Distribution-sensitive learning for imbalanced datasets
Automatic Face and Gesture Recognition(2013)
摘要
Many real-world face and gesture datasets are by nature imbalanced across classes. Conventional statistical learning models (e.g., SVM, HMM, CRY), however, are sensitive to imbalanced datasets. In this paper we show how an imbalanced dataset affects the performance of a standard learning algorithm, and propose a distribution-sensitive prior to deal with the imbalanced data problem. This prior analyzes the training dataset before learning a model, and puts more weight on the samples from underrepresented classes, allowing all samples in the dataset to have a balanced impact in the learning process. We report on two empirical studies regarding learning with imbalanced data, using two publicly available recent gesture datasets, the Microsoft Research Cambridge-12 (MSRC-12) and NATOPS aircraft handling signals datasets. Experimental results show that learning from balanced data is important, and that the distribution-sensitive prior improves performance with imbalanced datasets.
更多查看译文
关键词
data analysis,hidden markov models,learning (artificial intelligence),support vector machines,cry,hmm,msrc-12,microsoft research cambridge-12,natops aircraft handling signals datasets,svm,distribution-sensitive learning,face datasets,gesture datasets,imbalanced datasets,nature imbalanced across classes,standard learning algorithm,statistical learning models,learning artificial intelligence
AI 理解论文
溯源树
样例
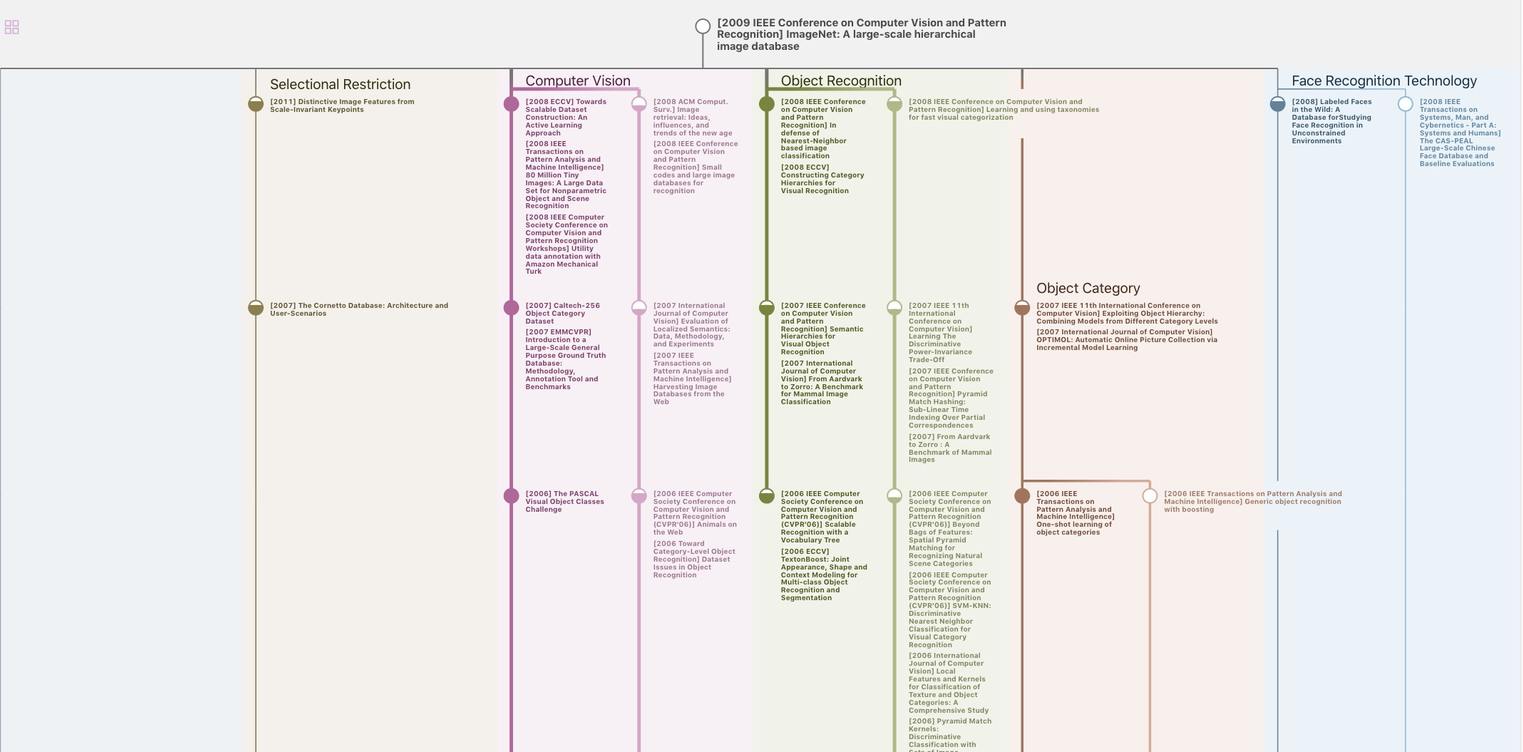
生成溯源树,研究论文发展脉络
Chat Paper
正在生成论文摘要