Basis selection for maximally independent EEG sources.
EMBC(2014)
摘要
We suggest a solution to the following problem: "Given multichannel linear source mixture data Y, and an overcomplete dictionary, A, of source projections, ai, how can we construct a complete basis, A0, by selecting columns from A such that the sources X = A0(-1)Y are as statistically independent as possible from each other?". While conventional independent component analysis (ICA) methods learn the mixing matrix A0 from scratch given Y, we restrict ourselves to selecting basis vectors from a known overcomplete dictionary. We develop two methods based on modifications of the maximum likelihood equivalent of the Infomax approach and the reconstruction-ICA (RICA) algorithm. We show that on realistic synthetic electroencephalographic (EEG) data our algorithms can find the true sources in the case of a highly coherent dictionary while requiring relatively fewer data points compared to other algorithms. On real EEG data, our algorithms obtain higher mutual information reduction.
更多查看译文
关键词
bioelectric potentials,neurophysiology,maximum likelihood estimation,electroencephalography,medical signal processing,independent component analysis,reconstruction-ica algorithm,maximally independent eeg sources,infomax approach,independent component analysis methods,given multichannel linear source mixture data,maximum likelihood equivalent modifications,realistic synthetic electroencephalographic data
AI 理解论文
溯源树
样例
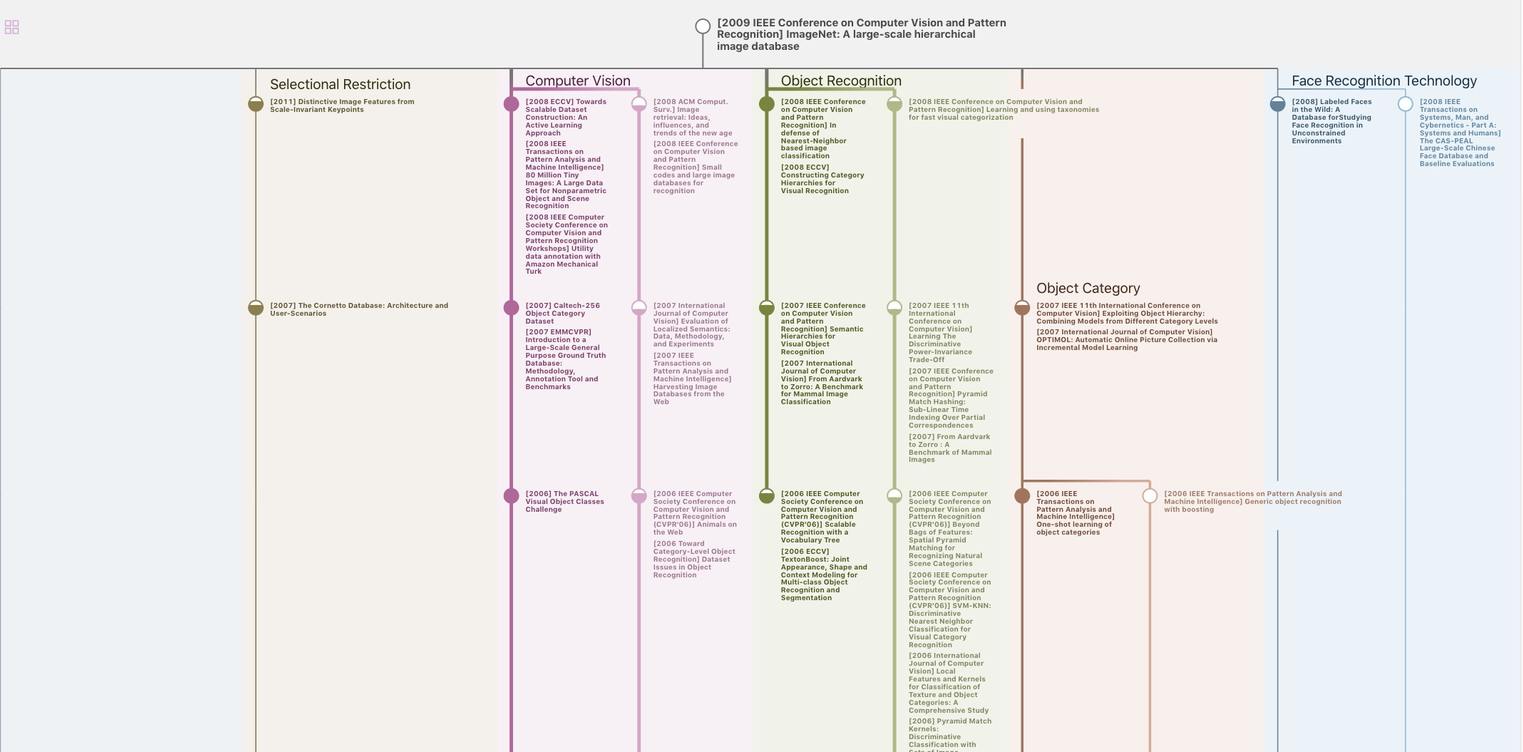
生成溯源树,研究论文发展脉络
Chat Paper
正在生成论文摘要