Exactly Sparse Delayed-State Filters
ICRA(2005)
摘要
This paper presents the novel insight that the SLAM information matrix is exactly sparse in a delayed-state framework. Such a framework is used in view-based representations of the environment which rely upon scan-matching raw sensor data. Scan-matching raw data results in virtual observations of robot motion with respect to a place its previously been. The exact sparseness of the delayed-state information matrix is in contrast to other recent feature based SLAM information algorithms like Sparse Extended Information Filters or Thin Junction Tree Filters. These methods have to make approximations in order to force the feature-based SLAM information matrix to be sparse. The benefit of the exact sparseness of the delayed-state framework is that it allows one to take advantage of the information space parameterization without having to make any approximations. Therefore, it can produce equivalent results to the “full-covariance” solution.
更多查看译文
关键词
Delayed states,EIF,SLAM,Delayed states,EIF,SLAM
AI 理解论文
溯源树
样例
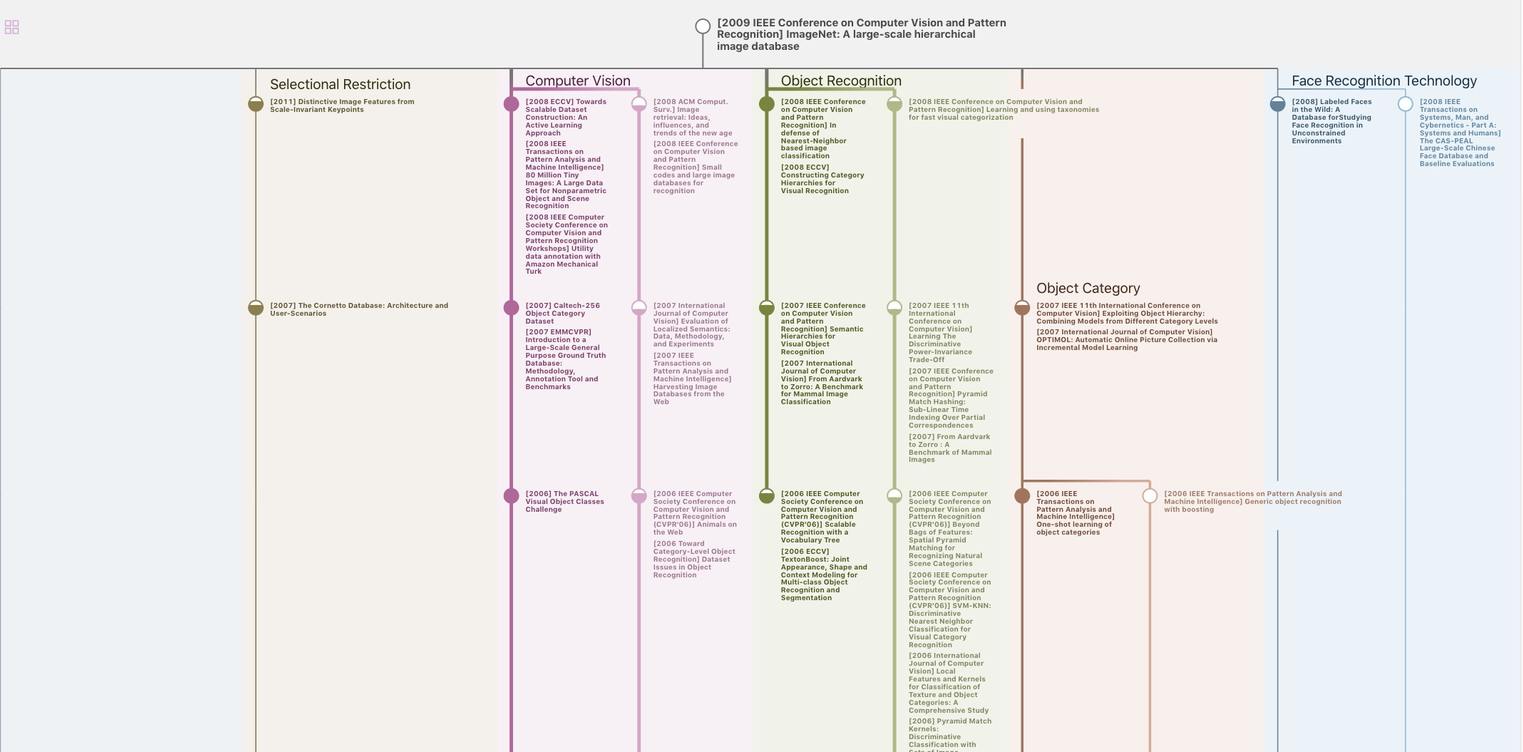
生成溯源树,研究论文发展脉络
Chat Paper
正在生成论文摘要