Parameter-free subsequences time series clustering with various-width clusters
Knowledge and Smart Technology(2013)
摘要
In time series mining, one of the interesting tasks that attract many researchers is time series clustering which is classified into two main categories. Whole time series clustering considers how to cluster multiple time series, and the other one is Subsequence Time Series (STS) clustering, a clustering of subparts or subsequences within a single time series. Deplorably, STS clustering is not preferable even though it had widely been used as a subroutine in various mining tasks, e.g., rule discovery, anomaly detection, or classification, due to the recent finding a decade ago that STS clustering problem can produce meaningless results. There have been numerous attempts to resolve this problem but seemed to be unsuccessful. Until the two most recent attempts, they seem to accomplish in producing meaningful results; however, their approaches do need some predefined constraint values, such as the width of the subsequences that are in fact quite subjective and sensitive. Thus, we propose a novel parameter-free clustering technique to eliminate this problem by utilizing a motif discovery algorithm and some statistical principles to properly determine these parameters. Our experimental results from well-known datasets demonstrate the effectiveness of the proposed algorithm in selecting the proper subsequence width, and in turn leading to meaningful and highly accurate results.
更多查看译文
关键词
Parameter-Free,STS Clustering,Time Series,Various Length,
AI 理解论文
溯源树
样例
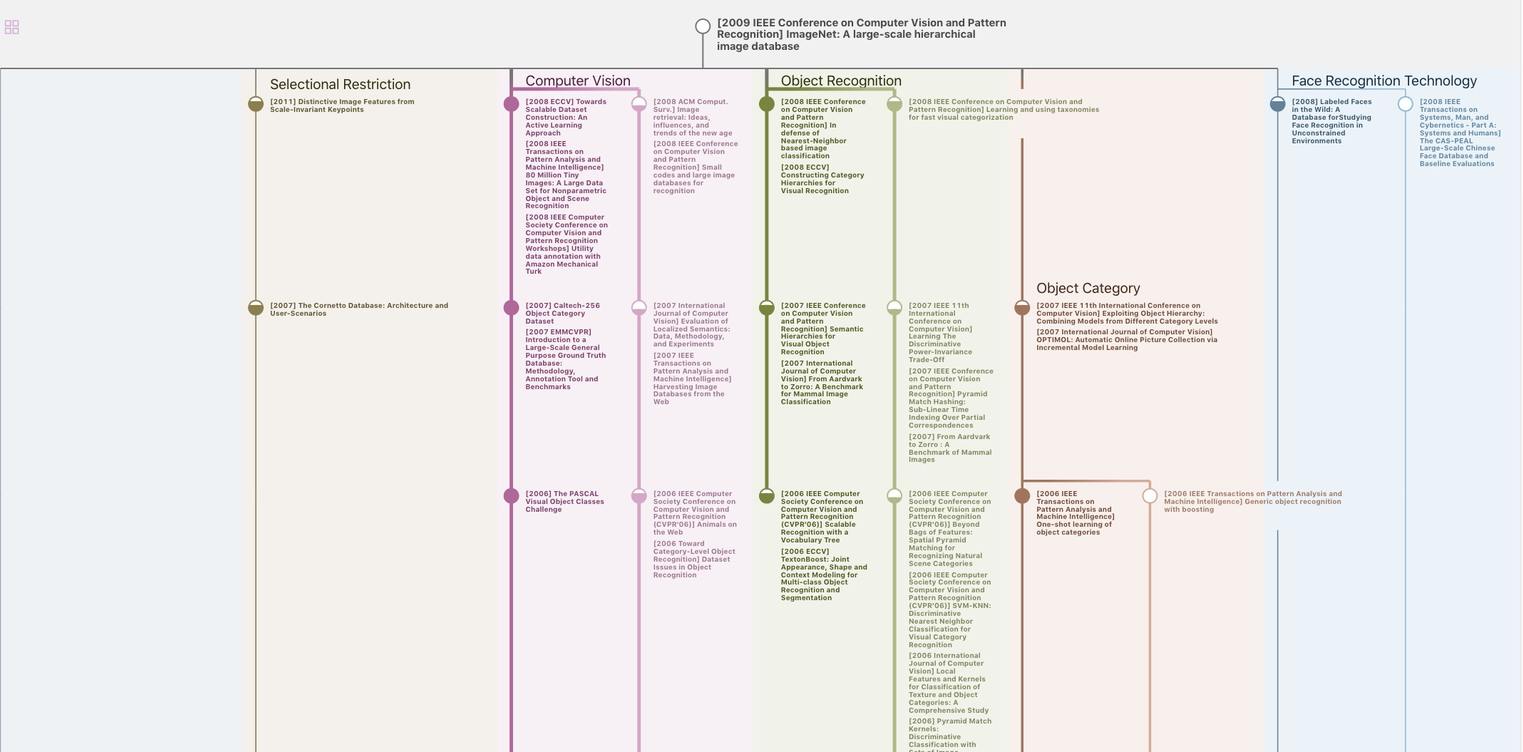
生成溯源树,研究论文发展脉络
Chat Paper
正在生成论文摘要