Epileptiform events detection using a two-stage approach based on multiscale edge detection and artificial neural networks
Neural Engineering(2011)
摘要
An automated detection of epileptiform events can streamline the process of reviewing lengthy EEG records and provide a more quantitative evaluation. This paper introduces a novel two-stage approach of merging human expert knowledge and artificial neural network modeling capabilities. In the first stage, singularities marked through wavelet transform maxima are grouped into epileptiform candidates. These candidates are ranked according to expert mimetic measures that judge candidate morphology, spatial confinement and temporal reproducibility. The second engine is based on ANN. It is trained with the raw EEG data of the topmost candidates to capture high confidence events characteristics. During the evaluation phase, the ANN tests how these characteristics generalize to other candidates. A total of 600 minutes of EEG recordings using all channels were utilized for this study. Data was acquired from 5 patients having different epileptic syndromes. The results showed an average sensitivity of 92% and average selectivity of 96%.
更多查看译文
关键词
diseases,edge detection,electroencephalography,medical signal detection,neural nets,wavelet transforms,ann,eeg,artificial neural network modeling,artificial neural networks,epileptic syndromes,epileptiform events detection,multiscale edge detection,two-stage approach,wavelet transform,artificial neural network,epilepsy,multiscale singularity detection,spike detection,sensitivity,indexing terms,engines
AI 理解论文
溯源树
样例
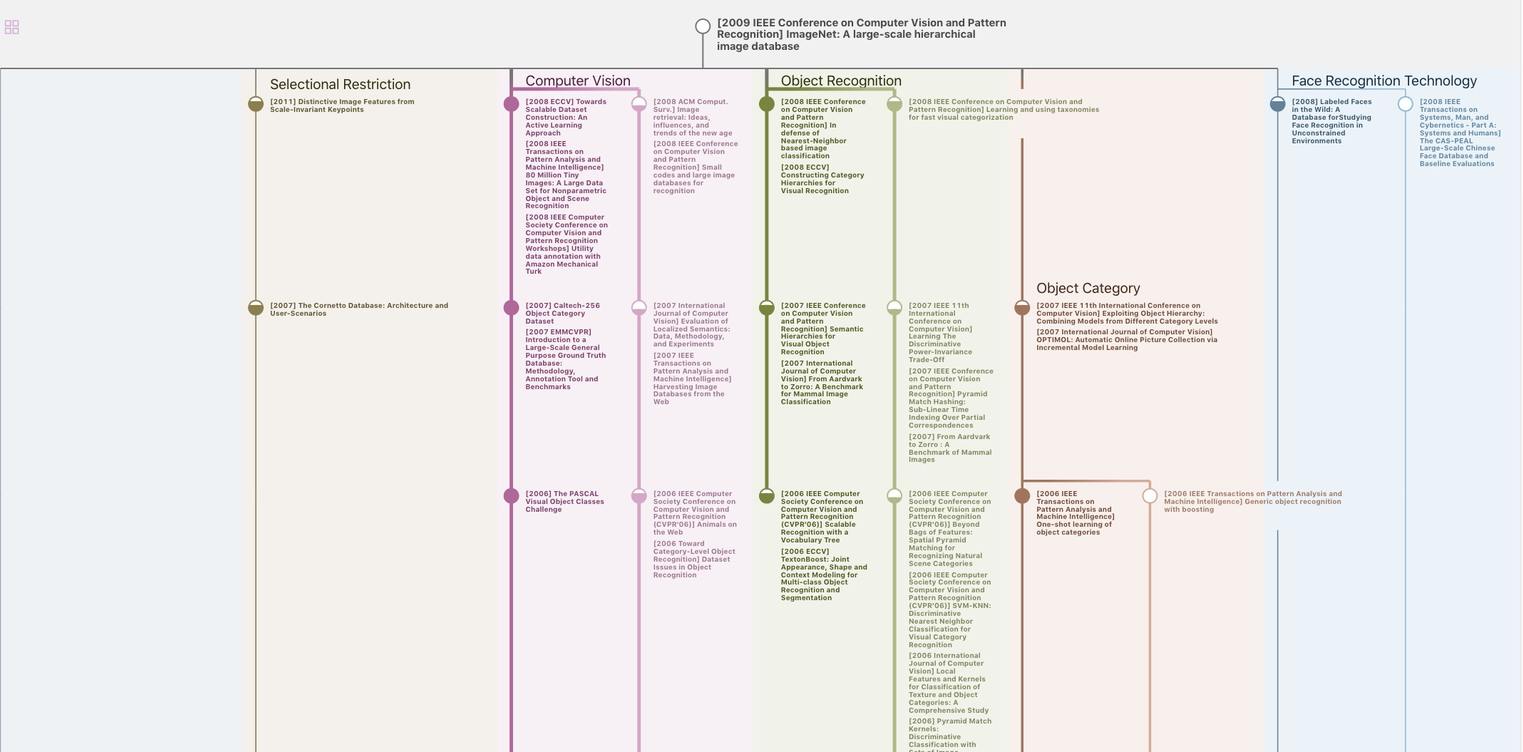
生成溯源树,研究论文发展脉络
Chat Paper
正在生成论文摘要