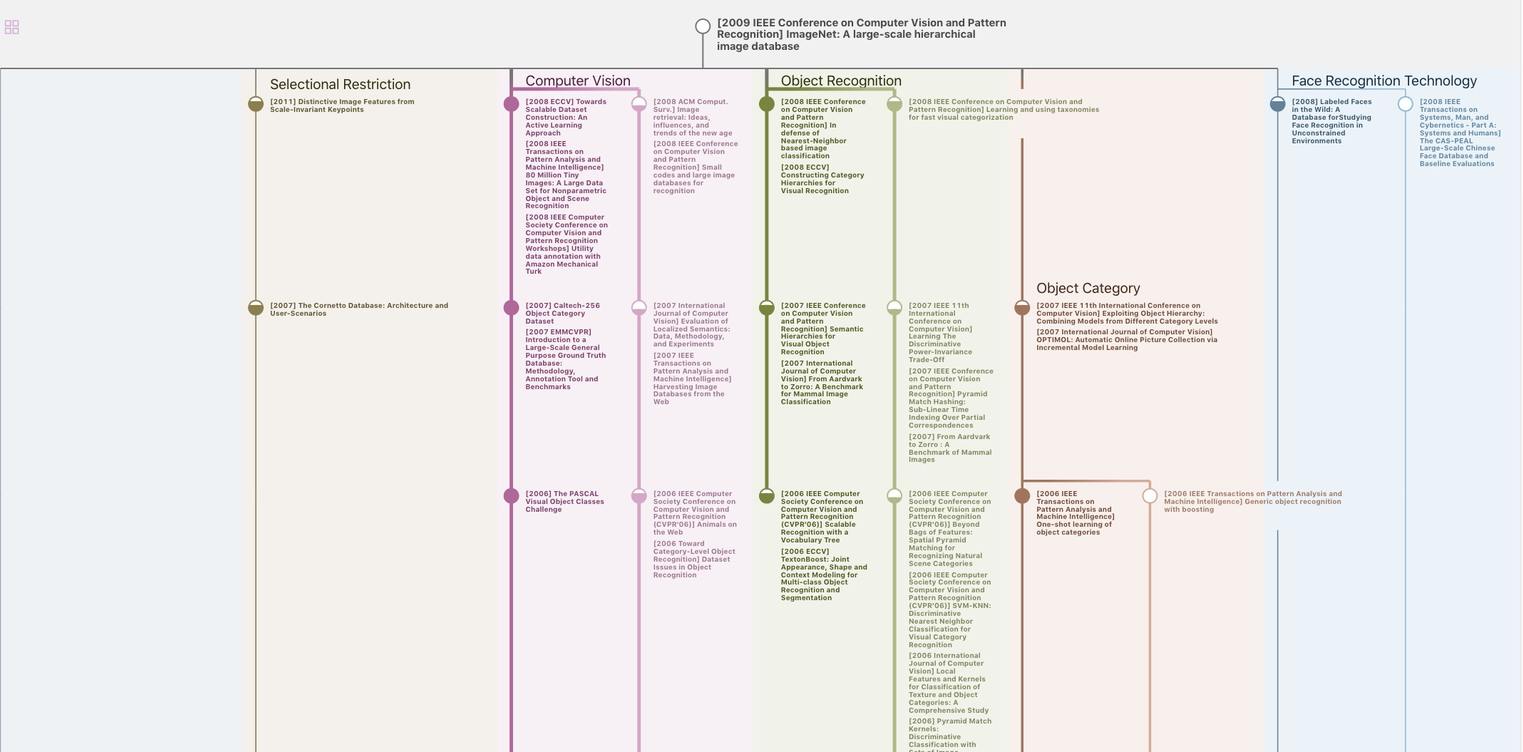
生成溯源树,研究论文发展脉络
Chat Paper
正在生成论文摘要