Building high-level features using large scale unsupervised learning
Acoustics, Speech and Signal Processing(2012)
摘要
We consider the problem of building high-level, class-specific feature detectors from only unlabeled data. For example, is it possible to learn a face detector using only unlabeled images? To answer this, we train a deep sparse autoencoder on a large dataset of images (the model has 1 billion connections, the dataset has 10 million 200×200 pixel images downloaded from the Internet). We train this network using model parallelism and asynchronous SGD on a cluster with 1,000 machines (16,000 cores) for three days. Contrary to what appears to be a widely-held intuition, our experimental results reveal that it is possible to train a face detector without having to label images as containing a face or not. Control experiments show that this feature detector is robust not only to translation but also to scaling and out-of-plane rotation. We also find that the same network is sensitive to other high-level concepts such as cat faces and human bodies. Starting from these learned features, we trained our network to recognize 22,000 object categories from ImageNet and achieve a leap of 70% relative improvement over the previous state-of-the-art.
更多查看译文
关键词
face recognition,image coding,object detection,unsupervised learning,imagenet,asynchronous sgd,cat faces,deep sparse autoencoder,face detector,high-level class-specific feature detectors,human bodies,model parallelism,picture size 200 pixel
AI 理解论文
溯源树
样例
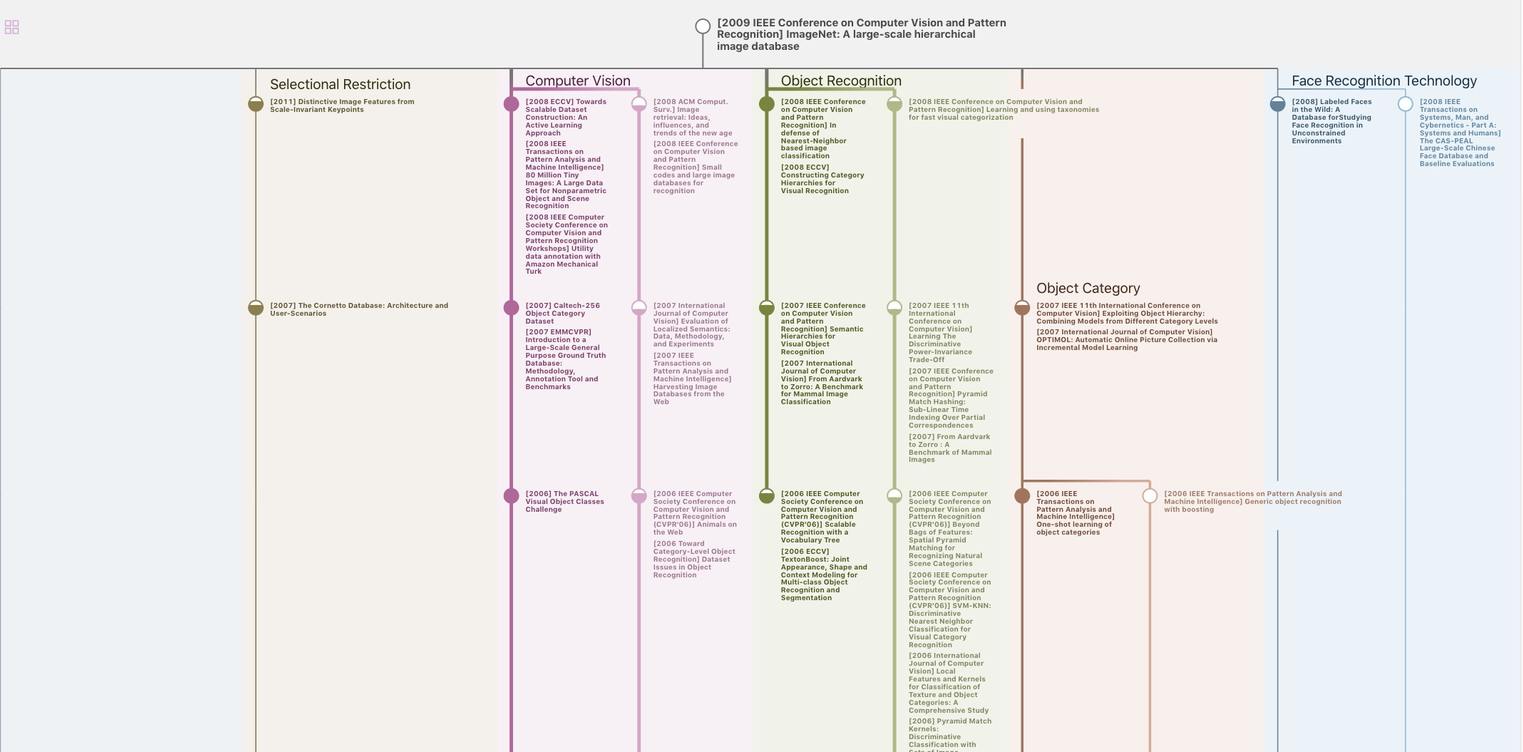
生成溯源树,研究论文发展脉络
Chat Paper
正在生成论文摘要