Single image super-resolution using adaptive domain transformation
Image Processing(2013)
摘要
In this paper we propose a new image domain prior term for regularizing the super-resolution reconstruction algorithm. This term encourages preserving the local ramp structure around edges, in the reconstruction algorithm. Ramp at a pixel is defined as the steepest sequence of monotonically increasing (or decreasing) pixels among all feasible directions around the pixel. As described in previous work, ramp based modeling is a richer characterization of local image structure than conventional gradients. Our proposed ramp-preserving constraint image is obtained by first running an accurate segmentation algorithm (which is itself obtained by ramp based modeling) on the low resolution image. We then perform a domain transformation of the pixels belonging to the steepest ramps at the edge pixels, in order to preserve sharpness. The resulting non-uniformly spaced image is then upscaled to a uniform, high resolution grid, using an edge preserving non-uniform interpolation scheme. This image is then used both as the prior constraint as well as the initial guess for the iterative super-resolution reconstruction algorithm. Our results compare favorably to the classical back-projection algorithm as well as newer methods which use learning based gradient domain priors.
更多查看译文
关键词
edge detection,gradient methods,image resolution,image segmentation,image sequences,interpolation,learning (artificial intelligence),transforms,adaptive domain transformation,edge preserving nonuniform interpolation scheme,iterative super-resolution reconstruction algorithm,learning based gradient domain priors,local ramp structure preservation,ramp-preserving constraint image,segmentation algorithm,sharpness preservation,single image super-resolution algorithm,steepest pixel sequence,Super-resolution,image prior,regularization,segmentation
AI 理解论文
溯源树
样例
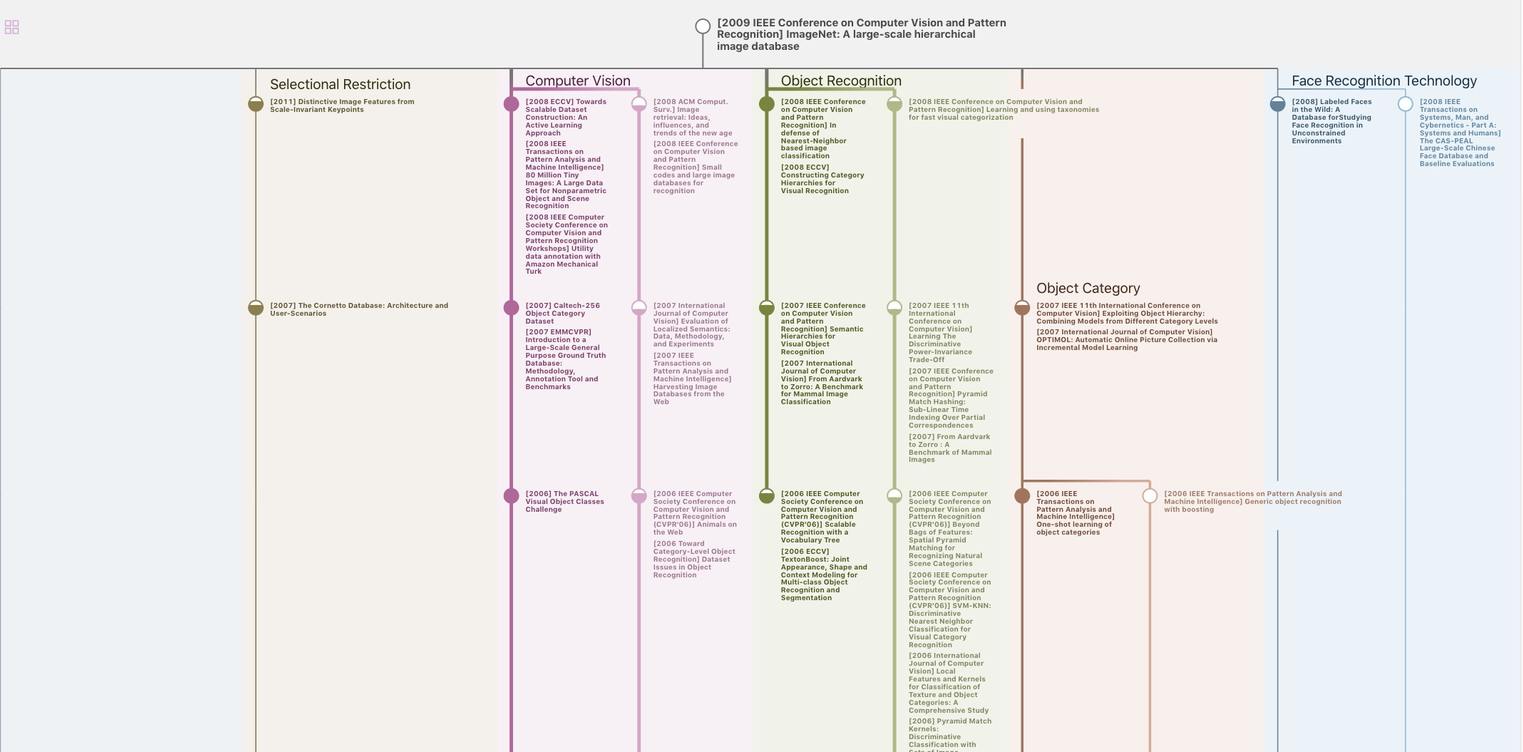
生成溯源树,研究论文发展脉络
Chat Paper
正在生成论文摘要