A new neural network approach for short-term glucose prediction using continuous glucose monitoring time-series and meal information.
EMBC(2011)
摘要
In the last decade, improvements in diabetes daily management have become possible thanks to the development of minimally-invasive portable sensors which allow continuous glucose monitoring (CGM) for several days. In particular, hypo and hyperglycemia can be promptly detected when glucose exceeds the normal range thresholds, and even avoided through the use of on-line glucose prediction algorithms. Several algorithms with prediction horizon (PH) of 15-30-45 min have been proposed in the literature, e.g. including AR/ARMA time-series modeling and neural networks. Most of them are fed by CGM signals only. The purpose of this work is to develop a new short-term glucose prediction algorithm based on a neural network that, in addition to past CGM readings, also exploits information on carbohydrates intakes quantitatively described through a physiological model. Results on simulated data quantitatively show that the new method outperforms other published algorithms. Qualitative preliminary results on a real diabetic subject confirm the potentialities of the new approach.
更多查看译文
关键词
neural network,minimally-invasive portable sensors,diseases,sugar,diabetes daily management,biomedical measurement,carbohydrates intakes,physiological model,medical diagnostic computing,time series,neural nets,short-term glucose prediction,continuous glucose monitoring,diabetes,time series model,sensors,predictive models,artificial neural network,artificial neural networks,prediction model,prediction algorithms
AI 理解论文
溯源树
样例
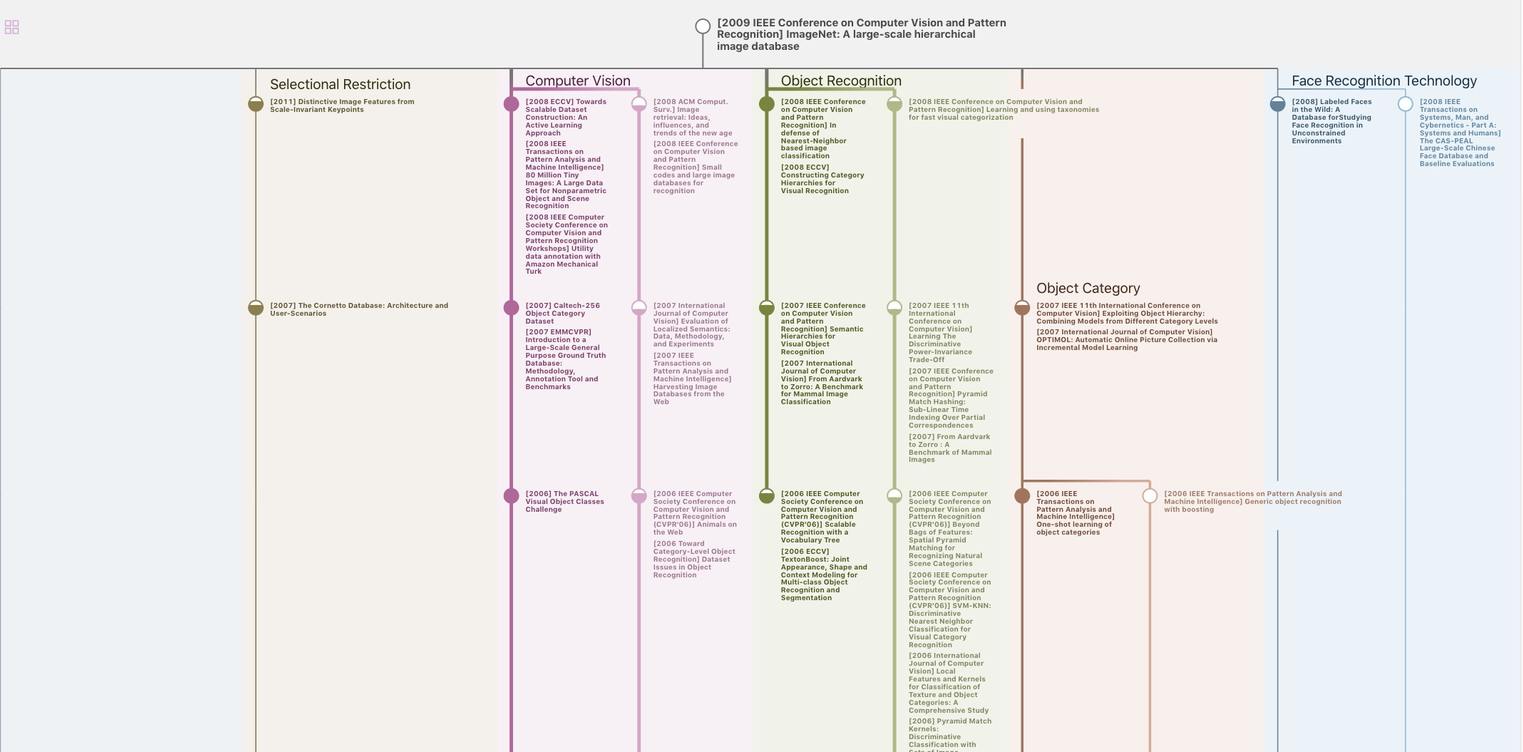
生成溯源树,研究论文发展脉络
Chat Paper
正在生成论文摘要