Combination of Recognizers and Fusion of Features Approach to Missing Data ASR Under Non-Stationary Noise Conditions
Acoustics, Speech and Signal Processing, 2007. ICASSP 2007. IEEE International Conference(2007)
摘要
The difficulty of ASR under non-stationary noise conditions is a major contributing factor hindering the widespread deployment of ASR systems. Bottom up techniques such as speech noise separation and top down methods to adapt the acoustic model to the environment have been applied to address the issue. The missing data approach to ASR improves upon existing techniques basing recognition solely on the reliable components of the signal and has been demonstrated as an effective method to handle non-stationarity. Proposed in this paper is a novel technique whereby ASR using missing data theory under non-stationary noise conditions is improved by use of a fusion of models at the decision level. This fused model introduces more resilient features to the missing data decode process. The fused decoder is found to significantly increase recognition performance over conventional missing data techniques. A major finding in this paper is when the fused decoder exhibits the fusion of bottom up and top down processes. Under this condition, the proposed combination of recognizers technique is found to outperform all other tested ASR systems.
更多查看译文
关键词
decoding,feature extraction,speech coding,speech recognition,acoustic model,features fusion,features recognizers,missing data ASR,missing data decode process,non-stationary noise conditions,speech noise separation,Hidden Markov models,Pattern recognition,Speech processing,Speech recognition,Time Series
AI 理解论文
溯源树
样例
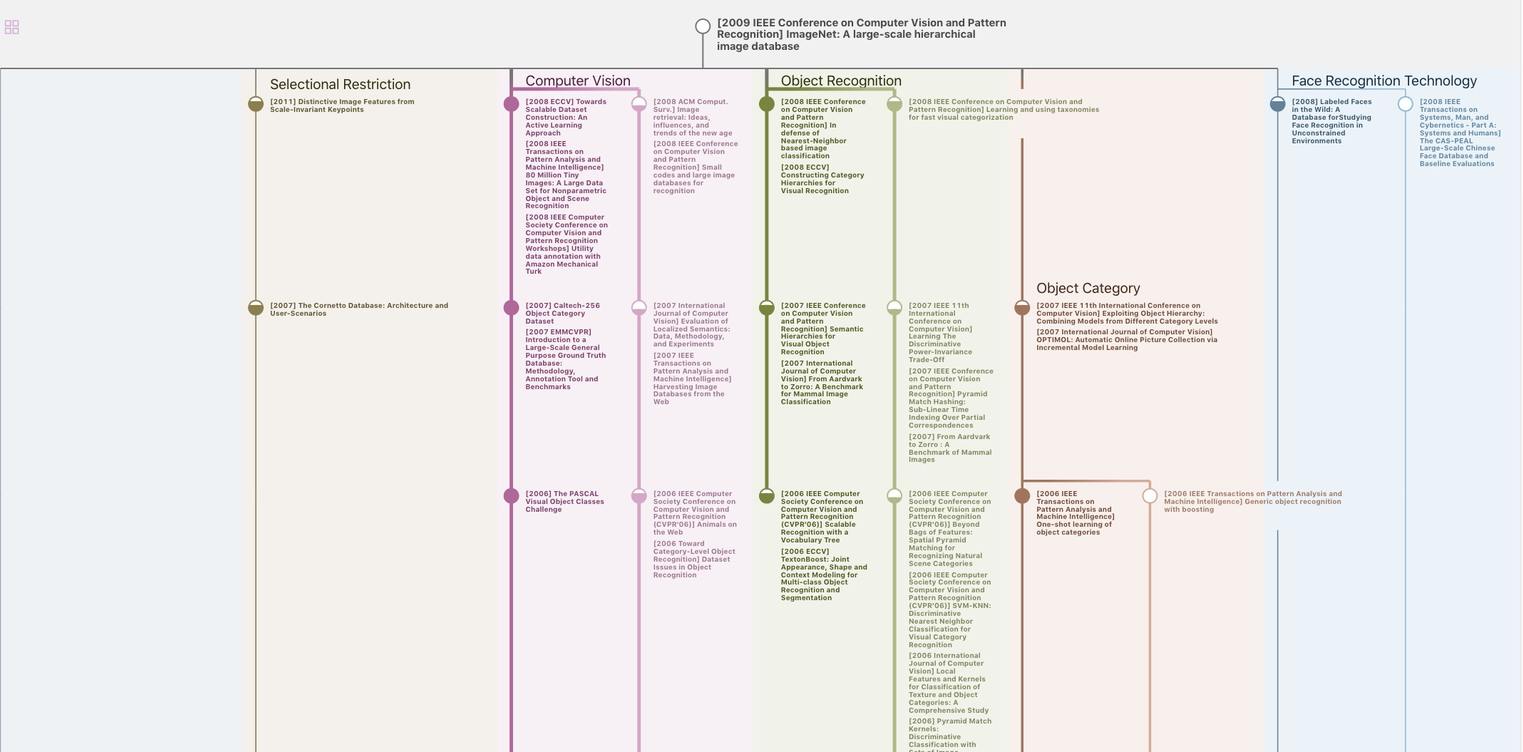
生成溯源树,研究论文发展脉络
Chat Paper
正在生成论文摘要