Early prediction of tilt test outcome, with support vector machine non linear classifier, using ECG, pressure and impedance signals
Hangzhou(2011)
摘要
The tilt test is a valuable clinical tool for the diagnosis of Vasovagal Syncope. No practical system has been implemented to predict the tilt test outcome at the beginning in the procedure. Our objective was to evaluate and benchmark, over a sufficient database, the predictive performance of the proposed parameters in the literature. We analyzed a database of 727 consecutive cases of tilt test. Previously proposed features were measured from heart rate and systolic/diastolic pressure, in several representative signal segments. A support vector machine (SVM) was used to predict the test outcome with the available features. Also the inclusion of additional physiological signals (impedance) was intended to improve the performance. The predictive performance of the nonline-arly combined previously proposed features was limited (p<;0.03 and area under ROC curve 0.57±0.12), especially in the beginning of the test, which is the most clinically relevant period. The improvement with additional available physiological information and SVM was limited (area under ROC curve 0.59±0.22). We conclude that the existing methods for tilt test outcome prediction knowledge should be considered with caution.
更多查看译文
关键词
electrocardiography,medical signal processing,pattern classification,physiology,signal classification,support vector machines,ecg,roc curve,svm,diastolic pressure signal,heart rate,impedance signal,linear classifier,physiological information,physiological signals,predictive performance,representative signal segment,support vector machine,systolic pressure signal,tilt test,vasovagal syncope diagnosis,databases,benchmark testing,machine learning
AI 理解论文
溯源树
样例
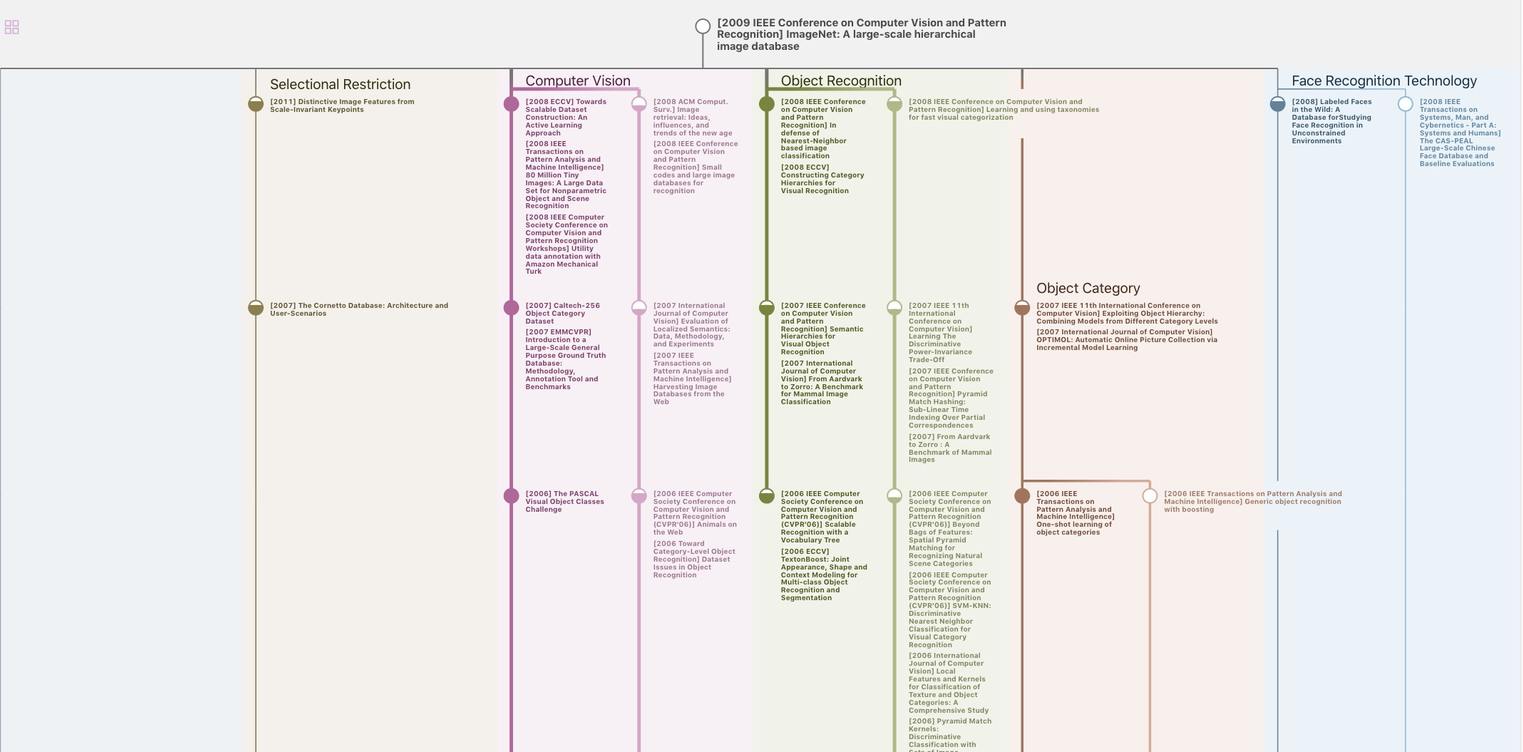
生成溯源树,研究论文发展脉络
Chat Paper
正在生成论文摘要