A new method of concurrently visualizing states, values, and actions in reinforcement based brain machine interfaces.
EMBC(2013)
摘要
This paper presents the first attempt to quantify the individual performance of the subject and of the computer agent on a closed loop Reinforcement Learning Brain Machine Interface (RLBMI). The distinctive feature of the RLBMI architecture is the co-adaptation of two systems (a BMI decoder in agent and a BMI user in environment). In this work, an agent implemented using Q-learning via kernel temporal difference (KTD)(λ) decodes the neural states of a monkey and transforms them into action directions of a robotic arm. We analyze how each participant influences the overall performance both in successful and missed trials by visualizing states, corresponding action value Q, and resulting actions in two-dimensional space. With the proposed methodology, we can observe how the decoder effectively learns a good state to action mapping, and how neural states affect the prediction performance.
更多查看译文
关键词
reinforcement based brain machine interfaces,biocontrol,q-learning,learning (artificial intelligence),brain-computer interfaces,bmi decoder,monkey,robotic arm,kernel temporal difference,neural states,concurrent visualization,closed loop rlbmi,closed loop reinforcement learning brain machine interface,robots,vectors,decoding,learning artificial intelligence,brain computer interfaces,visualization,principal component analysis,kernel,q learning
AI 理解论文
溯源树
样例
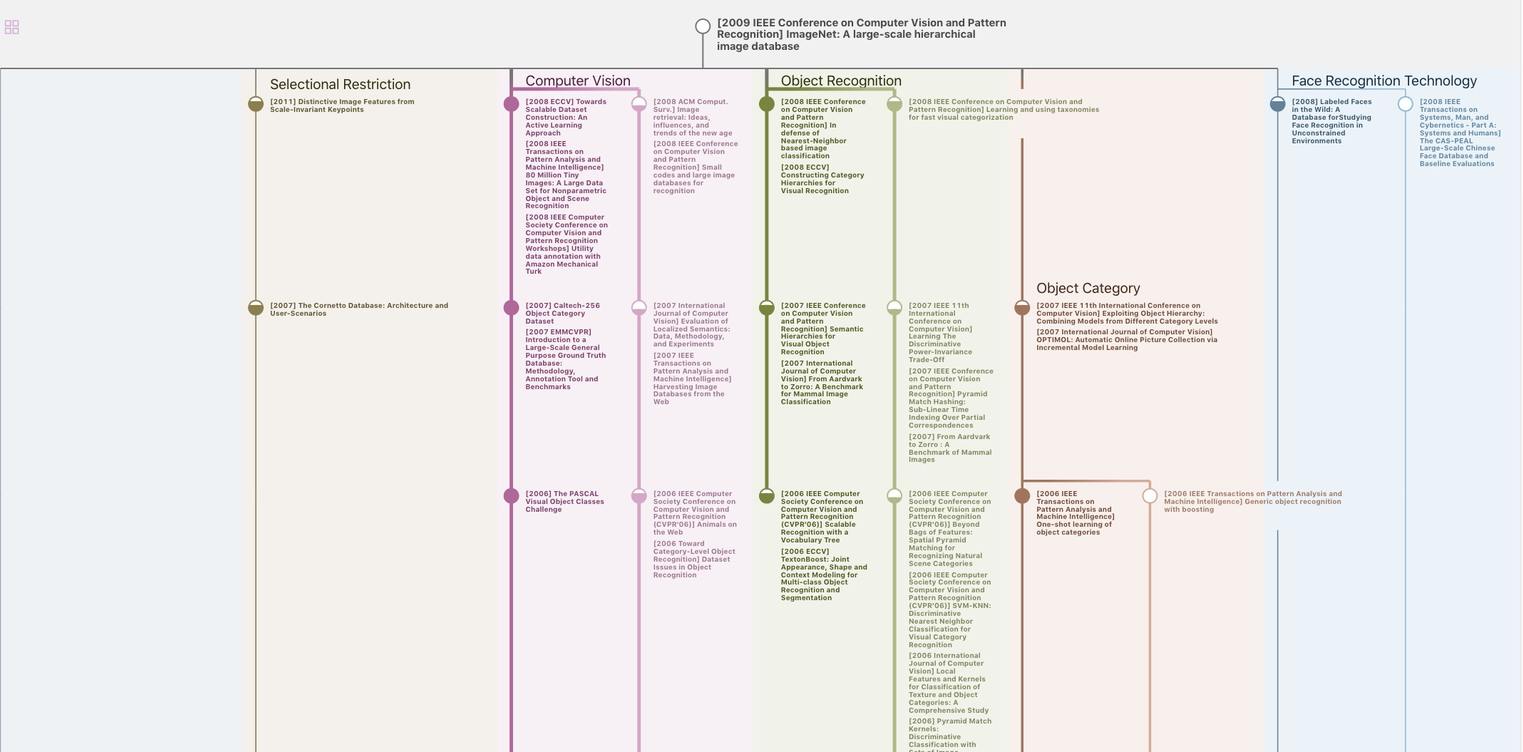
生成溯源树,研究论文发展脉络
Chat Paper
正在生成论文摘要