Scalable machine learning framework for behavior-based access control
Resilient Control Systems(2013)
摘要
Today's activities in cyber space are more connected than ever before, driven by the ability to dynamically interact and share information with a changing set of partners over a wide variety of networks. The success of approaches aimed at securing the infrastructure has changed the threat profile to point where the biggest threat to the US cyber infrastructure is posed by targeted cyber attacks. The Behavior-Based Access Control (BBAC) effort has been investigating means to increase resilience against these attacks. Using statistical machine learning, BBAC (a) analyzes behaviors of insiders pursuing targeted attacks and (b) assesses trustworthiness of information to support real-time decision making about information sharing. The scope of this paper is to describe the challenge of processing disparate cyber security information at scale, together with an architecture and work-in-progress prototype implementation for a cloud framework supporting a strategic combination of stream and batch processing.
更多查看译文
关键词
authorisation,learning (artificial intelligence),statistical analysis,bbac,us cyber infrastructure,batch processing,behavior based access control,cloud framework,cyber attacks,cyber security information,cyber space,information sharing,real-time decision making,scalable machine learning framework,statistical machine learning,stream processing,access control,cloud computing,machine learning,security reasoning,trust management,learning artificial intelligence,computer network security,behavior,algorithms,classification
AI 理解论文
溯源树
样例
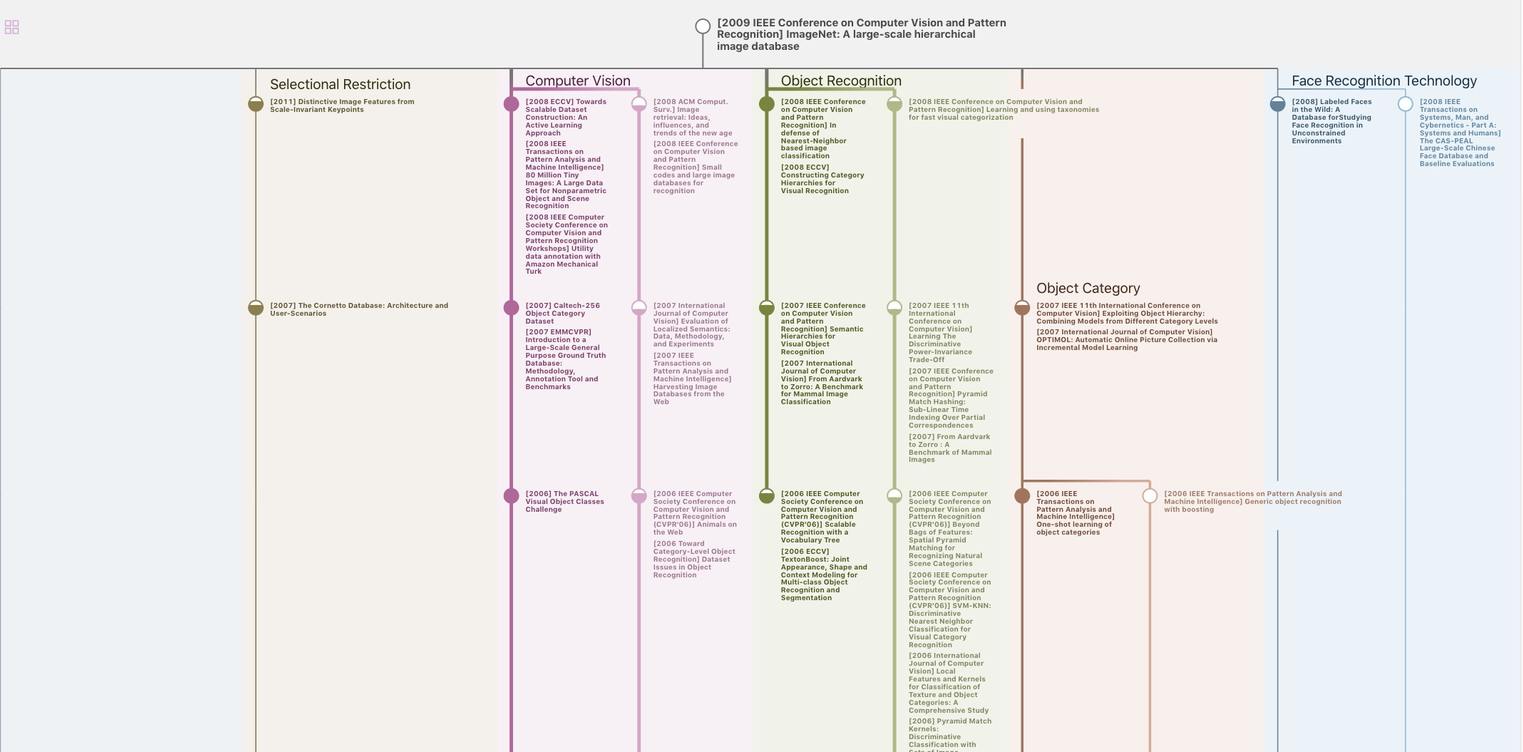
生成溯源树,研究论文发展脉络
Chat Paper
正在生成论文摘要