Predicting protein localization using a domain adaptation na¨ıve Bayes classifier with burrows wheeler transform features
Bioinformatics and Biomedicine(2014)
摘要
The reduced cost of the next generation sequencing technologies provides opportunities to study non-model organisms. However, one challenge is the large volume of data generated and, thus, the need to use automated approaches to annotate these data. Machine learning algorithms could provide a cost-effective solution but they need lots of labeled data and informative features to represent these data. Our proposed approach addresses both these problems by using a domain adaptation classifier in conjunction with features generated with unsupervised techniques to annotate biological sequence data.
更多查看译文
关键词
Bayes methods,adaptive systems,bioinformatics,classification,data analysis,data structures,feature extraction,learning (artificial intelligence),macromolecules,molecular biophysics,molecular configurations,proteins,sequences,transforms,Burrows-Wheeler transform features,automated data annotation,biological sequence data annotation,cost-effective solution,data generation,data labeling,data representation,data volume,domain adaptation naive Bayes classifier,feature generation,informative feature,machine learning algorithm,next generation sequencing technology cost,nonmodel organism,protein localization prediction,unsupervised annotation
AI 理解论文
溯源树
样例
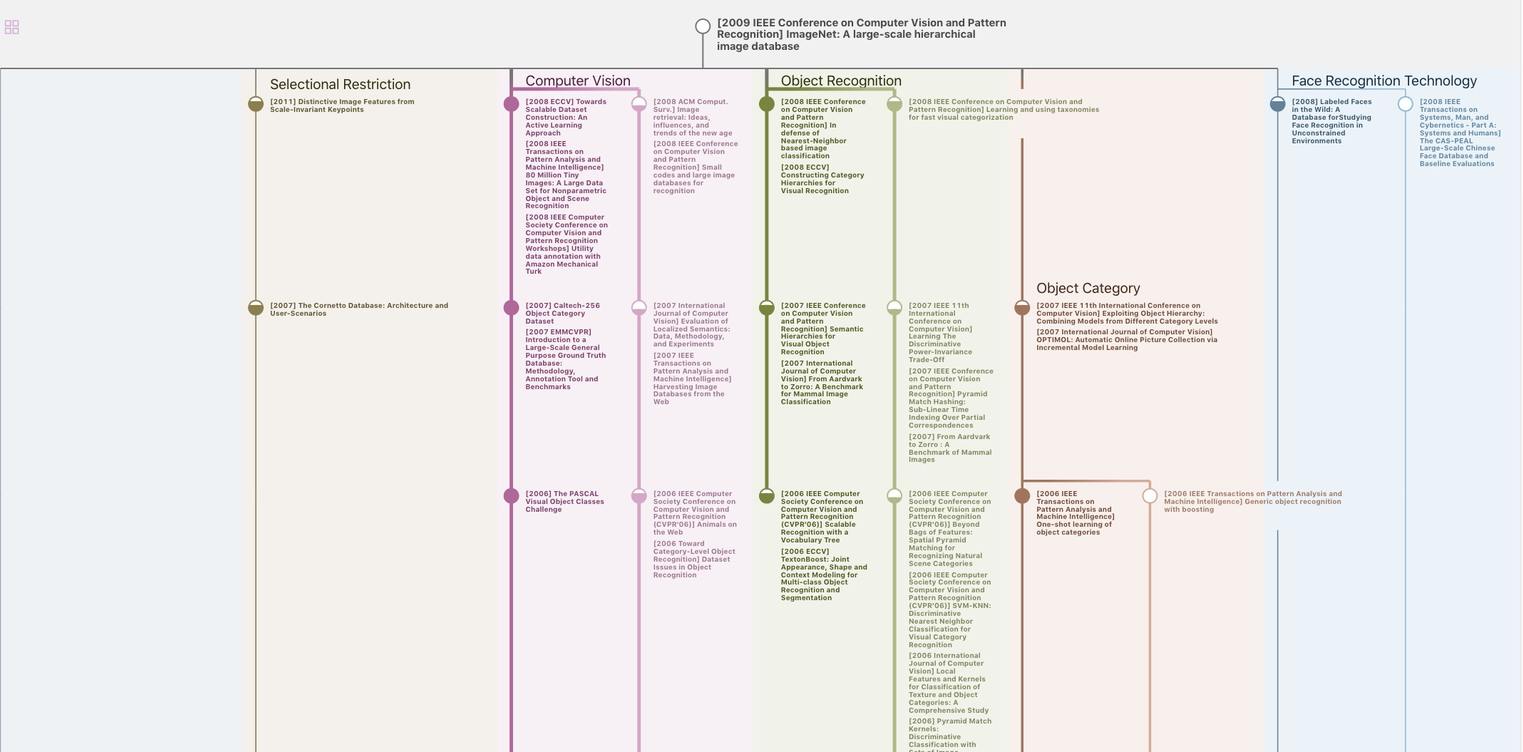
生成溯源树,研究论文发展脉络
Chat Paper
正在生成论文摘要