Information theoretic clustering of sparse cooccurrence data
international conference on data mining(2003)
摘要
A novel approach to clustering co-occurrence data posesit as an optimization problem in information theory whichminimizes the resulting loss in mutual information. A divisiveclustering algorithm that monotonically reduces thisloss function was recently proposed. In this paper we showthat sparse high-dimensional data presents special challengeswhich can result in the algorithm getting stuck atpoor local minima. We propose two solutions to this problem:(a) a "prior" to overcome infinite relative entropy valuesas in the supervised Naive Bayes algorithm, and (b)local search to escape local minima. Finally, we combinethese solutions to get a robust algorithm that is computationallyefficient. We present experimental results to showthat the proposed method is effective in clustering documentcollections and outperforms previous information-theoreticclustering approaches.
更多查看译文
关键词
bayes methods,information theory,learning (artificial intelligence),optimisation,pattern clustering,divisive clustering algorithm,document clustering,local minima,sparse high-dimensional cooccurrence data,supervised naive bayes algorithm,loss function,high dimensional data,learning artificial intelligence,relative entropy,local search,probability distribution,naive bayes,optimization problem,mutual information,random variable
AI 理解论文
溯源树
样例
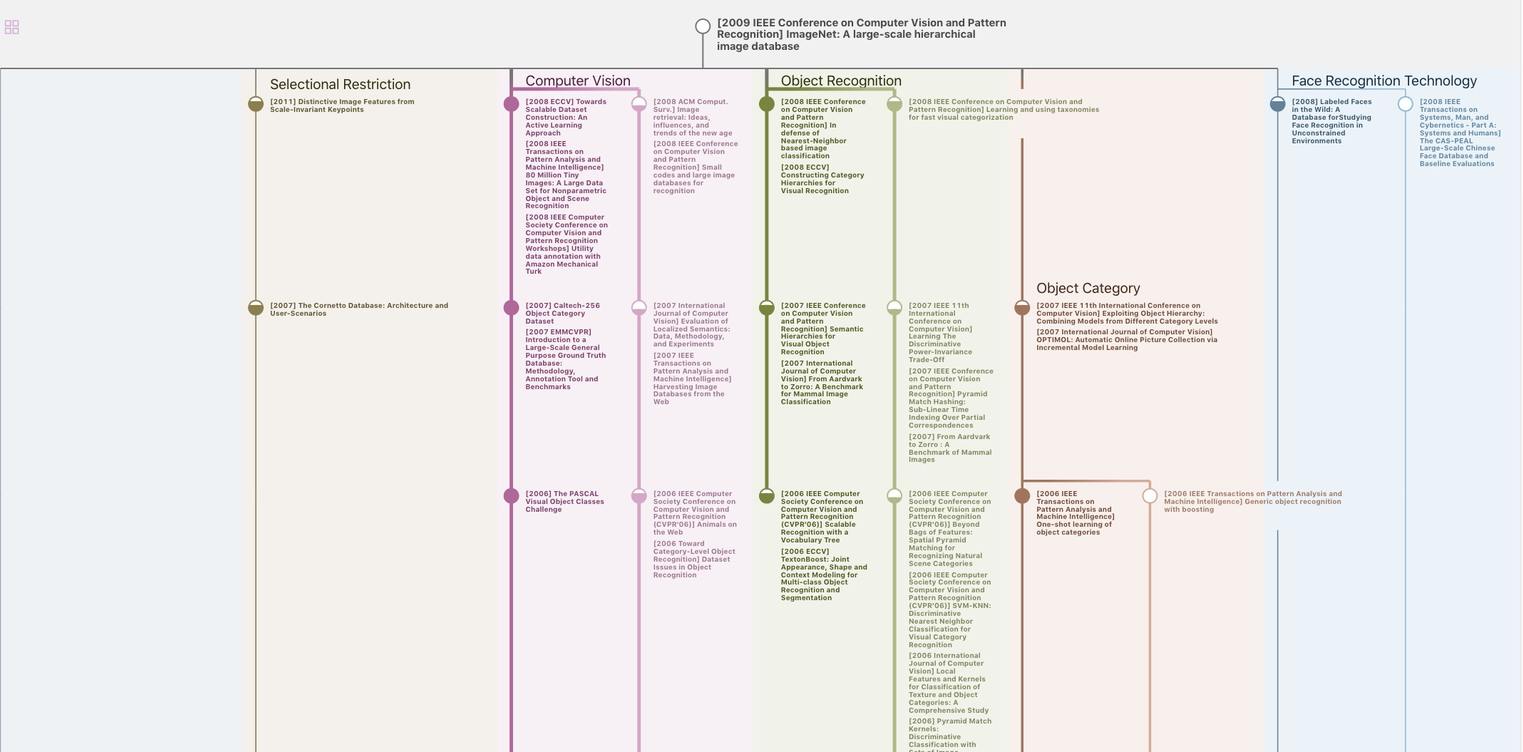
生成溯源树,研究论文发展脉络
Chat Paper
正在生成论文摘要