RIB detection in MR images using shape priors and appearance models
Biomedical Imaging(2014)
摘要
Automatic detection of ribs in magnetic resonance images (MRIs) is extremely challenging due to their poor image appearance. Yet localization of ribs is highly important for effective and safe focused ultrasound therapy. We propose a semi-automatic rib centerline detection method based on combining a rib cage shape model generated from computed tomography (CT) images and a rib appearance model from MRIs. It currently requires the definition of one landmark per rib, and 2 extra landmarks per subject. Using principle component analysis (PCA), 9 components were sufficient to cover 95% of the shape variability. The appearance model was based on 4 region-specific random forest classifiers, which were trained to discriminate between rib and non-rib patches. Their classification performance was 89% on average. The most likely rib location was determined by accepting the rib centerline hypothesis, generated according to the shape model, whose corresponding image patches provided the highest probability of rib appearance. The extracted centerlines had a mean distance of 8.1 mm from the manually selected centerlines. No cross-overs between ribs were observed.
更多查看译文
关键词
biomedical MRI,image classification,object detection,physiological models,principal component analysis,random processes,ultrasonic therapy,MRI,PCA,appearance models,automatic rib detection,classification performance,computed tomography images,distance 8.1 mm,focused ultrasound therapy,image appearance,image patches,landmarks,magnetic resonance images,manually selected centerlines,mean distance,nonrib patches,principle component analysis,region-specific random forest classifiers,rib appearance model,rib cage shape model,rib centerline hypothesis,rib location,semiautomatic rib centerline detection method,shape variability,MR imaging,Rib segmentation,appearance models,random forest classifier,shape models
AI 理解论文
溯源树
样例
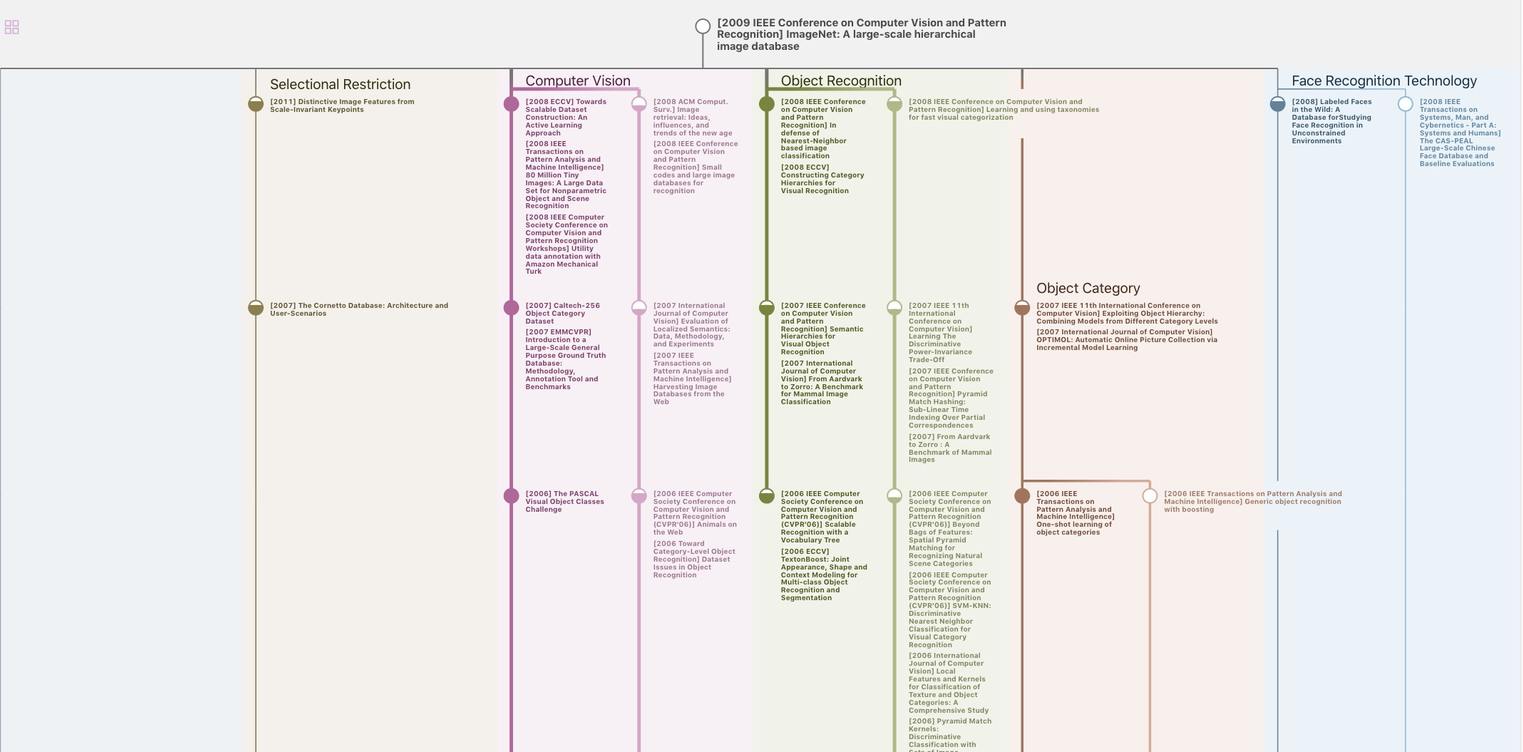
生成溯源树,研究论文发展脉络
Chat Paper
正在生成论文摘要