Identification of Functionally Related Enzymes by Learning-to-Rank Methods
Computational Biology and Bioinformatics, IEEE/ACM Transactions(2014)
摘要
Enzyme sequences and structures are routinely used in the biological sciences as queries to search for functionally related enzymes in online databases. To this end, one usually departs from some notion of similarity, comparing two enzymes by looking for correspondences in their sequences, structures or surfaces. For a given query, the search operation results in a ranking of the enzymes in the database, from very similar to dissimilar enzymes, while information about the biological function of annotated database enzymes is ignored. In this work, we show that rankings of that kind can be substantially improved by applying kernel-based learning algorithms. This approach enables the detection of statistical dependencies between similarities of the active cleft and the biological function of annotated enzymes. This is in contrast to search-based approaches, which do not take annotated training data into account. Similarity measures based on the active cleft are known to outperform sequence-based or structure-based measures under certain conditions. We consider the Enzyme Commission (EC) classification hierarchy for obtaining annotated enzymes during the training phase. The results of a set of sizeable experiments indicate a consistent and significant improvement for a set of similarity measures that exploit information about small cavities in the surface of enzymes.
更多查看译文
关键词
bioinformatics,enzymes,learning (artificial intelligence),molecular biophysics,molecular configurations,pattern classification,statistical analysis,annotated database enzymes,biological function,biological sciences,enzyme commission classification hierarchy,enzyme sequences,enzyme structures,enzyme surface,functionally related enzymes,functionally related enzymes identification,kernel-based learning algorithms,learning-to-rank methods,online databases,search operation,sequence-based measures,statistical dependencies,structure-based measures,Bioinformatics,biochemistry,machine learning,proteins
AI 理解论文
溯源树
样例
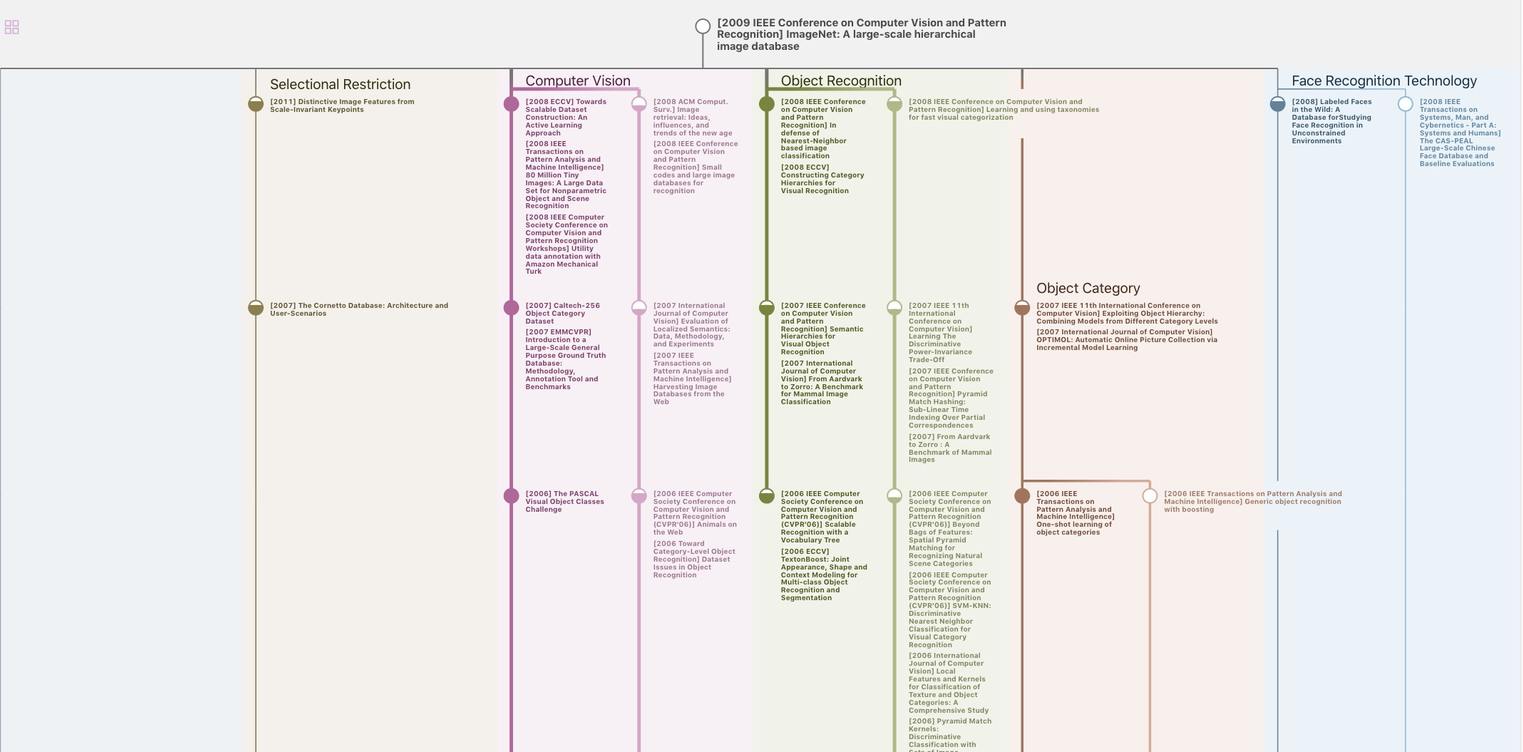
生成溯源树,研究论文发展脉络
Chat Paper
正在生成论文摘要