Enabling scalable publish/subscribe for logical-clustering in crowdsourcing via Mediasense
Science and Information Conference(2014)
摘要
Crowdsourcing was initially devised as a method for solving problems through soliciting contributions from a large online community. Crowdsourcing is facing new challenges to handle the increase of information in real-time from a vast number of sources in Internet-of-Things (IoT) scenarios. Thus we seek to leverage the power of social web, smart-devices, sensors, etc., fusing these heterogeneous sources into distributed context information in order to enable novel crowdsourcing scenarios. This mandates research in efficient management of heterogeneous and distributed context information through logical-clustering. Logical-clustering can efficiently filter out similar context information obtained from distributed sources based on context similarity. However, the efficiency of logical-clustering is challenged by the distribution of context information in crowdsourcing scenarios. Publish/Subscribe mechanism can counter this challenge. To this end, we propose a scalable publish/subscribe model, MediaSense, which is based on p2p technologies. This paper presents our approach to a scalable logical-clustering concept. The evaluation of our approach applied to MediaSense can achieve a rate of approximately 3530 messages/sec for publish/subscribe events. Moreover, this approach further achieves 99% increase for subscription matching and 163% improvement in memory requirements in comparison with other approaches.
更多查看译文
关键词
internet of things,pattern clustering,peer-to-peer computing,iot scenarios,mediasense,crowdsourcing scenarios,distributed context information,online community,p2p technologies,publish/subscribe events,scalable logical clustering,scalable publish/subscribe mechanism,scalable publish/subscribe model,sensors,smart devices,social web,subscription matching,publish/subscribe,context information,crowdsourcing,logical-clustering,pervasive computing,information systems
AI 理解论文
溯源树
样例
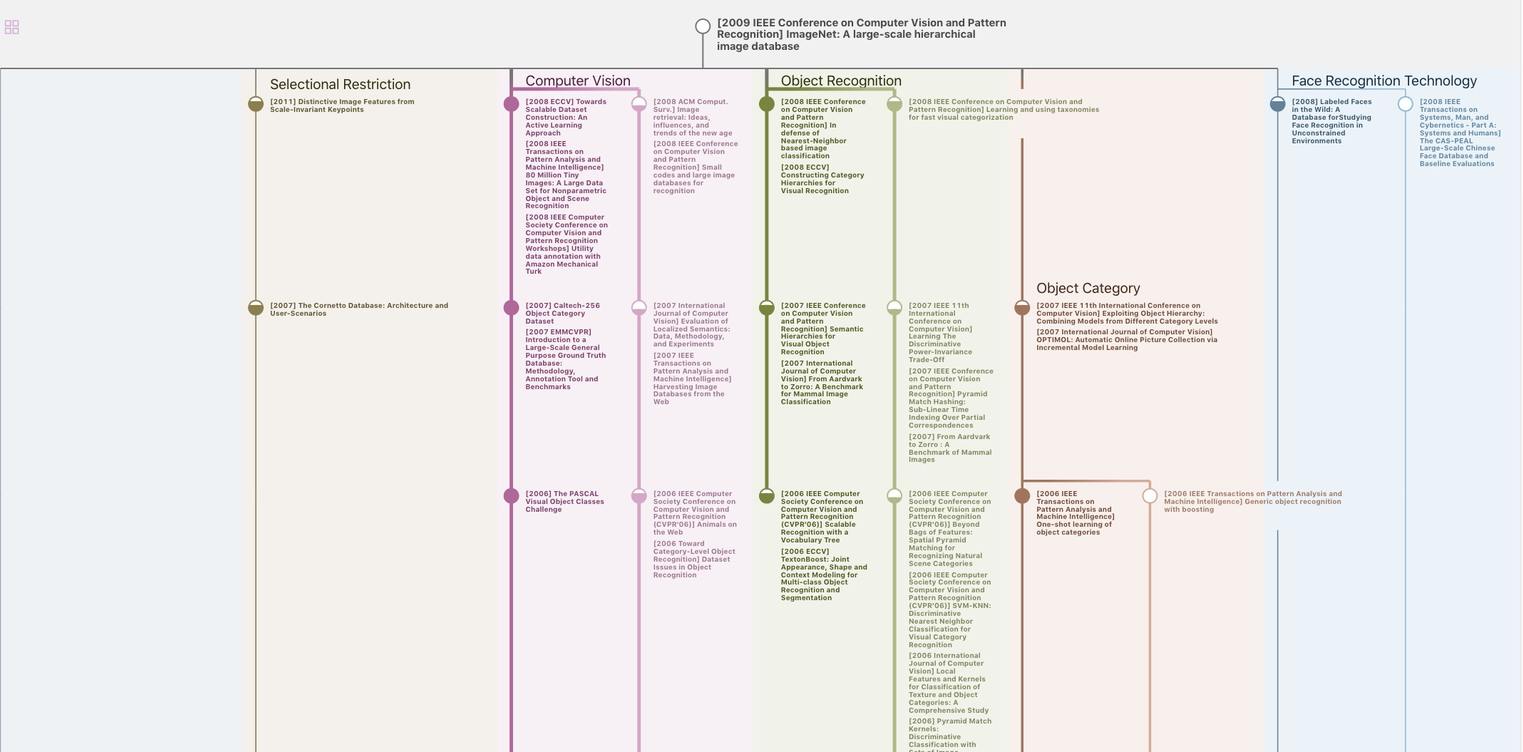
生成溯源树,研究论文发展脉络
Chat Paper
正在生成论文摘要