Well-calibrated heavy tailed Bayesian speaker verification for microphone speech
Acoustics, Speech and Signal Processing(2011)
摘要
The work presented in this paper is an extension of our two previous works. In the first paper, we proposed a low dimensional feature (i-vectors) extractor which is suit able for both telephone and microphone data of the NIST speaker recognition evaluation dataset. The second paper introduces the use of Probabilistic Linear Discriminant Analysis (PLDA) framework with a heavy tailed distribution for speaker verification. The advantage of PLDA comes from the fact that it does not require eigenchannel modelization nor scores normalization. However, this approach is only known for its success on telephone data speech but not for micro phone data. We propose to overcome this drawback by using PLDA as a second pass at the front-end feature extraction as well as a classifier. We present results on female speakers for the interview-interview condition in NIST2010 SRE. As measured by equal error rate (ERR) and NIST detection cost function (DCF), results with raw scores are 17% better than with score normalization. We have also calibrated our scores and we achieve a minimum and an actual DCF respectively of 0.559 and 0.607.
更多查看译文
关键词
belief networks,feature extraction,microphones,speaker recognition,DCF,ERR,NIST speaker recognition evaluation dataset,PLDA framework,detection cost function,equal error rate,front-end feature extraction,microphone speech,probabilistic linear discriminant analysis framework,well-calibrated heavy tailed Bayesian speaker verification,Probabilistic Linear Discriminant Analysis,Speaker verification,heavy tailed distribution,i-vectors
AI 理解论文
溯源树
样例
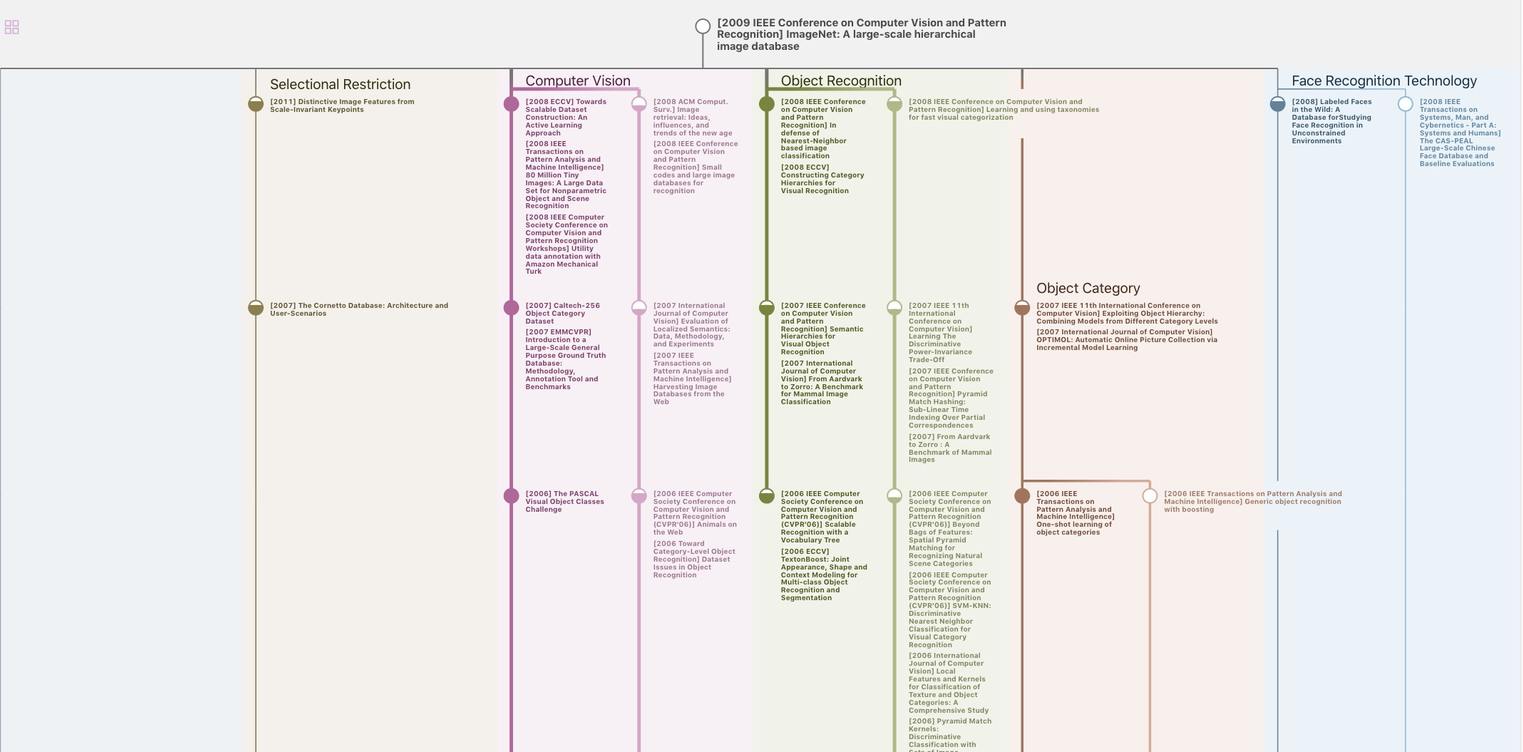
生成溯源树,研究论文发展脉络
Chat Paper
正在生成论文摘要