Artificial neural network features for speaker diarization
Spoken Language Technology Workshop(2014)
摘要
Speaker diarization finds contiguous speaker segments in an audio recording and clusters them by speaker identity, without any a-priori knowledge. Diarization is typically based on short-term spectral features such as Mel-frequency cepstral coefficients (MFCCs). Though these features carry average information about the vocal tract characteristics of a speaker, they are also susceptible to factors unrelated to the speaker identity. In this study, we propose an artificial neural network (ANN) architecture to learn a feature transform that is optimized for speaker diarization. We train a multi-hidden-layer ANN to judge whether two given speech segments came from the same or different speakers, using a shared transform of the input features that feeds into a bottleneck layer. We then use the bottleneck layer activations as features, either alone or in combination with baseline MFCC features in a multistream mode, for speaker diarization on test data. The resulting system is evaluated on various corpora of multi-party meetings. A combination of MFCC and ANN features gives up to 14% relative reduction in diarization error, demonstrating that these features are providing an additional independent source of knowledge.
更多查看译文
关键词
neural nets,speaker recognition,transforms,MFCC,artificial neural network architecture,artificial neural network features,audio recording,contiguous speaker segments,feature transform,mel-frequency cepstral coefficients,multihidden-layer ANN,multiparty meetings,multistream mode,shared transform,short-term spectral features,speaker diarization,speaker identity,speech segments,vocal tract characteristics,artificial neural networks,discriminative feature extraction,speaker diarization
AI 理解论文
溯源树
样例
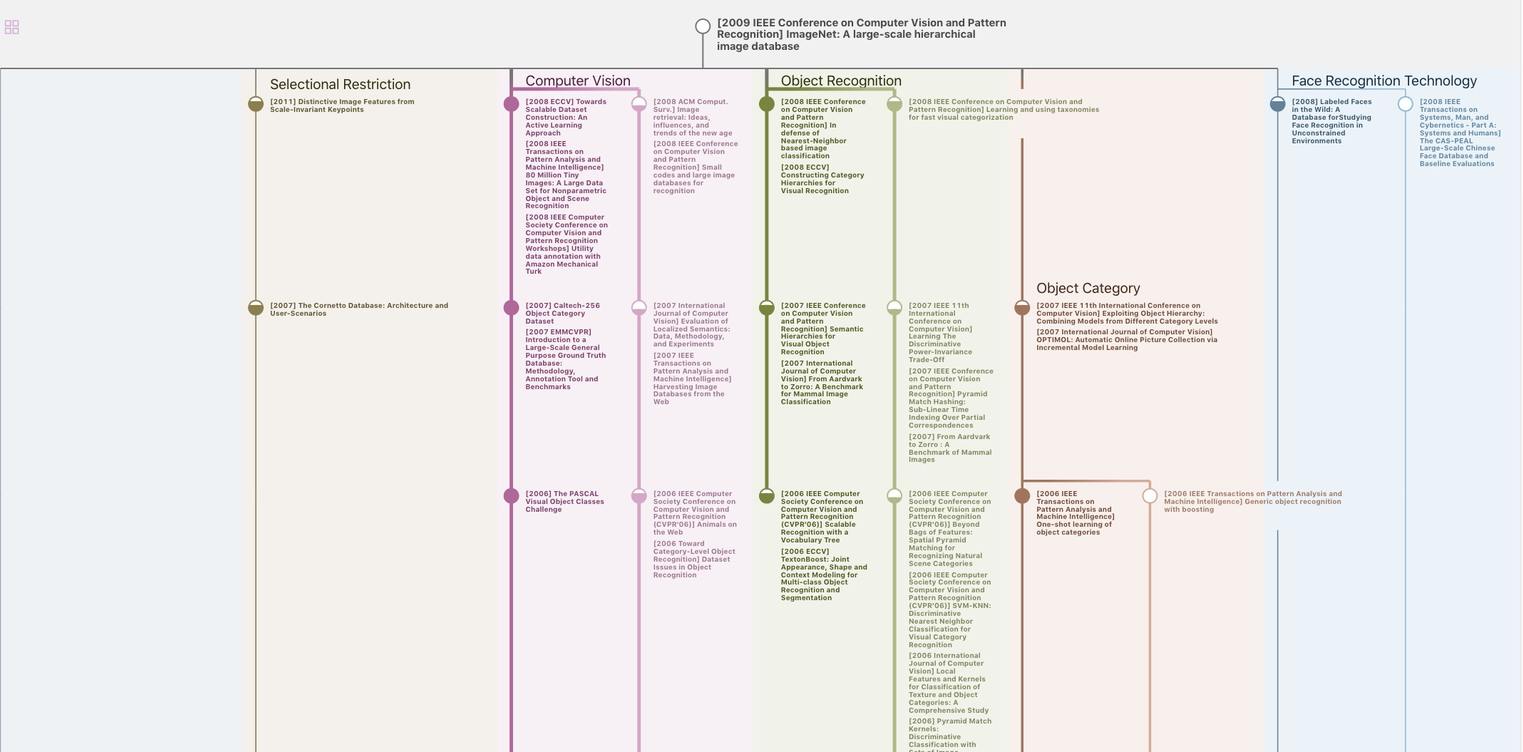
生成溯源树,研究论文发展脉络
Chat Paper
正在生成论文摘要