IMAGE DENOISING USING HYPER-LAPLACIAN PRIORS AND GRADIENT HISTOGRAM PRESERVATION MODEL
2014 12TH INTERNATIONAL CONFERENCE ON SIGNAL PROCESSING (ICSP)(2014)
摘要
Image noise is difficult to avoid during the image acquisition and communication, and thus we need to suppress noise in the low level vision. Among all of the existing image denoising methods, image priors, such as hyper-Laplacian priors of the heavy-tailed distribution of image gradient, play an important role. However, many denoising methods tend to smooth the fine textures while suppressing noise, degrading the image visual quality. In order to solve this problem, we introduce a gradient histogram preservation (GHP) model. Combining the GHP model with the hyper-Laplacian priors, we can improve the denoising performance. We use the alternating minimization scheme, among the two phases is a non-convex L-p - minimization problem. The problem can be solved by two methods, the lookup table (LUT) and the generalized iterative shrinkage algorithm (GISA). Furthermore, the experimental results demonstrate that with the gradient histogram preservation model, we can preserve the image textures while removing noise, leading to a much more natural denoising result.
更多查看译文
关键词
image denoising,gradient histogram perservation,hyper-Laplacian,alternating minimization
AI 理解论文
溯源树
样例
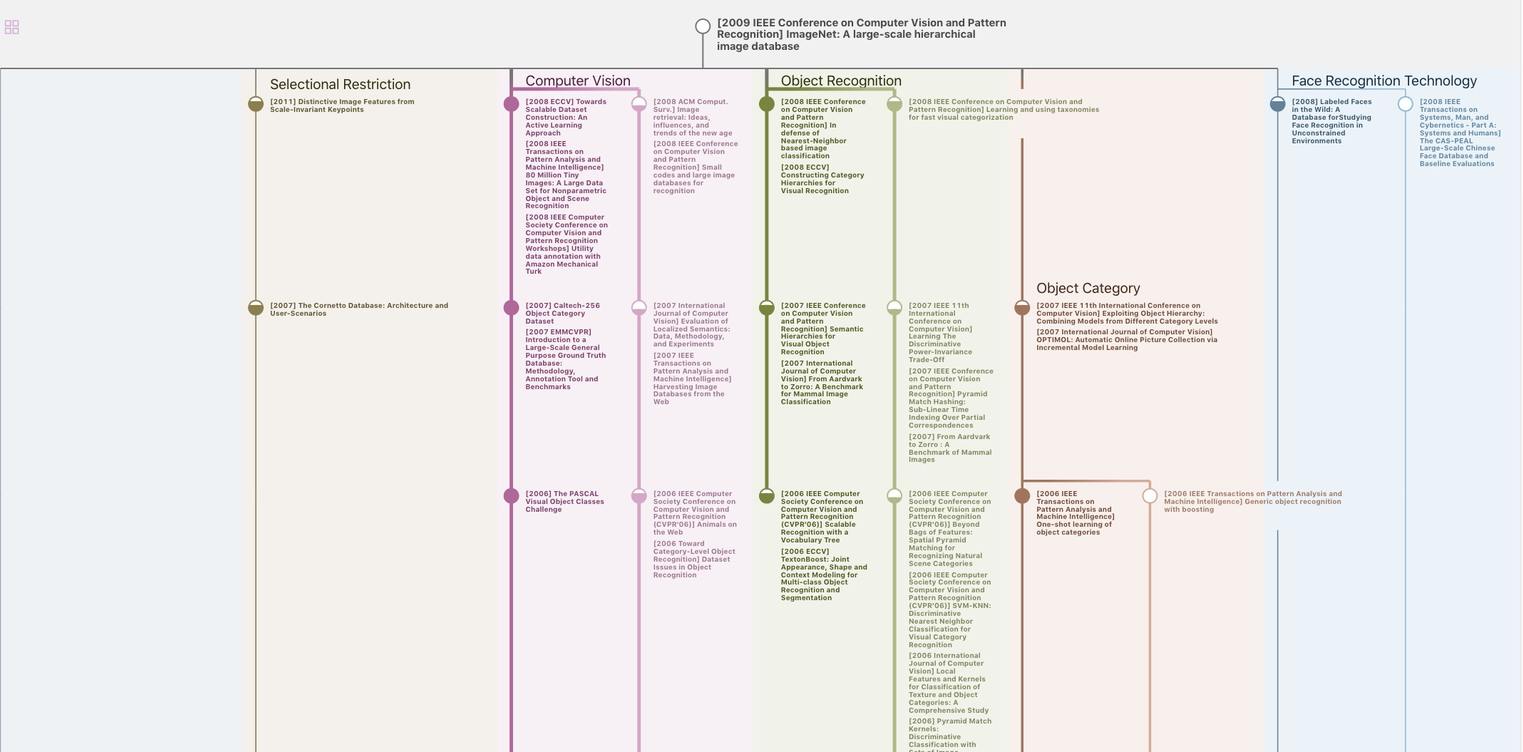
生成溯源树,研究论文发展脉络
Chat Paper
正在生成论文摘要