Robust feature extractors for continuous speech recognition
Signal Processing Conference(2015)
摘要
This paper presents robust feature extractors for a continuous speech recognition task in matched and mismatched environments. The mismatched conditions may occur due to additive noise, different channel, and acoustic reverberation. In the conventional Mel-frequency cepstral coefficient (MFCC) feature extraction framework, a subband spectrum enhancement technique is incorporated to improve its robustness. We denote this front-end as robust MFCCs (RMFCC). Based on the gammatone and compressive gammachirp filter-banks, robust gammatone filterbank cepstral coefficients (RGFCC) and robust compressive gammachirp filterbank cepstral coefficients (RCGCC) are also presented for comparison. We also employ low-variance spectrum estimators such as multitaper, regularized minimum- variance distortionless response (RMVDR), instead of a discrete Fourier transform-based direct spectrum estimator for improving robustness against mismatched environments. Speech recognition performances of the robust feature extractors are evaluated in clean as well as multi-style training conditions of the AURORA-4 continuous speech recognition task. Experimental results depict that the RMFCC and low-variance spectrum-estimators-based robust feature extractors outperformed the MFCC, PNCC (power normalized cepstral coefficients), and ETSI-AFE features both in clean and multi-condition training conditions.
更多查看译文
关键词
channel bank filters,discrete Fourier transforms,feature extraction,speech recognition,AURORA-4 continuous speech recognition task,RCGCC,RGFCC,conventional Mel-frequency cepstral coefficient feature extraction framework,low-variance spectrum estimators,robust MFCC,robust compressive gammachirp filterbank cepstral coefficients,robust feature extractors,robust gammatone filterbank cepstral coefficients,Robust feature extractor,aurora 4,multi-style training,multitaper,speech recognition
AI 理解论文
溯源树
样例
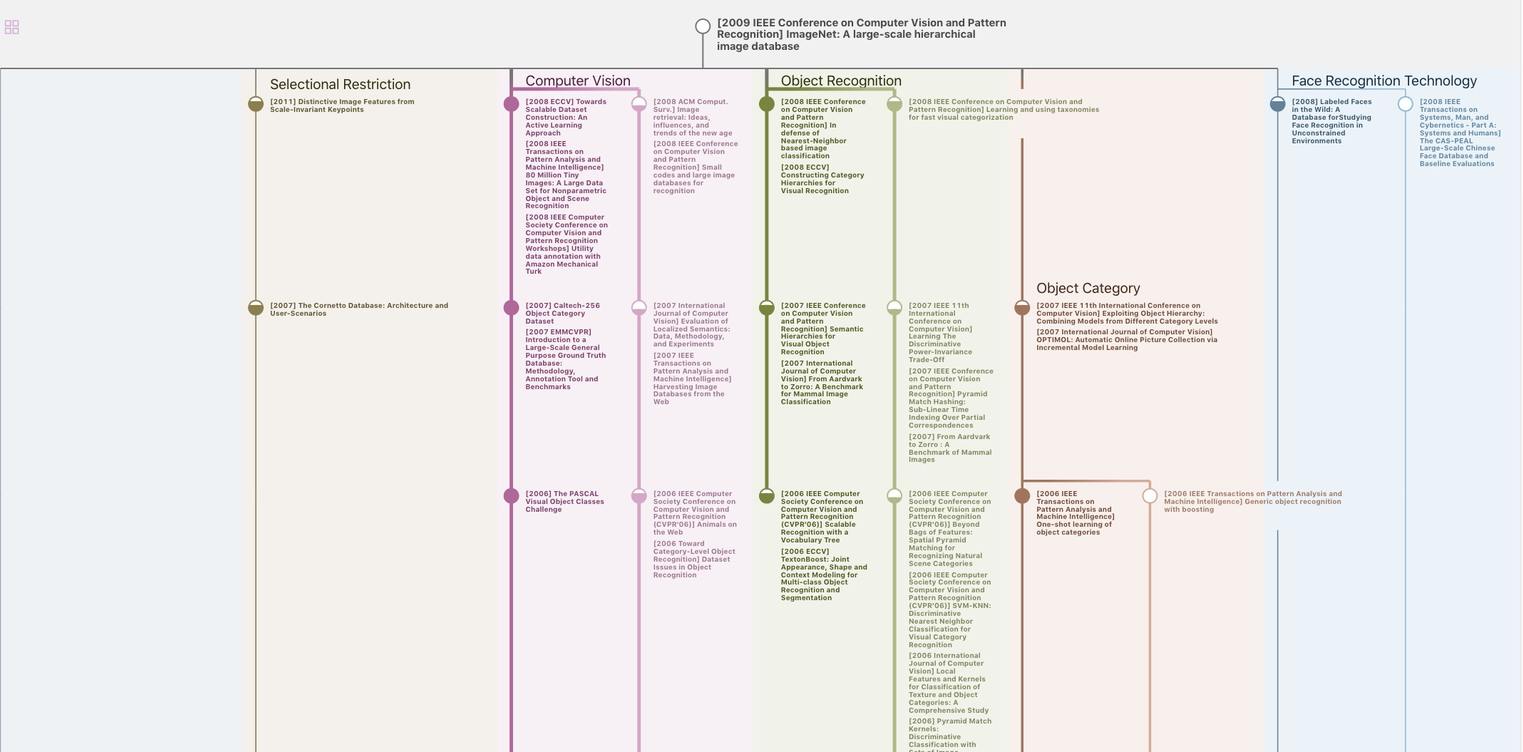
生成溯源树,研究论文发展脉络
Chat Paper
正在生成论文摘要