Detecting Group Interest-Level in Meetings
ICASSP '05). IEEE International Conference(2005)
摘要
Finding relevant segments in meeting recordings is important for summarization, browsing, and retrieval purposes. In this paper, we define relevance as the interest-level that meeting participants manifest as a group during the course of their interaction (as per- ceived by an external observer), and investigate the automatic de- tection of segments of high-interest from audio-visual cues. This is motivated by the assumption that there is a relationship between segments of interest to participants, and those of interest to the end user, e.g. of a meeting browser. We first address the prob- lem of human annotation of group interest-level. On a 50-meeting corpus, recorded in a room equipped with multiple cameras and microphones, we found that the annotations generated by multi- ple people exhibit a good degree of consistency, providing a stable ground-truth for automatic methods. For the automatic detection of high-interest segments, we investigate a methodology based on Hidden Markov Models (HMMs) and a number of audio and visual features. Single- and multi-stream approaches were studied. Using precision and recall as performance measures, the results suggest that the automatic detection of group interest-level is promising, and that while audio in general constitutes the predominant modal- ity in meetings, the use of a multi-modal approach is beneficial.
更多查看译文
关键词
audio recording,hidden Markov models,information retrieval,speech processing,HMM,audio-visual cues,automatic detection,browsing,group interest-level,hidden Markov models,human annotation,meeting recordings,multi-modal approach,multi-stream approach,performance measures,precision,recall,relevance,relevant segments,retrieval,single-stream approach,summarization
AI 理解论文
溯源树
样例
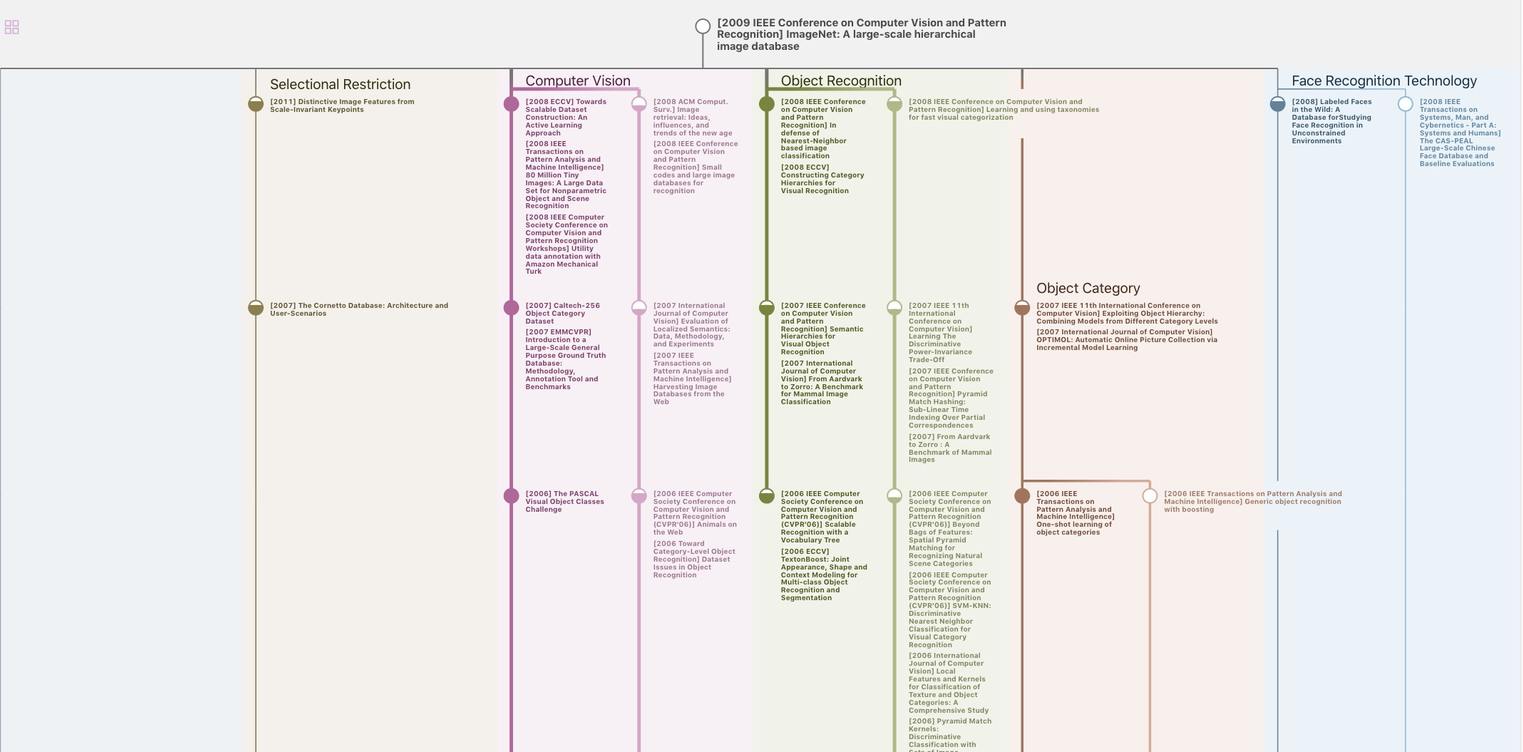
生成溯源树,研究论文发展脉络
Chat Paper
正在生成论文摘要