Method for enhancing low quality depth maps for 3D reconstruction on a embedded platform
Acoustics, Speech and Signal Processing(2013)
摘要
In the recent past, vast amounts of stereo and augmented reality based applications are being developed for hand-held devices. In most of these applications depth map is a key ingredient for acceptable user experience. Accuracy and high density of depth map are important along with meeting real-time constraints on an embedded system. There is an inherent tradeoff between depth map quality and speed and invariably performance is usually important for competing in todays high-definition video marketplace. In this paper we present a method that addresses depth map quality while still maintaining performance at video frame-rates. Specifically, we discuss a technique to enhance a low-quality depth map for 3D point cloud generation on an embedded platform. We provide performance metrics and estimates on a Texas Instruments (TI) OMAP embedded platform and show that using simple pre and post-processing techniques one can achieve both quality and performance. A preliminary version of our point cloud application developed has a frame rate of about 15fps, majority being display and rendering related overheads. The core algorithms including pre and post processing have a much higher frame rate of about 23-25fps. We estimate that with adequate mapping of the algorithms to various cores and accelerated kernels, the frame rate could reach real-time performance of 30fps.
更多查看译文
关键词
Texas Instruments computers,augmented reality,embedded systems,high definition video,image reconstruction,rendering (computer graphics),stereo image processing,3D point cloud generation,3D reconstruction,OMAP embedded platform,Texas instrument,augmented reality,depth map quality enhancement,embedded system,handheld device,high definition video marketplace,performance metrics,rendering,stereo reality,video frame rate,3D point cloud,Embedded Platform,TI OMAP,color/contrast match,depth quality,multi-core
AI 理解论文
溯源树
样例
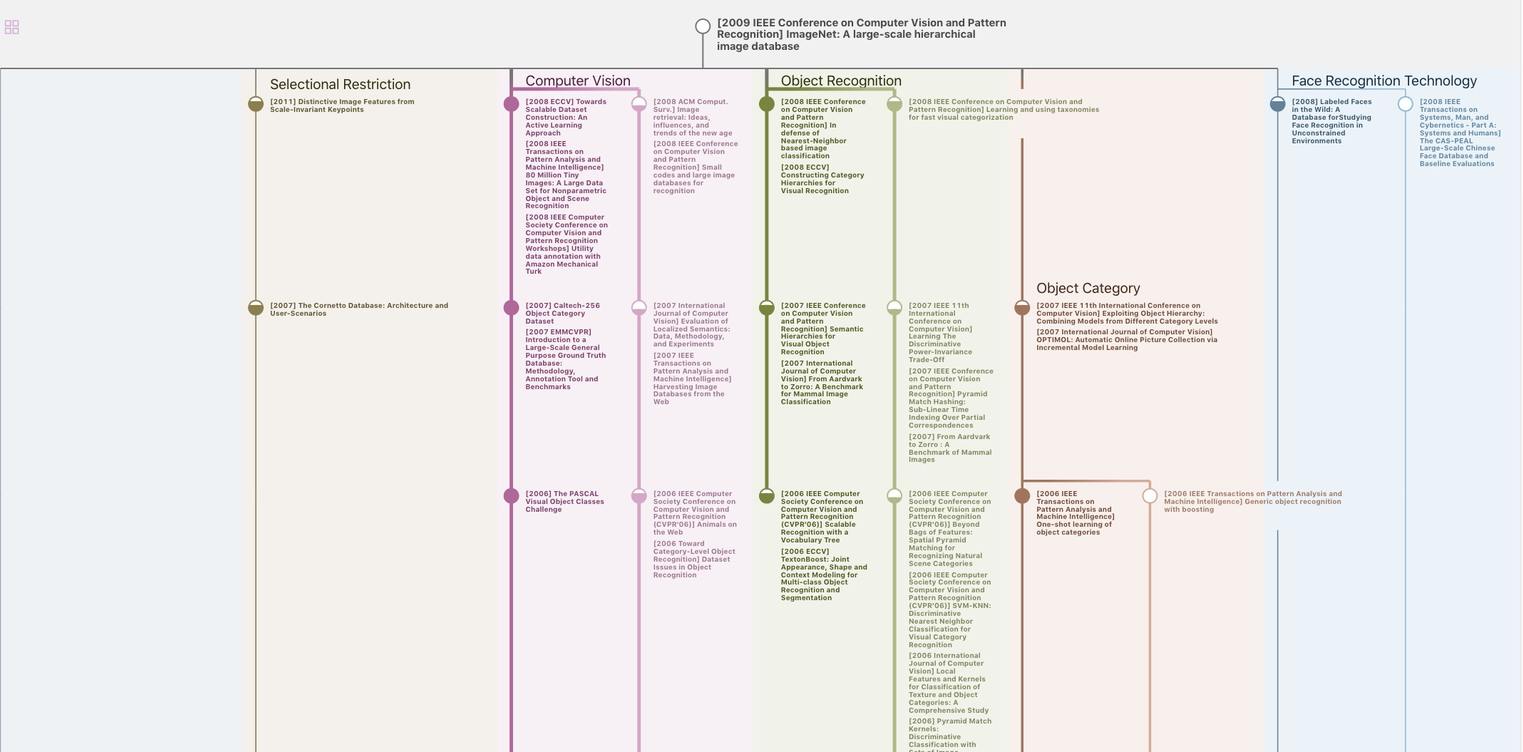
生成溯源树,研究论文发展脉络
Chat Paper
正在生成论文摘要