Scalable Infrastructures for Data in Motion
Cluster, Cloud and Grid Computing(2014)
摘要
Analytics applications for reporting and human interaction with big data rely upon scalable frameworks for data ingest, storage, and computation. Batch processing of analytic workloads increases latency of results and can perform redundant computation. In real-world applications, new data points are continuously arriving and a suite of algorithms must be updated to reflect the changes. Reducing the latency of re-computation by keeping algorithms online and up-to-date enables fast query, experimentation, and drill-down. In this paper, we share our experiences designing and implementing scalable infrastructure around No SQL databases for social media analytics applications. We propose a new heterogeneous architecture and execution model for streaming data applications that focuses on throughput and modularity.
更多查看译文
关键词
Big Data,SQL,data analysis,social networking (online),NoSQL databases,analytic workloads,batch processing,big data,data in motion,data ingest,data storage,execution model,heterogeneous architecture,recomputation latency reduction,redundant computation,scalable infrastructures,social media analytics applications,streaming data applications
AI 理解论文
溯源树
样例
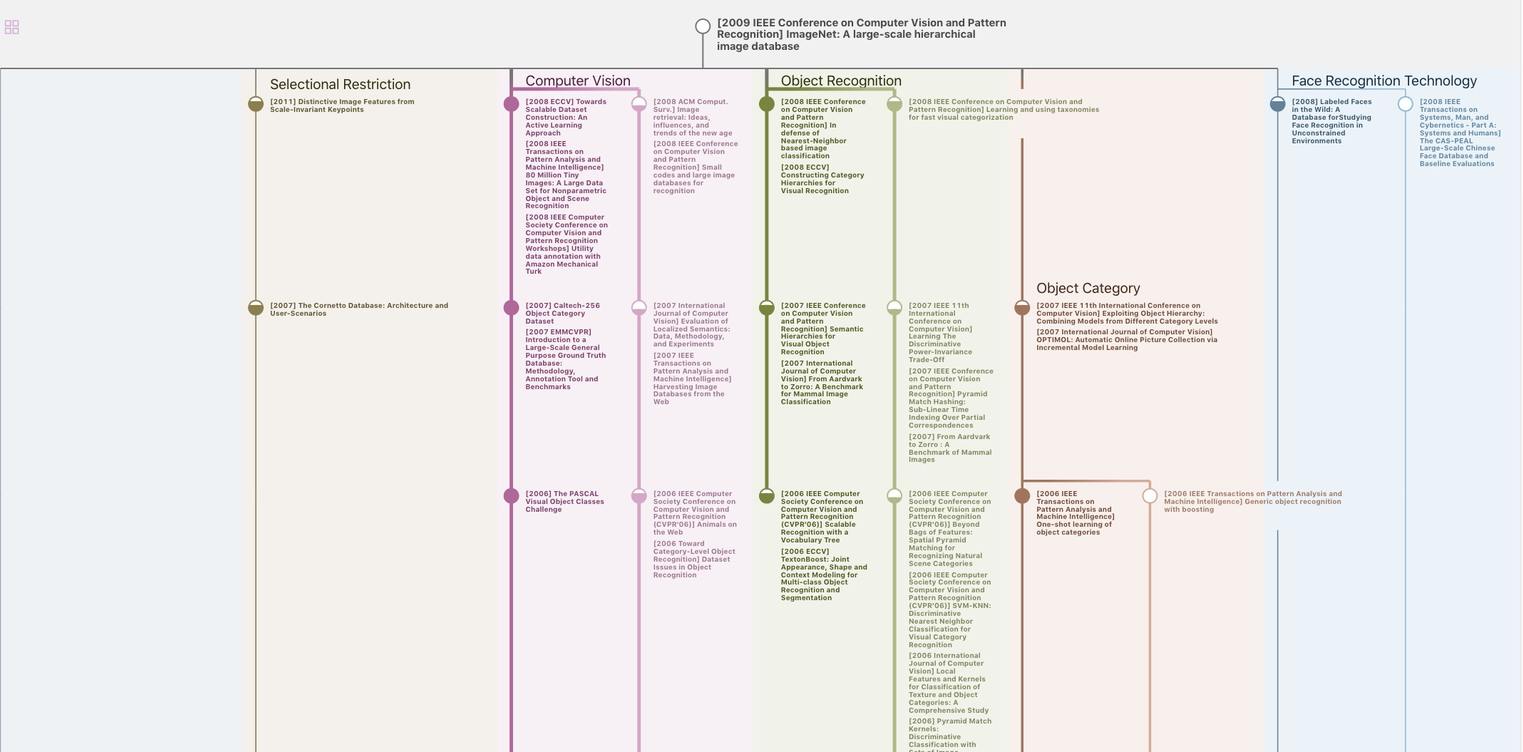
生成溯源树,研究论文发展脉络
Chat Paper
正在生成论文摘要