An Information-Theoretic Combining Method for Multi-Classifier Anomaly Detection Systems
Communications(2010)
摘要
Recent studies have shown that standalone anomaly classifiers used by network anomaly detectors are unable to provide acceptable accuracies in real-world deployments. To achieve higher accuracies, Network Anomaly Detection Systems (NADSs) now use multiple classifiers whose outputs are combined to formulate an aggregate anomaly score. Judicious methods of combining these classifiers' outputs are largely unexplored. In this paper, we propose a novel information-theoretic combining method which caters for the individual classifiers' accuracies in a multi-classifier NADS. We first show that existing combining schemes designed for or adapted to the problem of multi-classifier NADS combining do not provide good accuracies because they do not use individual classifiers' detection and false alarm rates in the combining process. Furthermore, we reveal that an accurate multi-classifier NADS, in addition to catering for the mean accuracy rates, must also consider the classifiers' variances during combining. Therefore, we propose a Standard Deviation normalized Entropy of Accuracy (SDnEA) method for classifier combining. Using 9 prominent classifiers operating on two publicly-available traffic datasets, we show that around 3%-10% increase in detection rate and a 40% decrease in false alarm rate over existing combining techniques can be provided by the proposed information-theoretic NADS combining technique.
更多查看译文
关键词
information theory,computer networks,intrusion detection,logic,entropy,detectors,anomaly detection,computer science
AI 理解论文
溯源树
样例
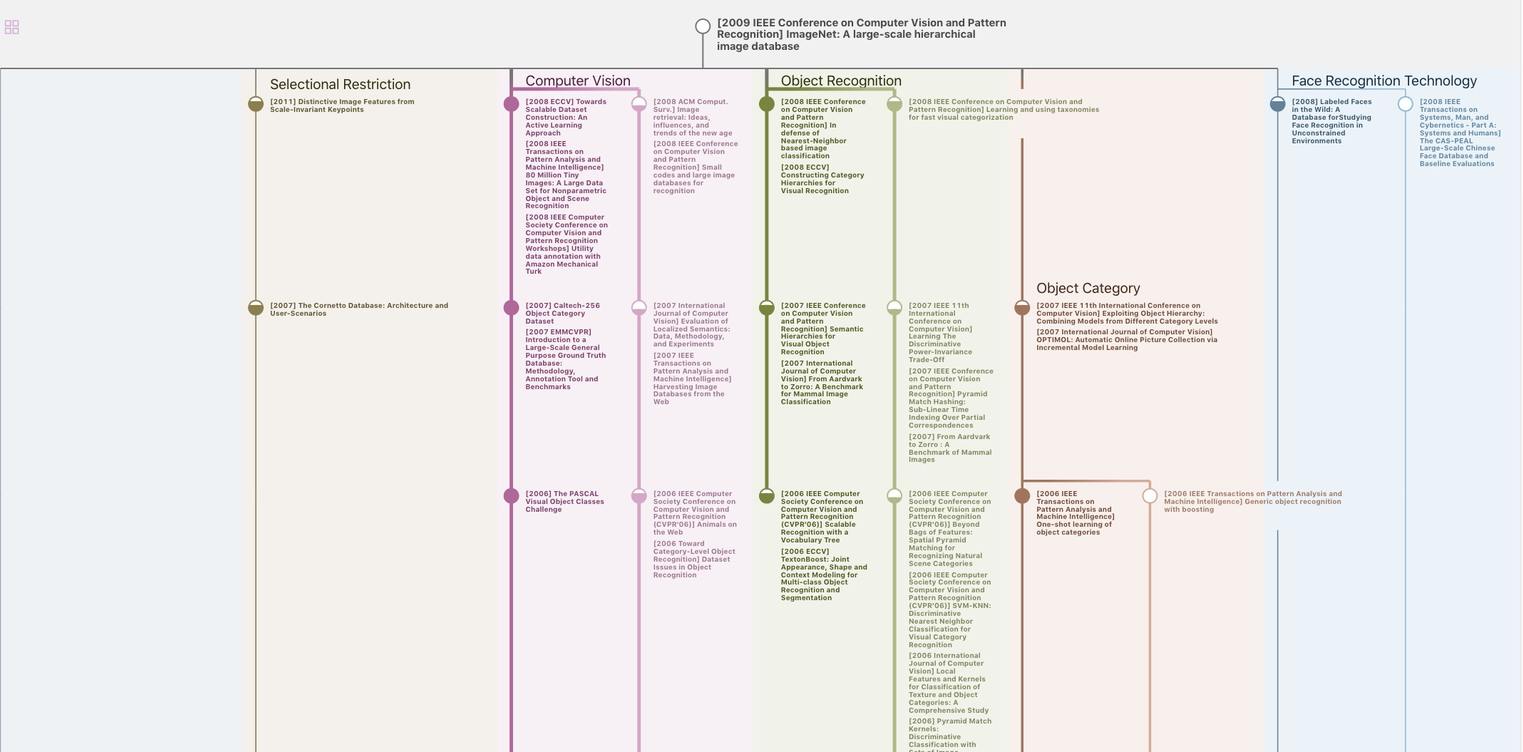
生成溯源树,研究论文发展脉络
Chat Paper
正在生成论文摘要