RBPF-SLAM based on probabilistic geometric planar constraints
IEEE Conf. of Intelligent Systems(2010)
摘要
Recent advances in visual SLAM have focused on improving estimation of sparse 3D points or patches that represent parts of surroundings. In order to establish an adequate scene understanding, inference of spatial relations among landmarks must be part of the SLAM processing. A novel Rao-Blackwilized PF-SLAM algorithm is proposed to utilize geometric relations of landmarks with respect to high level features, such as planes, for improving estimation. These geometric relations are defined as a set of geometric constraint hypotheses inferred during the mapping task. In each prediction-update cycle of estimation, probabilistic constraints are created and applied to update landmarks based on a hierarchical inference process. Based on experiments, improvement over estimation and completeness of the scene description is achieved using the proposal of this paper.
更多查看译文
关键词
SLAM (robots),particle filtering (numerical methods),robot vision,sparse matrices,spatial reasoning,RBPF-SLAM,Rao-Blackwilized particle filter,geometric constraint hypotheses,hierarchical inference process,prediction update cycle,probabilistic geometric planar constraint,simultaneous localization and mapping,sparse 3D point
AI 理解论文
溯源树
样例
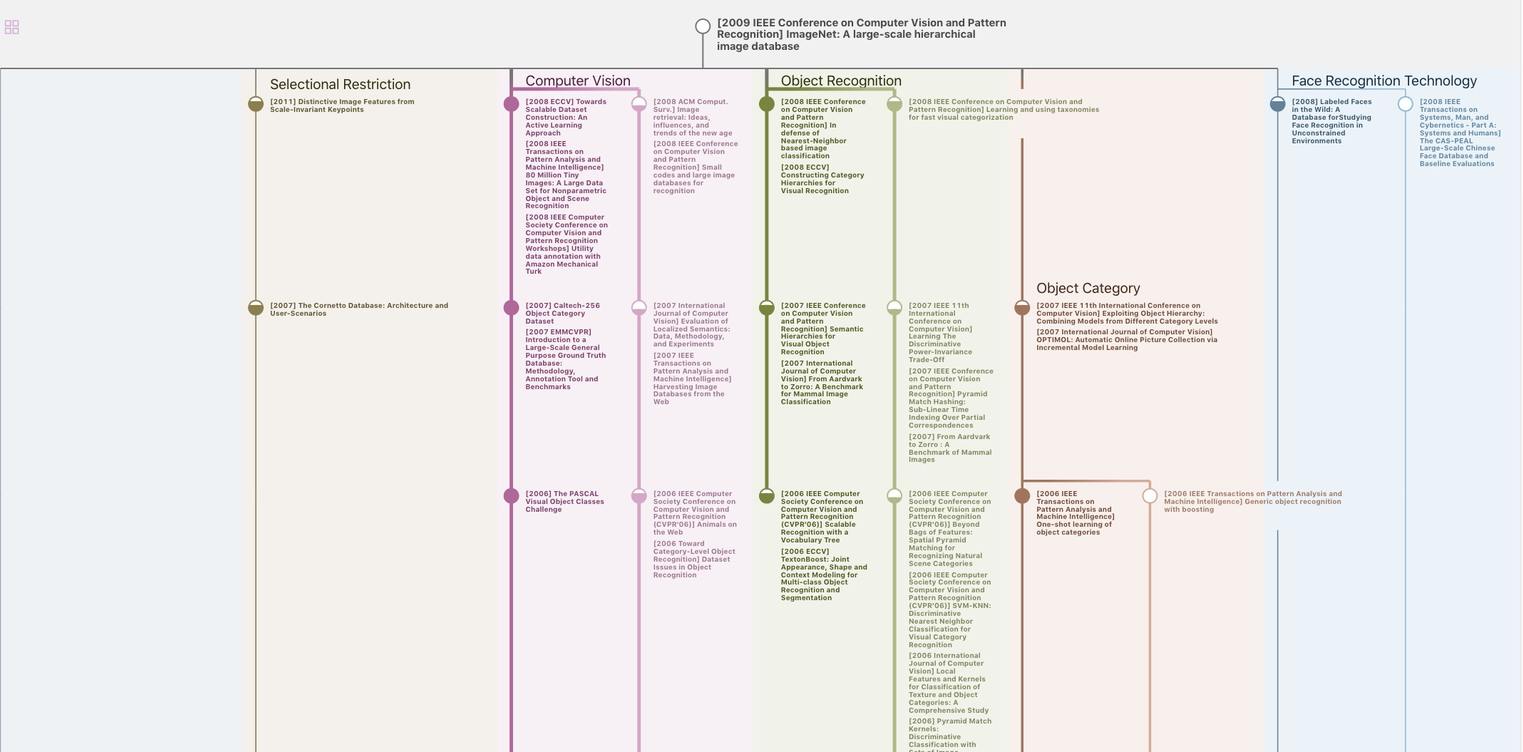
生成溯源树,研究论文发展脉络
Chat Paper
正在生成论文摘要