Random Distortion Testing and Optimality of Thresholding Tests
IEEE Transactions on Signal Processing(2013)
摘要
This paper addresses the problem of testing whether the Mahalanobis distance between a random signal $ \\Theta $ and a known deterministic model $ \\theta _{0}$ exceeds some given non-negative real number or not, when $ \\Theta $ has unknown probability distribution and is observed in additive independent Gaussian noise with positive definite covariance matrix. When $ \\Theta $ is deterministic unknown, we prove the existence of thresholding tests on the Mahalanobis distance to $ \\theta _{0}$ that have specified level and maximal constant power (MCP). The MCP property is a new optimality criterion involving Wald's notion of tests with uniformly best constant power ( UBCP) on ellipsoids for testing the mean of a normal distribution. When the signal is random with unknown distribution, constant power maximality extends to maximal constant conditional power (MCCP) and the thresholding tests on the Mahalanobis distance to $ \\theta _{0}$ still verify this novel optimality property. Our results apply to the detection of signals in independent and additive Gaussian noise. In particular, for a large class of possible model mismatches, MCCP tests can guarantee a specified false alarm probability, in contrast to standard Neyman-Pearson tests that may not respect this constraint.
更多查看译文
关键词
Gaussian noise,covariance matrices,distortion,normal distribution,probability,signal detection,MCP property,Mahalanobis distance,additive Gaussian noise,additive independent Gaussian noise,constant power maximality,covariance matrix,deterministic model,false alarm probability,maximal constant conditional power,maximal constant power,nonnegative real,probability distribution,random distortion testing,random signal,signal detection,standard Neyman-Pearson tests,thresholding tests optimality,uniformly best constant power,unknown distribution,Event testing,Mahalanobis norm,hypothesis testing,invariance,random distortion testing,test with maximal constant conditional power,test with maximal constant power,test with uniformly best constant power
AI 理解论文
溯源树
样例
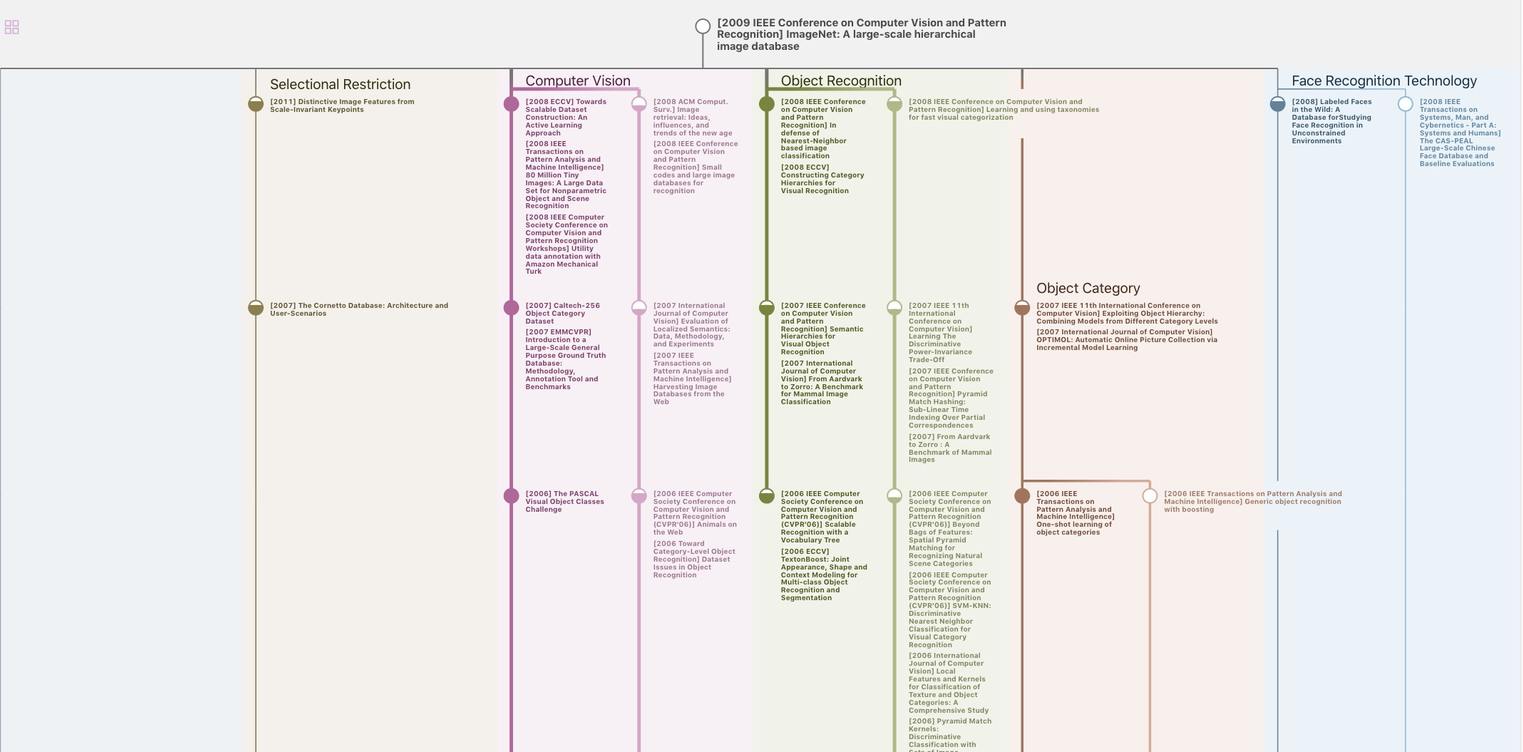
生成溯源树,研究论文发展脉络
Chat Paper
正在生成论文摘要