NDOD: An efficient neighboring dependent outlier detector for bias distributed large datasets
Information Science and Technology(2011)
摘要
Outlier detection is an important problem for many domains, including fraud detection, network intrusion and medical diagnosis. Discovery of unexpected knowledge revealed from outliers is becoming an integral aspect of data mining. Existing works in this field fall short of the adaptability to the distributive feature of the dataset. This paper presents a novel approach for outlier detection with high efficiency and the ability to closely monitor the neighboring density characteristics around outliers. A generalized neighboring dependent outlier is defined, followed by a cell-based detection algorithm. Results of extensive experimental studies on real-world and synthetic datasets demonstrate the effectiveness of the algorithm with respect to the size, the bias distributive structure of the datasets.
更多查看译文
关键词
data mining,bias distributed large datasets,cell based detection algorithm,fraud detection,medical diagnosis,neighboring dependent outlier detector,network intrusion,unexpected knowledge discovery,least squares approximation,clustering algorithms,algorithm design and analysis,algorithm design
AI 理解论文
溯源树
样例
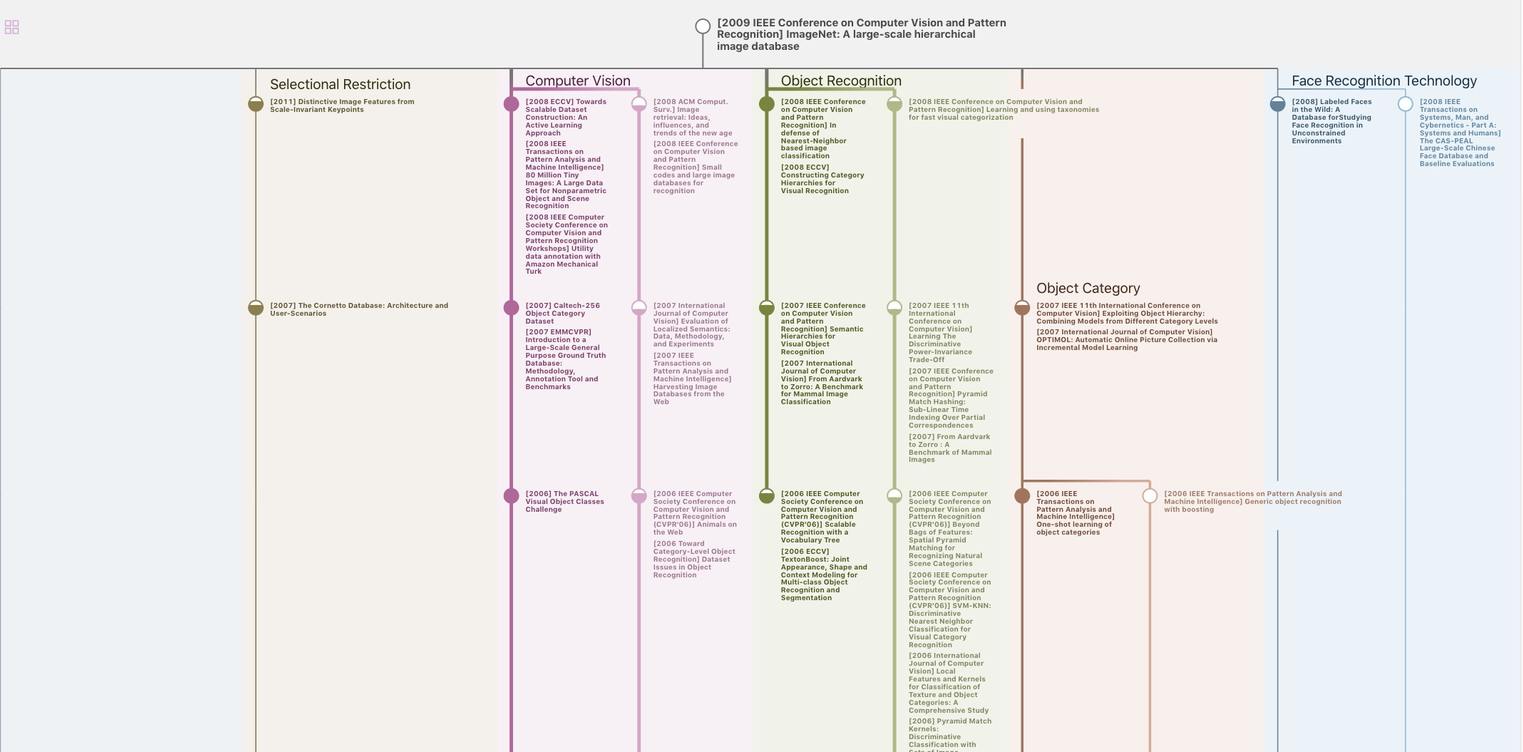
生成溯源树,研究论文发展脉络
Chat Paper
正在生成论文摘要