Harmonic variable-size dictionary learning for music source separation
Acoustics Speech and Signal Processing(2010)
摘要
Dictionary learning through matrix factorization has become widely popular for performing music transcription and source separation. These methods learn a concise set of dictionary atoms which represent spectrograms of musical objects. However, there is no guarantee that the atoms learned will be perceptually meaningful, particularly when there exists significant spectral and temporal overlap among the musical sources. In this paper, we propose a novel dictionary learning method that imposes additional harmonic constraints upon the atoms of the learned dictionary while allowing the dictionary size to grow appropriately during the learning procedure. When there is significant spectral-temporal overlap among the musical sources, our method outperforms popular existing matrix factorization methods as measured by the recall and precision of learned dictionary atoms.
更多查看译文
关键词
acoustic signal processing,harmonics,matrix decomposition,music,source separation,harmonic constraints,harmonic variable-size dictionary learning,matrix factorization,music source separation,music transcription,musical objects,Nonnegative matrix factorization,music transcription,pitch estimation,sparse coding
AI 理解论文
溯源树
样例
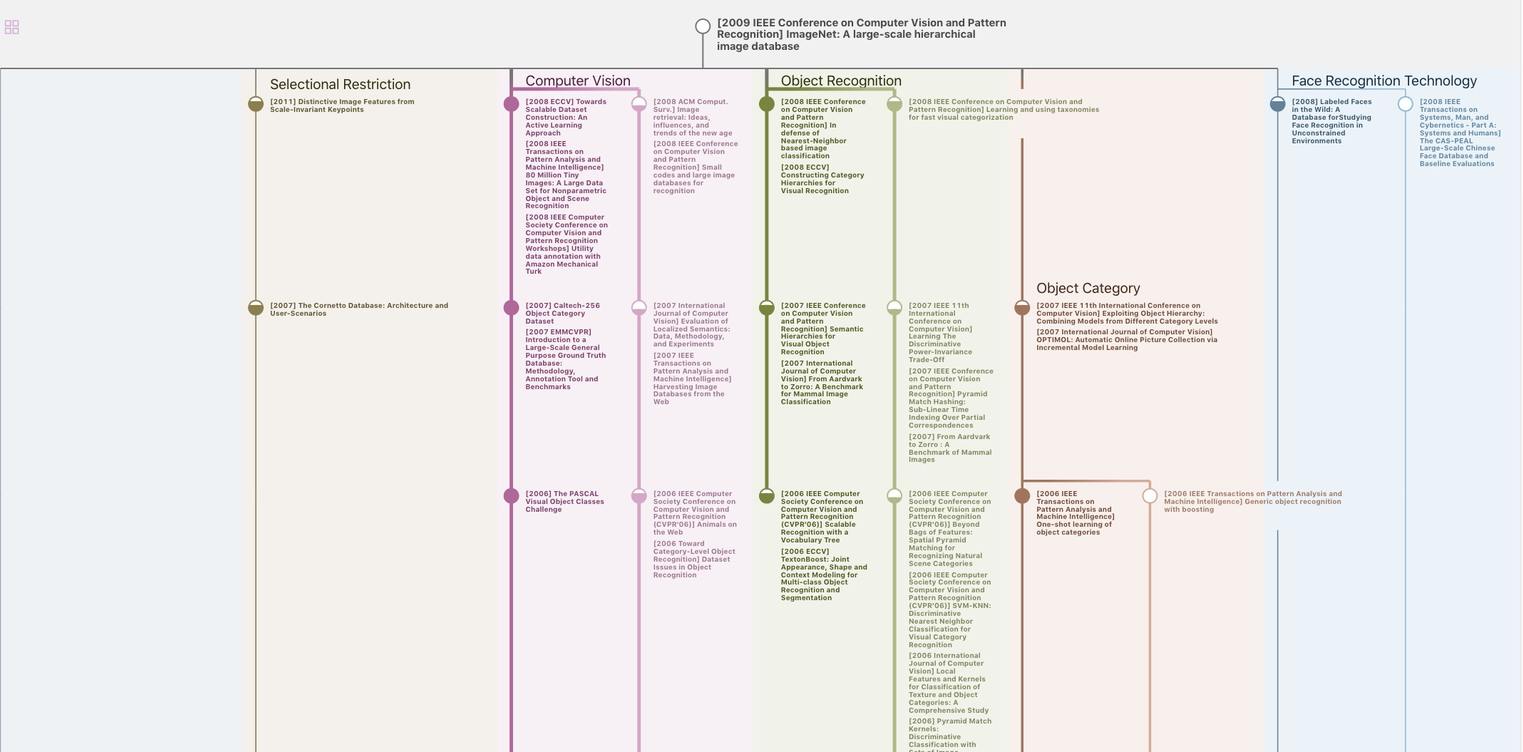
生成溯源树,研究论文发展脉络
Chat Paper
正在生成论文摘要