Towards real-time traffic sign detection and classification
IEEE Trans. Intelligent Transportation Systems(2016)
摘要
This paper aims to deal with real-time traffic sign recognition, i.e. localizing what type of traffic sign appears in which area of an input image at a fast processing time. To achieve this goal, a two-module framework (detection module and classification module) is proposed. In detection module, we firstly transform the input color image to probability maps by using color probability model. Then the traffic sign proposals are extracted by finding maximally stable extremal regions on these maps. Finally, an SVM classifier which trained with color HOG features is utilized to further filter out the false positives and classify the remaining proposals to their super classes. In classification module, we use CNN to classify the detected signs to their sub-classes within each super class. Experiments on the GTSDB benchmark show that our method achieves comparable performance to the state-of-the-art methods with significantly improved computational efficiency, which is 20 times faster than the existing best method.
更多查看译文
关键词
image classification,object detection,object recognition,traffic information systems,svm classifier,color hog features,color probability model,probability maps,real-time traffic sign classification,real-time traffic sign detection,real-time traffic sign recognition,classification,histograms,image analysis,cognition,real time information,support vector machines
AI 理解论文
溯源树
样例
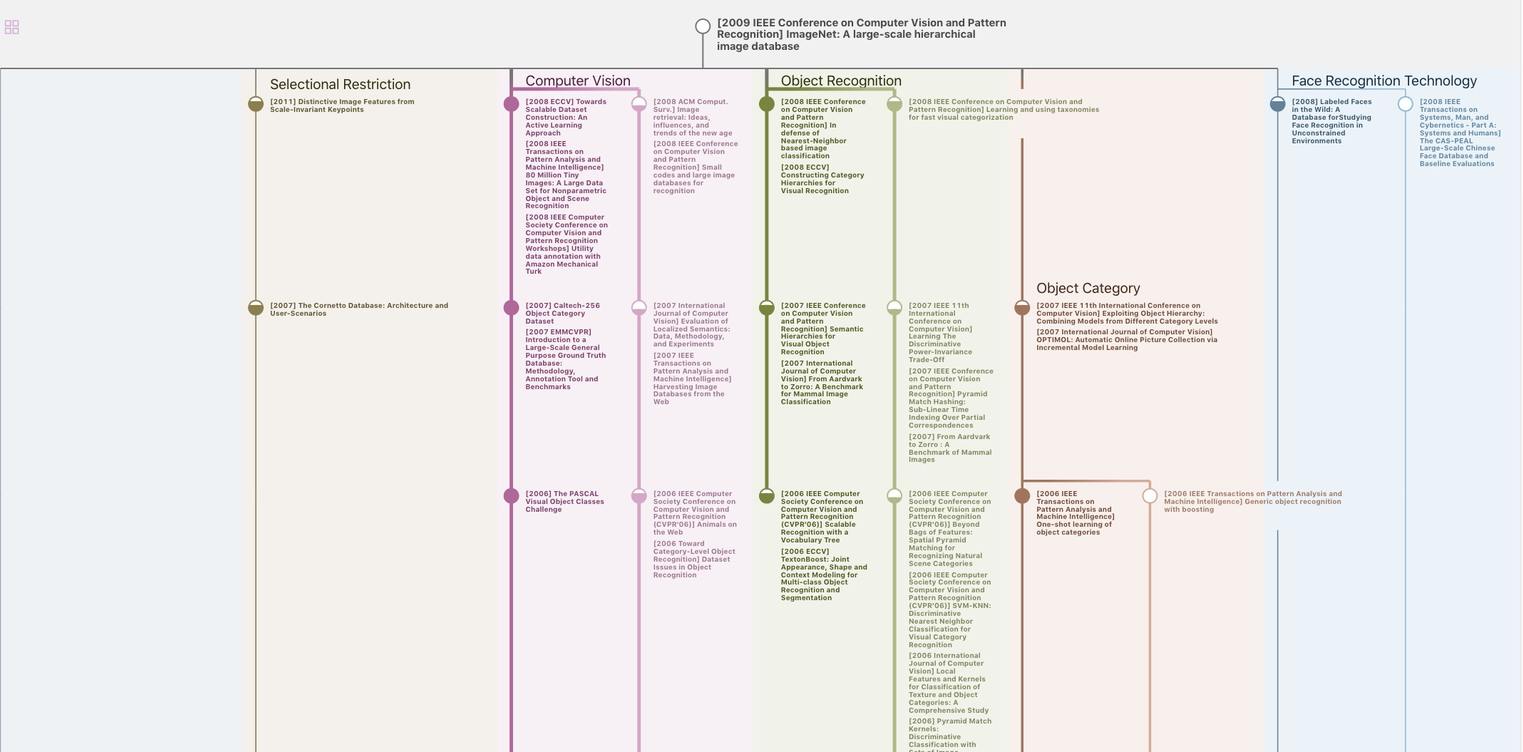
生成溯源树,研究论文发展脉络
Chat Paper
正在生成论文摘要