Unknown malcode detection via text categorization and the imbalance problem
Taipei(2008)
摘要
Todaypsilas signature-based anti-viruses are very accurate, but are limited in detecting new malicious code. Currently, dozens of new malicious codes are created every day, and this number is expected to increase in the coming years. Recently, classification algorithms were used successfully for the detection of unknown malicious code. These studies used a test collection with a limited size where the same malicious-benign-file ratio in both the training and test sets, which does not reflect real-life conditions. In this paper we present a methodology for the detection of unknown malicious code, based on text categorization concepts. We performed an extensive evaluation using a test collection that contains more than 30,000 malicious and benign files, in which we investigated the imbalance problem. In real-life scenarios, the malicious file content is expected to be low, about 10% of the total files. For practical purposes, it is unclear as to what the corresponding percentage in the training set should be. Our results indicate that greater than 95% accuracy can be achieved through the use of a training set that contains below 20% malicious file content.
更多查看译文
关键词
invasive software,pattern classification,text analysis,antiviruses,classification algorithm,imbalance problem,malicious code,malicious-benign-file ratio,test collection,text categorization,unknown malcode detection,Classification Algorithms,Malicious Code Detection
AI 理解论文
溯源树
样例
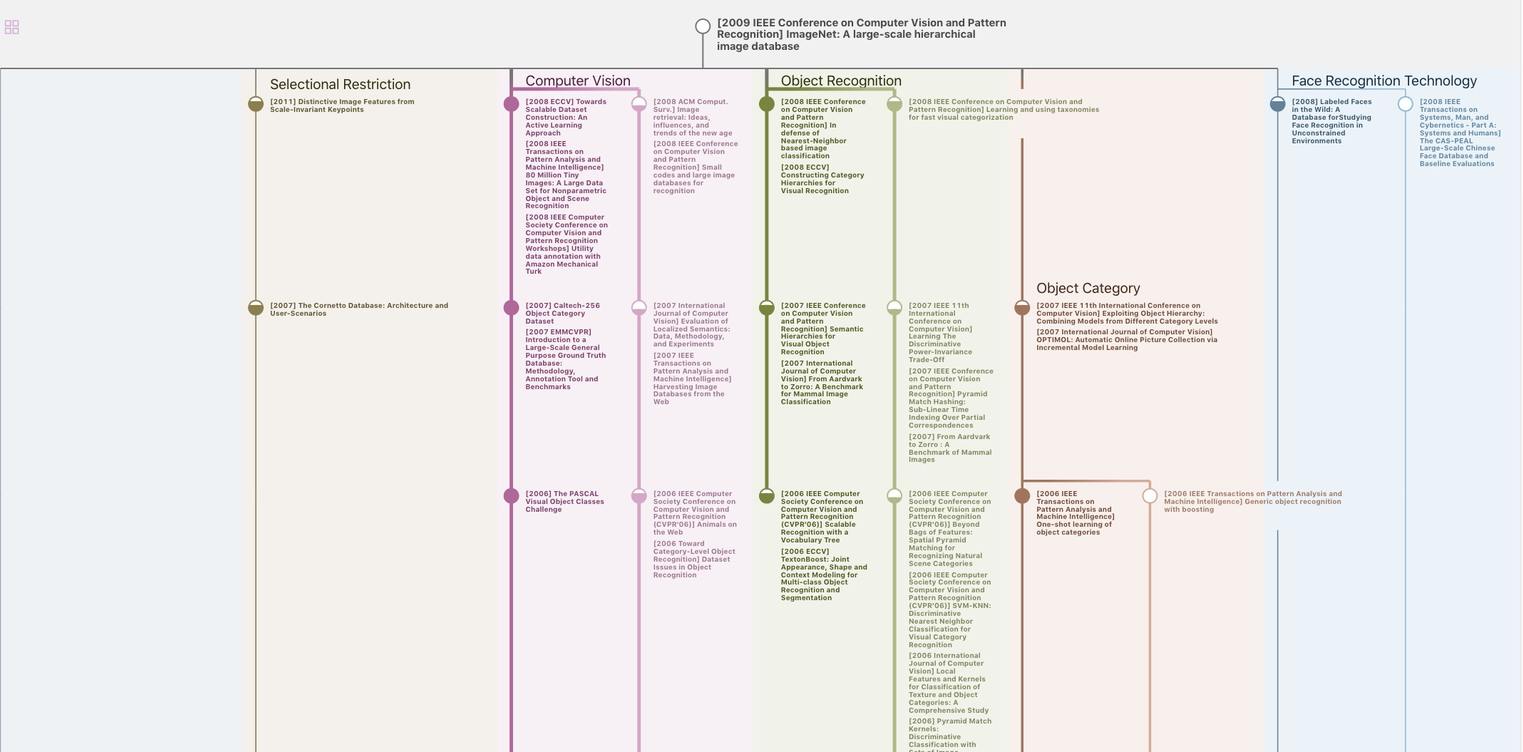
生成溯源树,研究论文发展脉络
Chat Paper
正在生成论文摘要