Anomaly detection in time series data using a fuzzy c-means clustering
IFSA World Congress and NAFIPS Annual Meeting(2013)
摘要
Detecting incident anomalies within temporal data - time series becomes useful in a variety of applications. In this paper, anomalies in time series are divided into two categories, namely amplitude anomalies and shape anomalies. A unified framework supporting the detection of both types of anomalies is introduced. A fuzzy clustering is employed to reveal the available structure within time series and a reconstruction criterion is used to assign an anomaly score to each subsequence. In the case of detecting anomalies in amplitude, the original representation of time series is used, while for detecting anomalies in shape an autocorrelation representation of time series to capture shape information is employed. Experimental studies concerning two real-world data sets are reported.
更多查看译文
关键词
data mining,data structures,fuzzy set theory,pattern clustering,time series,amplitude anomalies,autocorrelation representation,fuzzy C-means clustering,incident anomaly detection,real-world data sets,shape anomalies,shape information,temporal data,time series data,time series representation
AI 理解论文
溯源树
样例
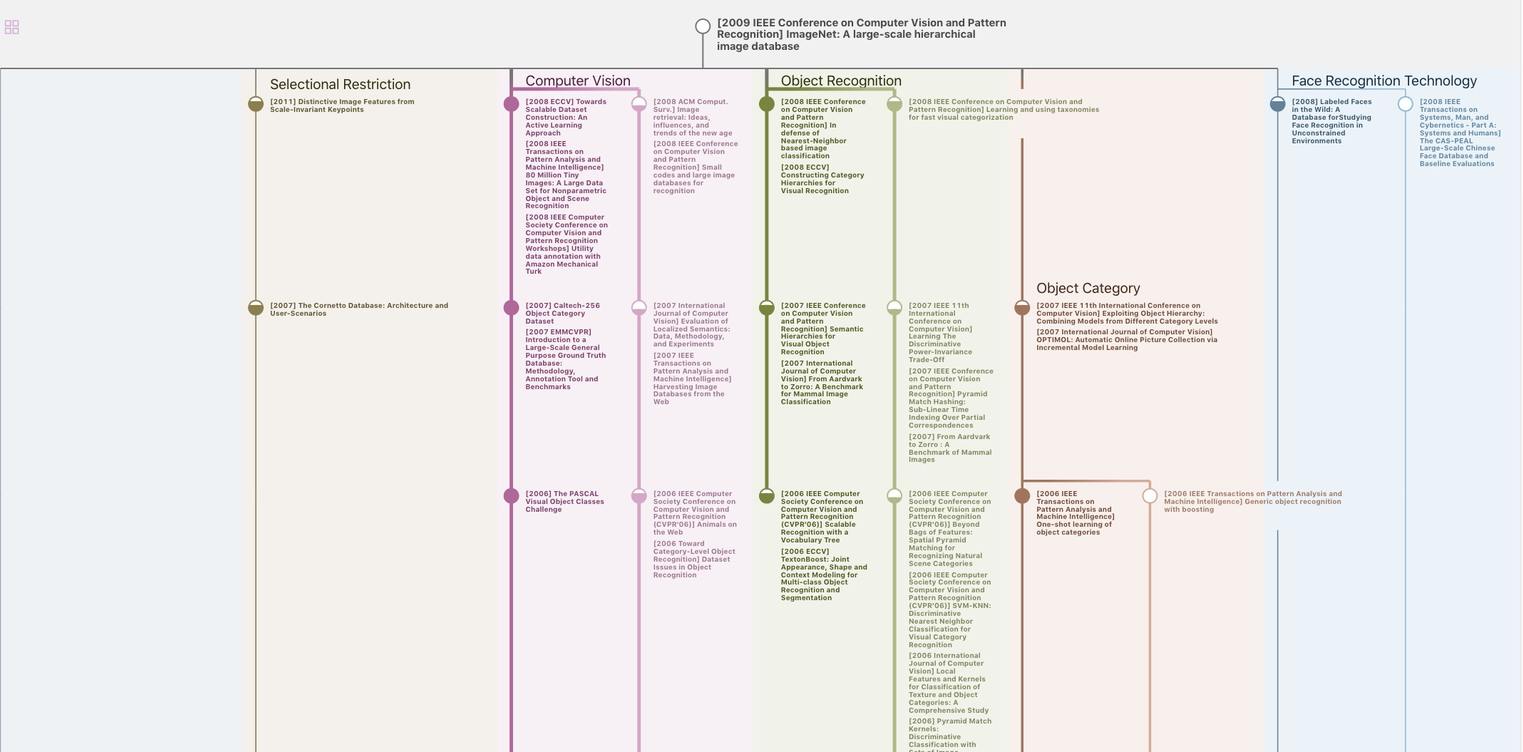
生成溯源树,研究论文发展脉络
Chat Paper
正在生成论文摘要