Leave-One-Out Bounds for Support Vector Regression Model Selection
Neural Computation(2005)
摘要
Minimizing bounds of leave-one-out errors is an important and efficient approach for support vector machine (SVM) model selection. Past research focuses on their use for classification but not regression. In this letter, we derive various leave-one-out bounds for support vector regression (SVR) and discuss the difference from those for classification. Experiments demonstrate that the proposed bounds are competitive with Bayesian SVR for parameter selection. We also discuss the differentiability of leave-one-out bounds.
更多查看译文
关键词
support vector regression,support vector machine,model selection
AI 理解论文
溯源树
样例
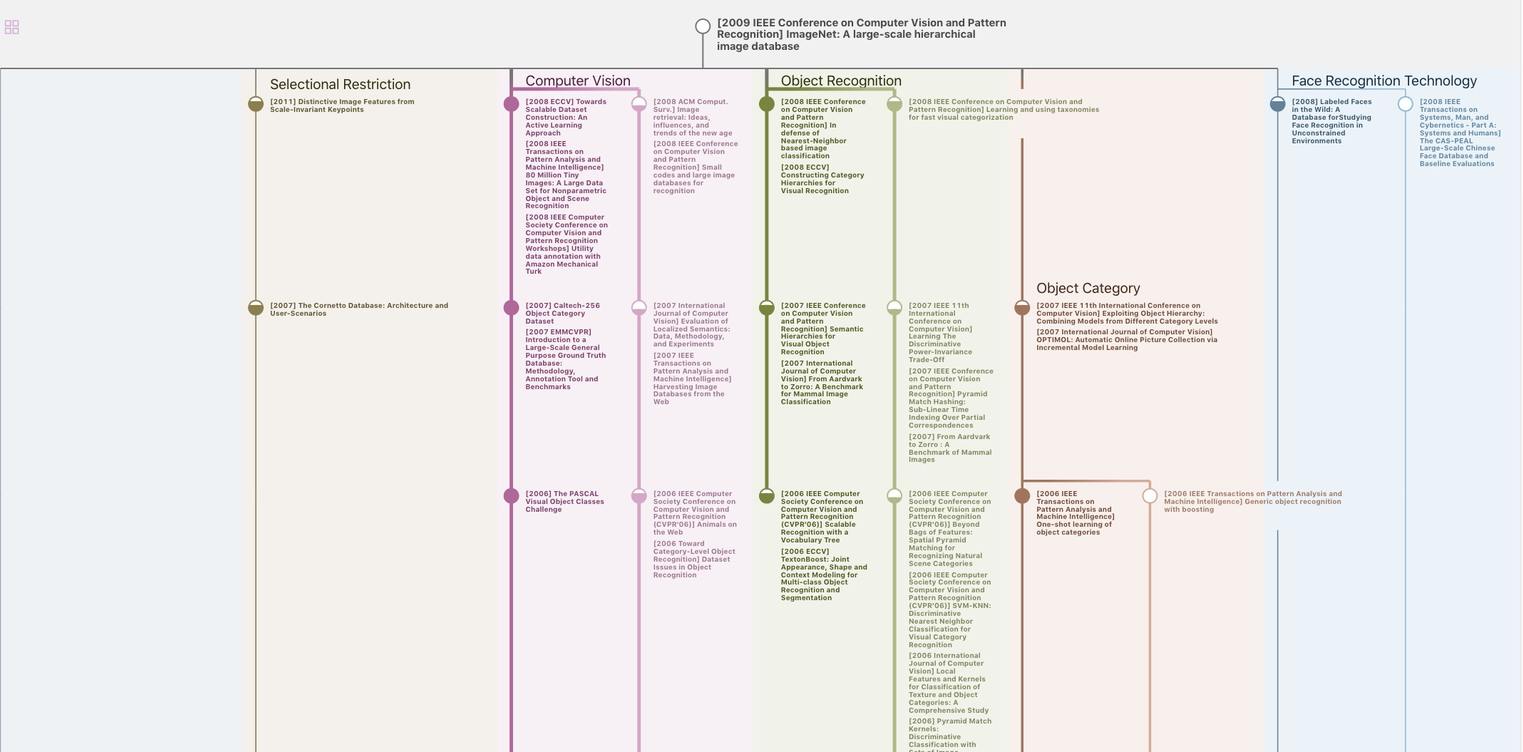
生成溯源树,研究论文发展脉络
Chat Paper
正在生成论文摘要