IVA and ICA: Use of diversity in independent decompositions
Signal Processing Conference(2012)
摘要
Starting with a simple linear generative model and the assumption of statistical independence of the underlying components, independent component analysis (ICA) decomposes a given set of observations by making use of the diversity in the data. Most of the ICA algorithms introduced to date have made use of one of the two types of diversity, non-Gaussianity or sample dependence. We first discuss the main results for ICA in terms of identifiability and performance with these two types of diversity, and then introduce independent vector analysis (IVA), generalization of ICA for decomposition of multiple datasets at a time. We show that the role of diversity in this case parallels that in ICA, and discuss identifiability conditions and performance bounds in a maximum likelihood framework.
更多查看译文
关键词
independent component analysis,maximum likelihood estimation,source separation,ICA algorithms,IVA algorithm,diversity,independent component analysis,independent decompositions,independent vector analysis,linear generative model,maximum likelihood framework,multiple dataset decomposition,source separation,statistical independence,Source separation,identifiability and performance,maximum likelihood
AI 理解论文
溯源树
样例
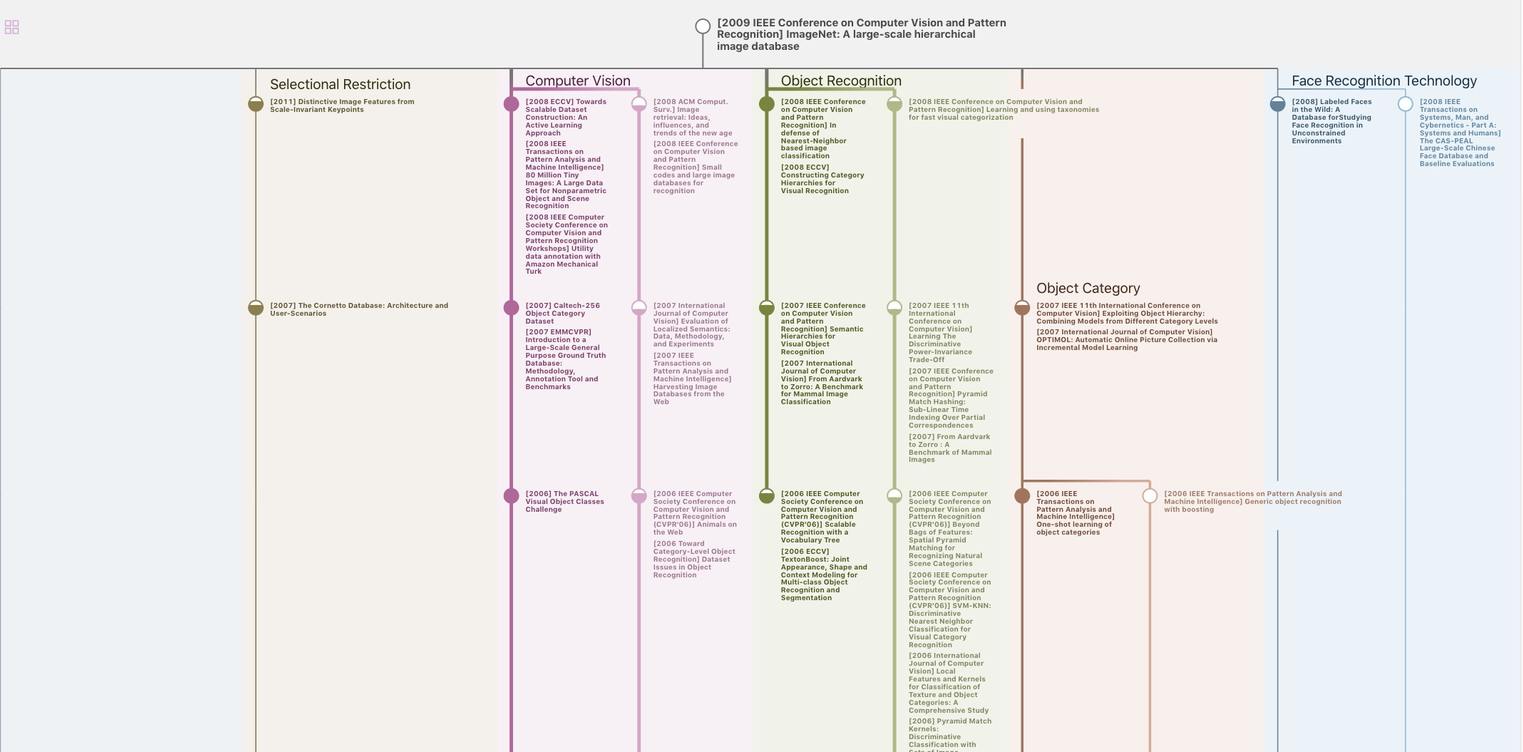
生成溯源树,研究论文发展脉络
Chat Paper
正在生成论文摘要