Image-based building reconstruction with Manhattan-world assumption
Pattern Recognition(2011)
摘要
The 3D reconstruction of buildings is a challenging research problem especially for image-based methods due to the absence of textured surfaces and difficulty in detecting high-level architectural structures. In this paper, we present an image-based reconstruction algorithm for efficiently modeling of buildings with the Manhattan-world assumption. The first key component of the algorithm is a clustering of geometric primitives (e.g. stereo points and lines) into sparse planes in Manhattan-world coordinates. The combination of such clustered planes greatly limits the possibility of building models to be reconstructed. In the second stage, we employ the graph-cut minimization to obtain an optimal model based on an energy functional that embeds image consistency, surface smoothness and Manhattanworld constraints. Real world building reconstruction results demonstrate the efficiency of the proposed algorithm in handling large scale data and its robustness against the variety of architectural structures.
更多查看译文
关键词
architecture,buildings (structures),civil engineering computing,geometry,graph theory,image reconstruction,pattern clustering,3D building reconstruction,Manhattan world constraints,Manhattan-world assumption,Manhattan-world coordinates,architectural structures,energy functional,geometric primitive clustering,graph-cut minimization,image consistency,image-based building reconstruction,image-based methods,optimal model,sparse planes,surface smoothness,
AI 理解论文
溯源树
样例
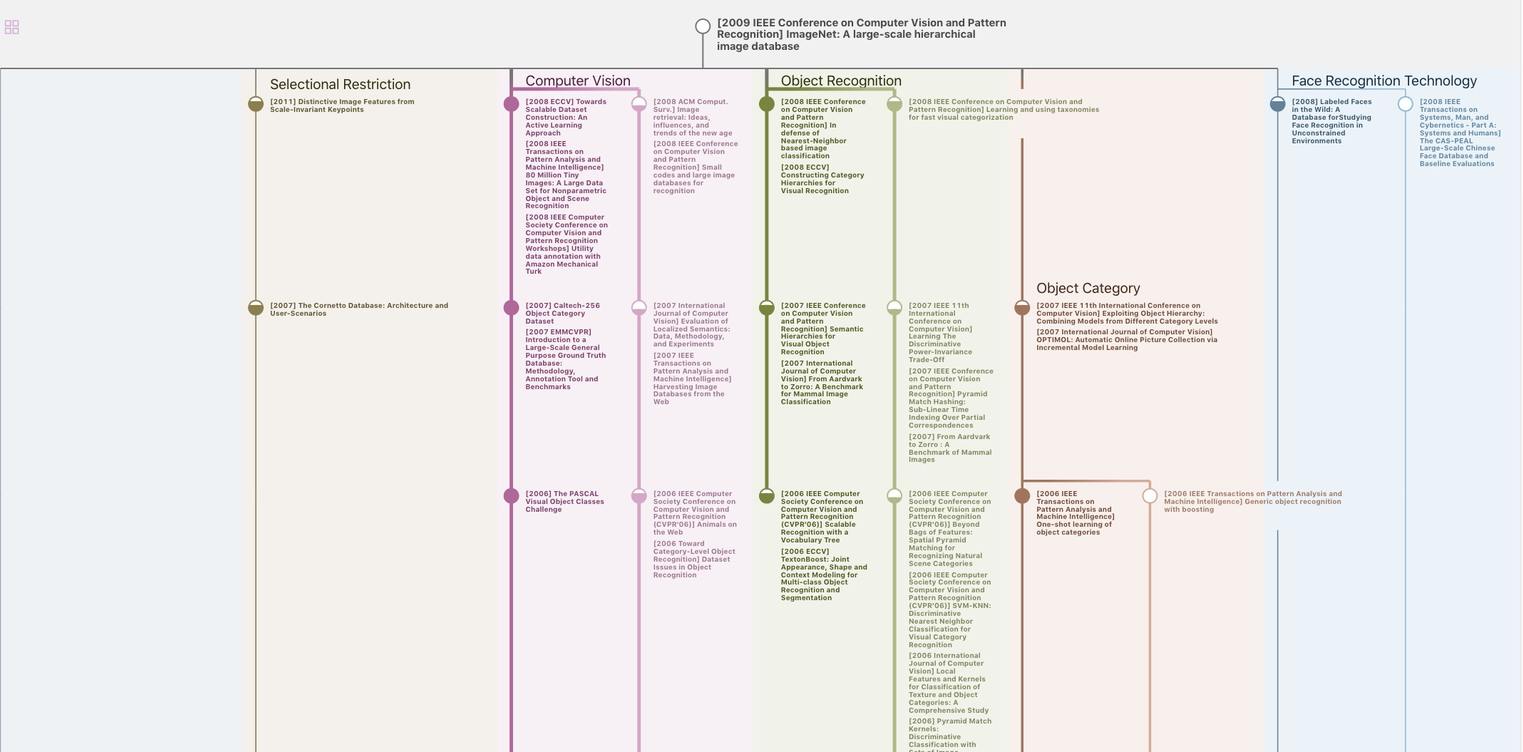
生成溯源树,研究论文发展脉络
Chat Paper
正在生成论文摘要