Morphology-based wavelet features and multiple mother wavelet strategy for spike classification in EEG signals.
EMBC(2012)
摘要
New wavelet-derived features and strategies that can improve autonomous EEG classifier performance are presented. Various feature sets based on the morphological structure of wavelet subband coefficients are derived and evaluated. The performance of these new feature sets is superior to Guler's classic features in both sensitivity and specificity. In addition, the use of (scalp electrode) spatial information is also shown to improve EEG classification. Finally, a new strategy based upon concurrent use of several mother wavelets is shown to result in increased sensitivity and specificity. Various attempts at reducing feature vector dimension are shown. A non-parametric method, k-NNR, is implemented for classification and 10-fold cross-validation is used for assessment.
更多查看译文
关键词
sensitivity,multiple mother wavelet strategy,wavelet transforms,eeg signals,biomedical electrodes,morphological structure,electroencephalography,medical signal processing,mathematical morphology,scalp electrode,feature extraction,wavelet subband coefficients,k-nnr,signal classification,wavelet features,spike classification,vectors,specificity
AI 理解论文
溯源树
样例
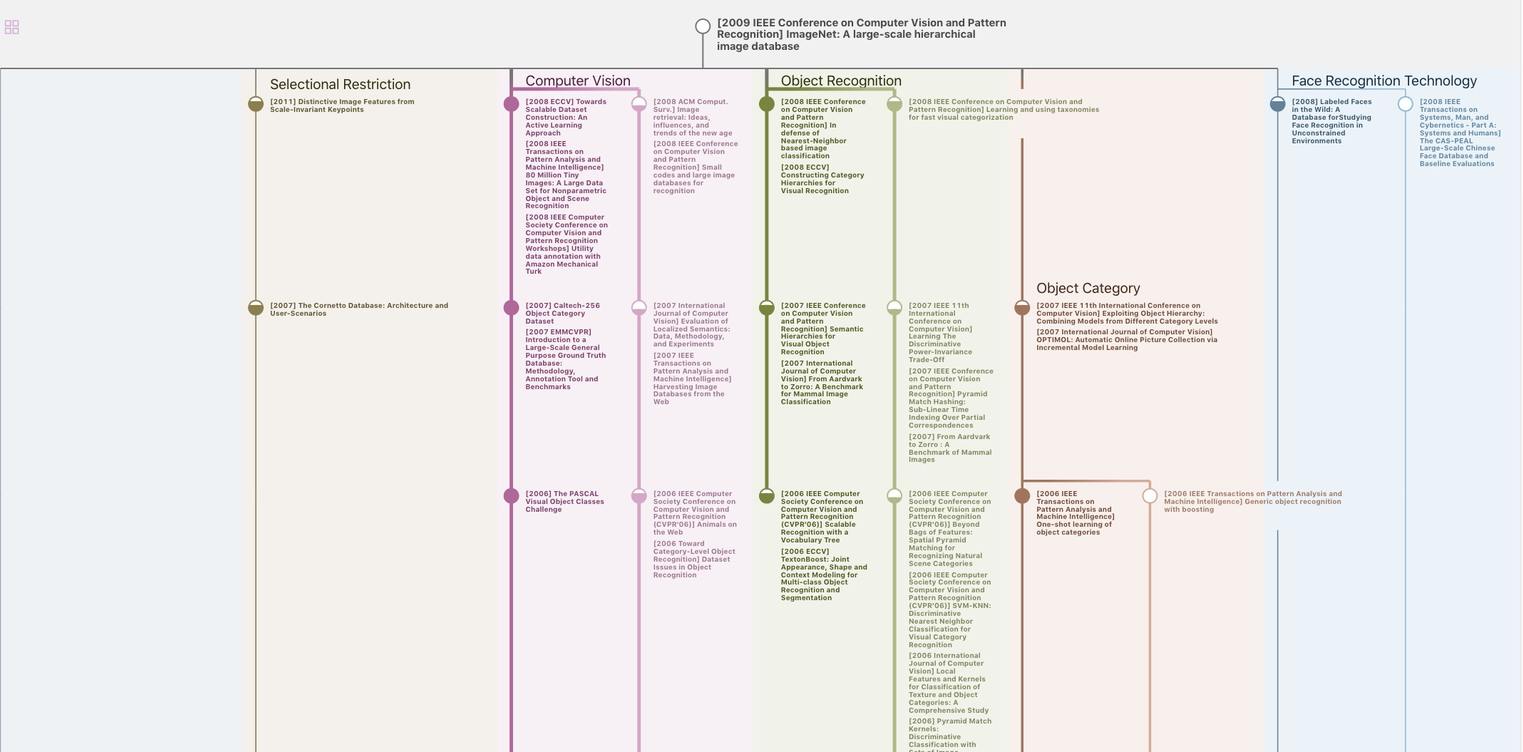
生成溯源树,研究论文发展脉络
Chat Paper
正在生成论文摘要